Gauss-like Logarithmic Kernel Function to improve the performance of kernel machines on the small datasets
Pattern Recognition Letters(2024)
摘要
Support vector machine is one of the most used machine learning algorithms with a comprehensive mathematical infrastructure. The power behind the algorithm is the kernel trick that enables the model to overcome non-linear data distributions by using functions that satisfy the Mercer condition. Undoubtedly, the radial basis function (RBF) is among the most widely used of these functions. The RBF kernel, which has a Gaussian curve, performs local boundary surfaces and supports the generalization capability of the model. In this study, a novel kernel function named Logarithmic Kernel Function (LKF), which has a Gaussian-like curve is presented. The crucial contribution of the LKF is that it can model the dataset better than other similarly shaped kernels in the case of a few training samples (10% and 30%). In the study, 6 classifications and 5 regression sets are handled to compare kernels. Instead of giving the hyperparameters needed by the models manually, they are estimated through the Tree Parzen Estimator, which is based on Sequential Modeling Optimization. This estimation process is repeated 10 times and the average results are obtained. The proposed LKF surpasses the most competitive kernels in various classification and regression tasks.
更多查看译文
关键词
Kernel learning,Support vector machine,Gaussian-like kernel,Small sample size
AI 理解论文
溯源树
样例
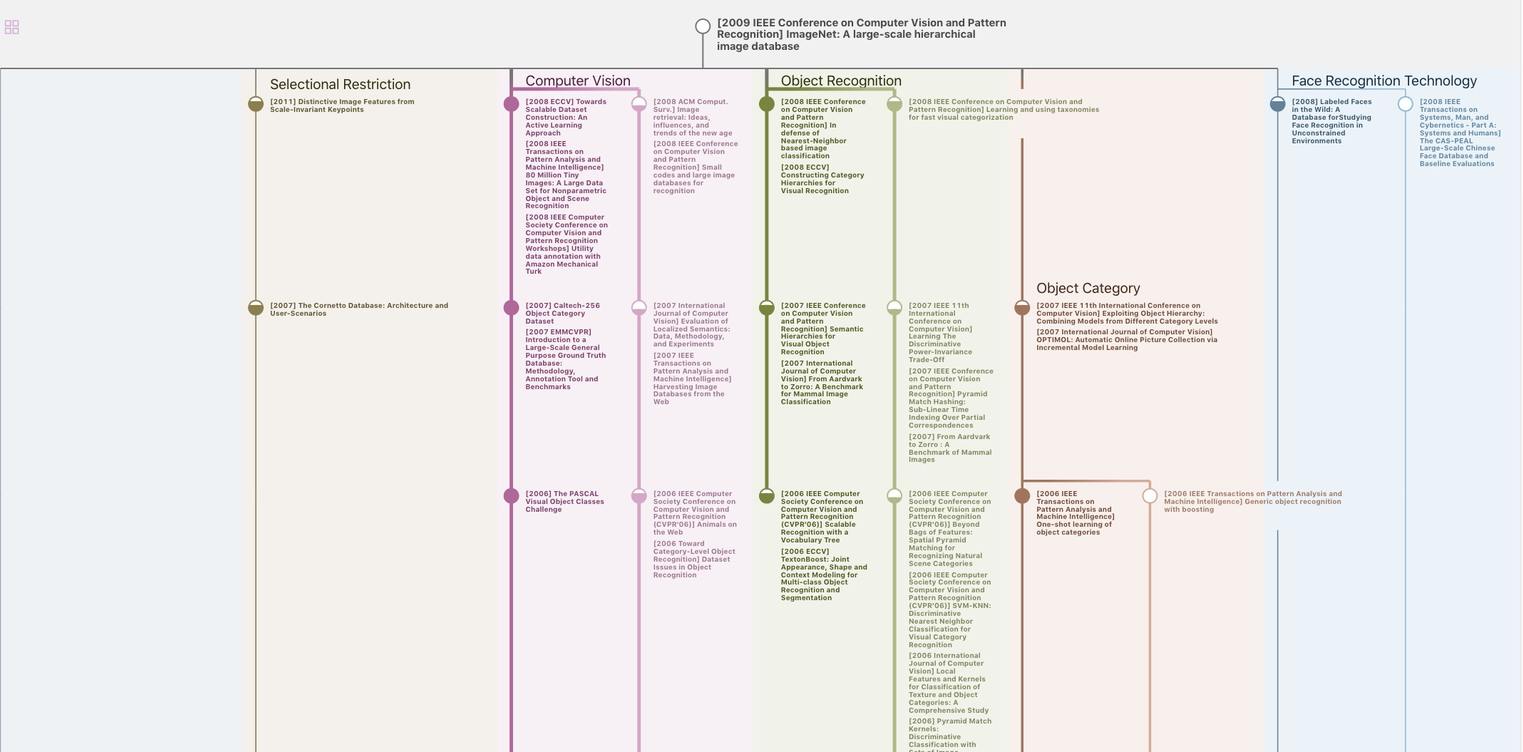
生成溯源树,研究论文发展脉络
Chat Paper
正在生成论文摘要