Tri-relational multi-faceted graph neural networks for automatic question tagging
NEUROCOMPUTING(2024)
摘要
Automatic question tagging is a crucial task in Community Question Answering (CQA) systems such as Zhihu or Quora, as it can significantly enhance the user experience by improving the efficiency of question answering and expert recommendations. Graph -based collaborative filtering models show promising performance on this task, as they can exploit not only the semantics of text content but also the existing relations between questions and tags. However, existing approaches typically encode each question into a single vector, which may not be able to capture the diverse semantic facets of questions in CQA systems. To address this challenge, we propose a novel question -tagging framework, named Tri-Relational Multi -Faceted Graph Neural Networks (TRMFG) for Automatic Question Tagging. In TRMFG, a tri-relational graph structure is designed to better model the question -tag relations. We also propose tri-relational question -tag GNN to extract hidden latent representations of questions and tags. Specially, the Multi -Faceted Question GNN helps capture the diverse semantics of questions from relevant tags. Then we build a multiple matching component to capture more complex matching patterns of the questions based on the diverse semantics. Our experimental results on three benchmark datasets demonstrate that TRMFG significantly improves question tagging performance for CQA, outperforming the state-of-the-art methods.
更多查看译文
关键词
Graph Neural Networks,Community Question Answering,Question Tagging
AI 理解论文
溯源树
样例
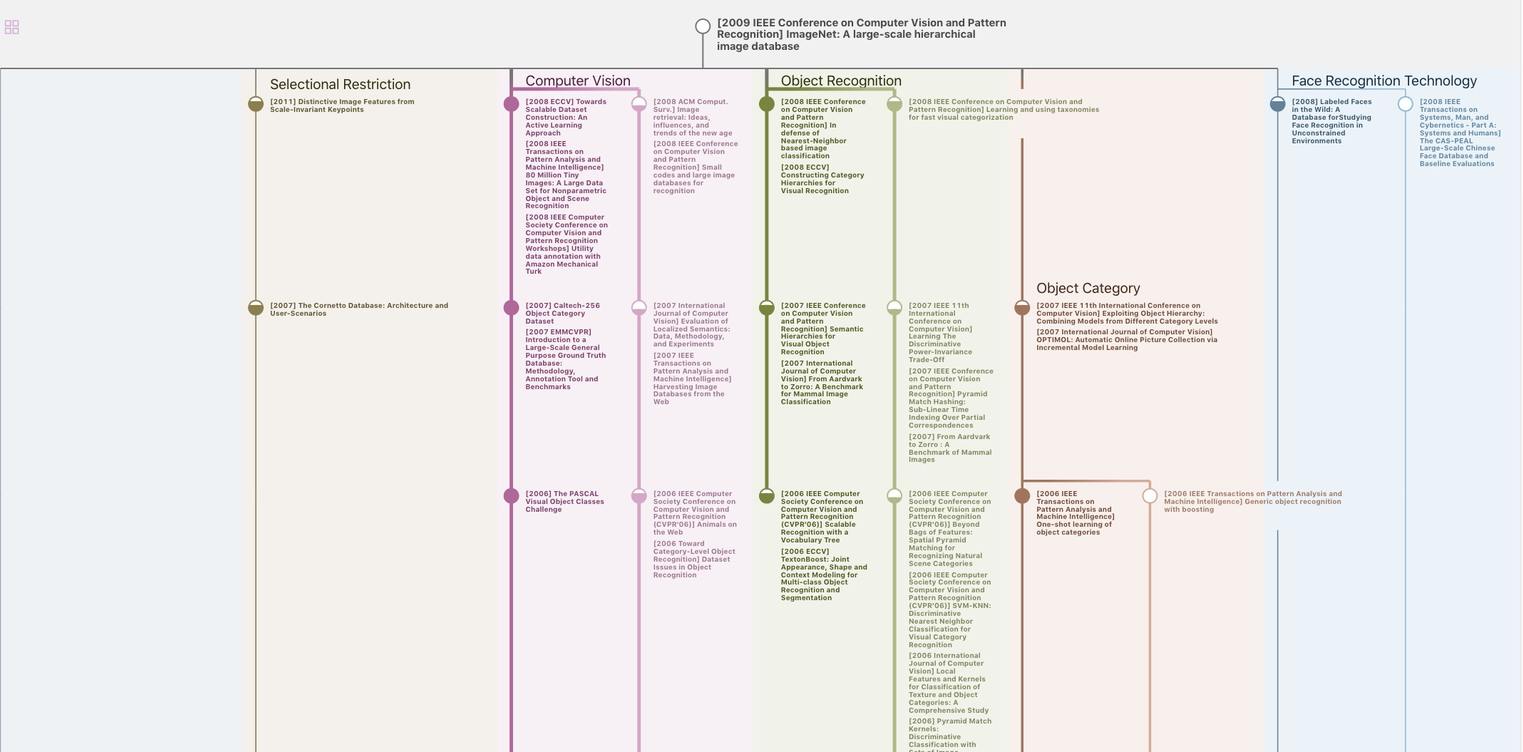
生成溯源树,研究论文发展脉络
Chat Paper
正在生成论文摘要