Predicting dynamic compressive strength of frozen-thawed rocks by characteristic impedance and data-driven methods
Journal of Rock Mechanics and Geotechnical Engineering(2023)
摘要
In cold regions, the dynamic compressive strength (DCS) of rock damaged by freeze-thaw weathering significantly influences the stability of rock engineering. Nevertheless, testing the dynamic strength under freeze-thaw weathering conditions is often both time-consuming and expensive. Therefore, this study considers the effect of characteristic impedance on DCS and aims to quickly determine the DCS of frozen-thawed rocks through the application of machine-learning techniques. Initially, a database of DCS for frozen-thawed rocks, comprising 216 rock specimens, was compiled. Three external load parameters (freeze-thaw cycle number, confining pressure, and impact pressure) and two rock parameters (characteristic impedance and porosity) were selected as input variables, with DCS as the predicted target. This research optimized the kernel scale, penalty factor, and insensitive loss coefficient of the support vector regression (SVR) model using five swarm intelligent optimization algorithms, leading to the development of five hybrid models. In addition, a statistical DCS prediction equation using multiple linear regression techniques was developed. The performance of the prediction models was comprehensively evaluated using two error indexes and two trend indexes. A sensitivity analysis based on the cosine amplitude method has also been conducted. The results demonstrate that the proposed hybrid SVR-based models consistently provided accurate DCS predictions. Among these models, the SVR model optimized with the chameleon swarm algorithm exhibited the best performance, with metrics indicating its effectiveness, including root mean square error (RMSE) = 3.9675, mean absolute error (MAE) = 2.9673, coefficient of determination (R2) = 0.98631, and variance accounted for (VAF) = 98.634. This suggests that the chameleon swarm algorithm yielded the most optimal results for enhancing SVR models. Notably, impact pressure and characteristic impedance emerged as the two most influential parameters in DCS prediction. This research is anticipated to serve as a reliable reference for estimating the DCS of rocks subjected to freeze-thaw weathering.
更多查看译文
关键词
Freeze-thaw cycle,Characteristic impedance,Dynamic compressive strength,Machine learning,Support vector regression
AI 理解论文
溯源树
样例
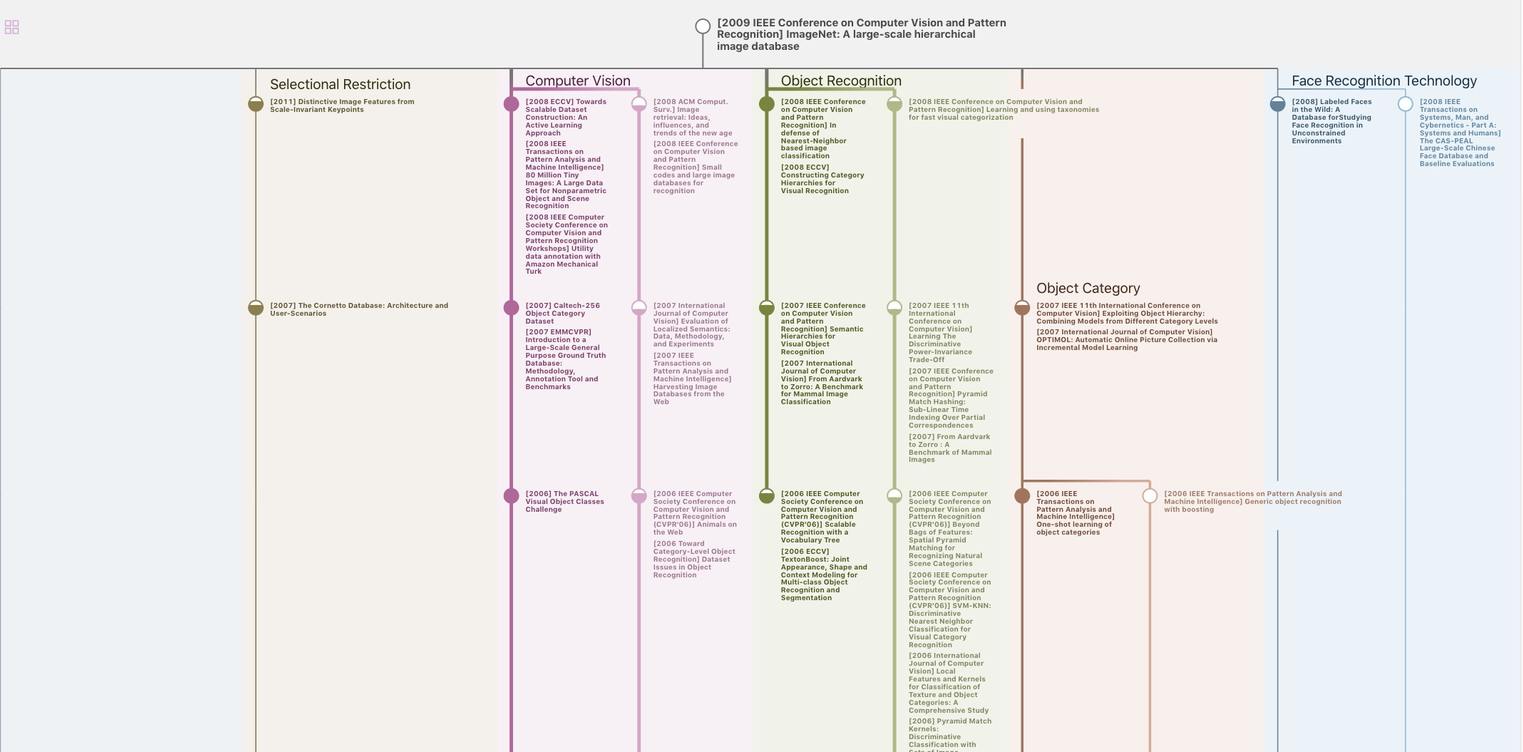
生成溯源树,研究论文发展脉络
Chat Paper
正在生成论文摘要