DctViT: Discrete Cosine Transform meet vision transformers
Neural Networks(2024)
摘要
Vision transformers (ViTs) have become one of the dominant frameworks for vision tasks in recent years because of their ability to efficiently capture long-range dependencies in image recognition tasks using self-attention. In fact, both CNNs and ViTs have advantages and disadvantages in vision tasks, and some studies suggest that the use of both may be an effective way to balance performance and computational cost. In this paper, we propose a new hybrid network based on CNN and transformer, using CNN to extract local features and transformer to capture long-distance dependencies. We also proposed a new feature map resolution reduction based on Discrete Cosine Transform and self-attention, named DCT-Attention Down-sample (DAD). Our DctViT-L achieves 84.8% top-1 accuracy on ImageNet 1K, far outperforming CMT, Next-ViT, SpectFormer and other state-of-the-art models, with lower computational costs. Using DctViT-B as the backbone, RetinaNet can achieve 46.8% mAP on COCO val2017, which improves mAP by 2.5% and 1.1% with less calculation cost compared with CMT-S and SpectFormer as the backbone.
更多查看译文
关键词
Deep learning,Computer vision,Image classification,Vision transformer,Discrete cosine transform
AI 理解论文
溯源树
样例
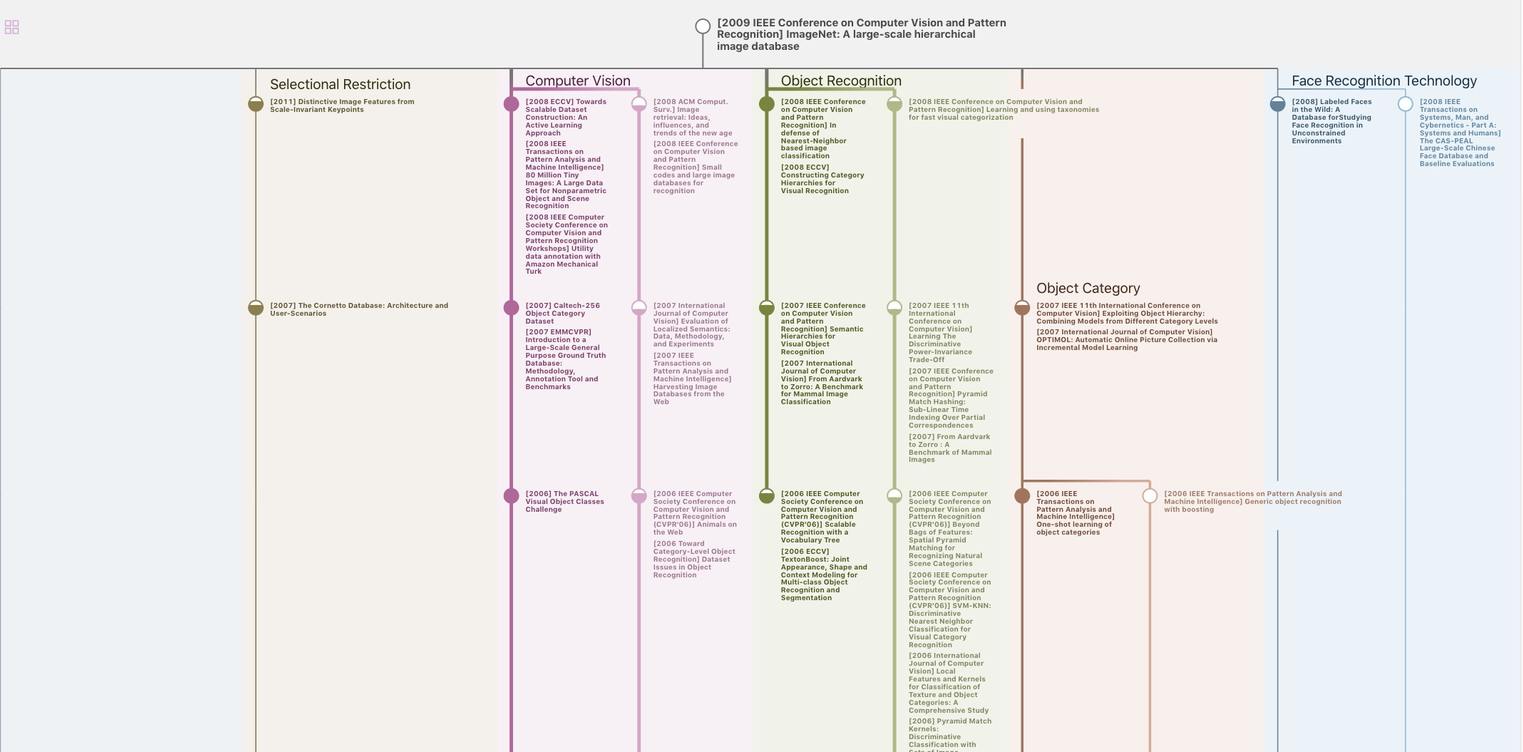
生成溯源树,研究论文发展脉络
Chat Paper
正在生成论文摘要