Improved Foreign Object Tracking Algorithm in Coal for Belt Conveyor Gangue Selection Robot with YOLOv7 and DeepSORT
Measurement(2024)
摘要
Given the indistinct dissimilarities between foreign matter and coal in terms of their physical characteristics, the utilization of machine vision detection technology for foreign matter tracking yields suboptimal accuracy and precision, thereby failing to satisfy the exigencies of coal mine production. In this paper, we proffer an enhanced you only look once version 7 (YOLOv7) and simple online and realtime tracking with a deep association metric (DeepSORT) algorithm for the purpose of tracking foreign entities in the coal domain. The YOLOv7 network model undergoes enhancements through the reduction of Backbone convolutional layers, the introduction of the context overlap and transition network (COTN) module, and the incorporation of a compact target detection layer. Concurrently, the DeepSORT tracking algorithm is refined by substituting the re-recognition network structure of DeepSORT with the machine translation interface (MTL) framework and replacing the DeepSORT foreign object tracking algorithm with the occlusion-aware spatial attention (OSA) module. Empirical findings substantiate the efficacy of the proposed algorithm, as it successfully achieves foreign object detection and tracking. Specifically, the algorithm attains a foreign object detection accuracy of 91.3% and a recall rate of 90.6%. In addition, it achieves a tracking accuracy of 76.1% for multiple object tracking accuracy (MOTA), and a precision rate of 80.6% for multiple object tracking precision (MOTP). Notably, in comparison to the DeepSORT tracking algorithm, the proposed algorithm exhibits a significant improvement of 6 percentage points in MOTA and 3.9 percentage points in MOTP.
更多查看译文
关键词
Deeping learning,Mining,YOLOv7,DeepSORT,Multi-target tracking
AI 理解论文
溯源树
样例
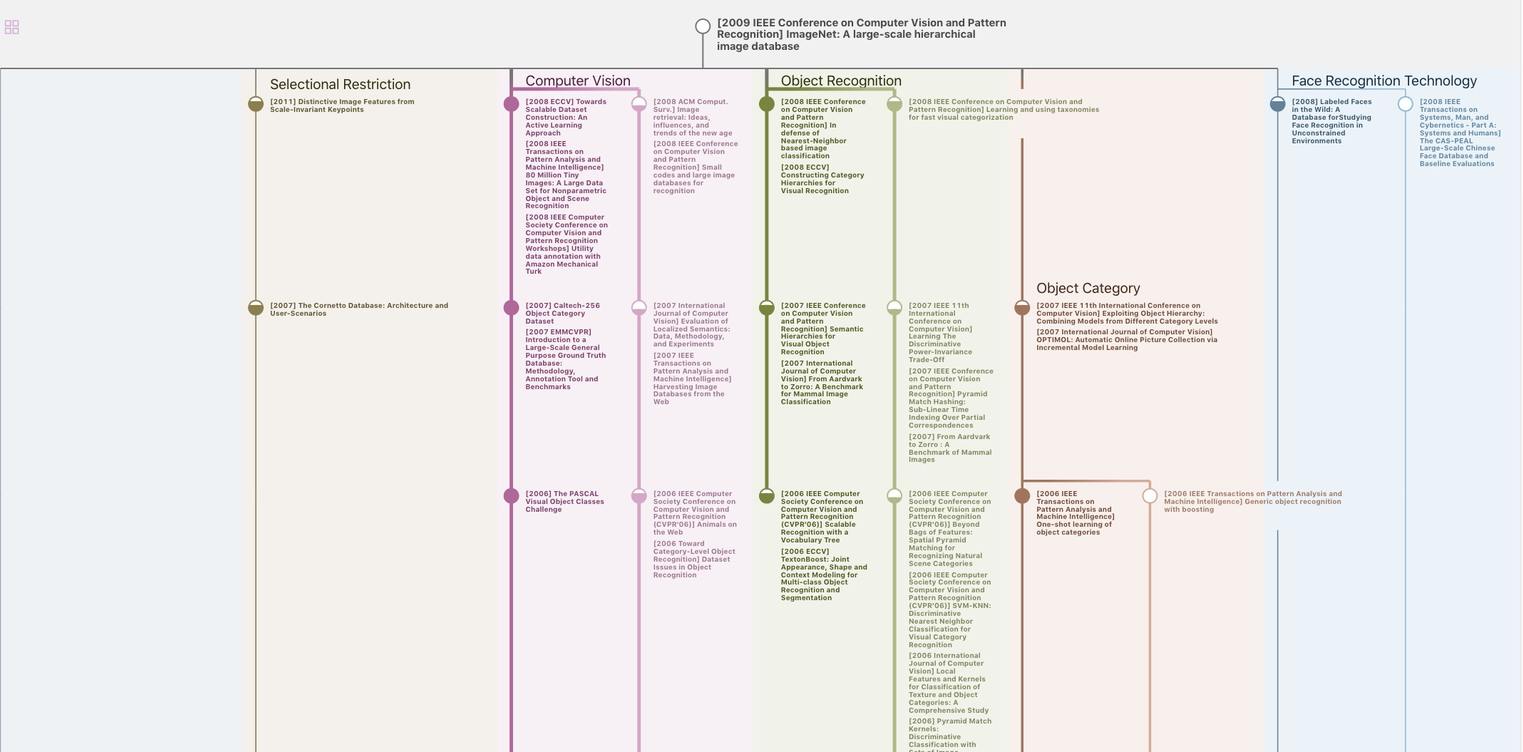
生成溯源树,研究论文发展脉络
Chat Paper
正在生成论文摘要