Evaluation of Integrated Transport Efficiency and Equity at the County Level——taking the Counties in Ningbo City As an Example
TRANSPORT POLICY(2024)
Abstract
The evaluation research on integrated transport has mainly focused on urban agglomeration or cities. Yet the integration of various modes of transportation is increasingly being seen at smaller scales, such as counties, due to population concentration and rising affluence levels. Consequently, it is becoming necessary to evaluate the efficiency and convenience of transportation services for people at each location in the county space. This study has evaluated the efficiency and equity of integrated transport from a county perspective. With respect to efficiency indexes, in addition to transportation infrastructure investment, transportation energy consumption, transportation turnover, and other general indicators, we also take into account the accessibility of people to various types of hubs in the county. We use the BCC (Banker, Charnes, Cooper) model in DEA (Data Envelopment Analysis) for efficiency evaluation. Besides, we evaluate transport equity by using the Gini coefficient to reflect the population distribution of the county and GIS-based spatial accessibility of each hub site. Finally, we establish an evaluation matrix of efficiency and equity; we employ a spatial overlay method to classify research counties into different quadrants. By comprehensively considering the relationship between efficiency and equity in integrated transport, this study can identify areas for improvement in transportation development. The case study evaluation in Ningbo counties, using data from 2017 to 2020, provides practical questions and constructive suggestions for integrated transport development in these areas. Overall, this research has important implications for local transportation development and could inform policy decisions related to integrated transport.
MoreTranslated text
Key words
Integrated transport,Hub accessibility,Comprehensive evaluation,Data envelopment analysis (DEA),Gini coefficient
求助PDF
上传PDF
View via Publisher
AI Read Science
AI Summary
AI Summary is the key point extracted automatically understanding the full text of the paper, including the background, methods, results, conclusions, icons and other key content, so that you can get the outline of the paper at a glance.
Example
Background
Key content
Introduction
Methods
Results
Related work
Fund
Key content
- Pretraining has recently greatly promoted the development of natural language processing (NLP)
- We show that M6 outperforms the baselines in multimodal downstream tasks, and the large M6 with 10 parameters can reach a better performance
- We propose a method called M6 that is able to process information of multiple modalities and perform both single-modal and cross-modal understanding and generation
- The model is scaled to large model with 10 billion parameters with sophisticated deployment, and the 10 -parameter M6-large is the largest pretrained model in Chinese
- Experimental results show that our proposed M6 outperforms the baseline in a number of downstream tasks concerning both single modality and multiple modalities We will continue the pretraining of extremely large models by increasing data to explore the limit of its performance
Upload PDF to Generate Summary
Must-Reading Tree
Example
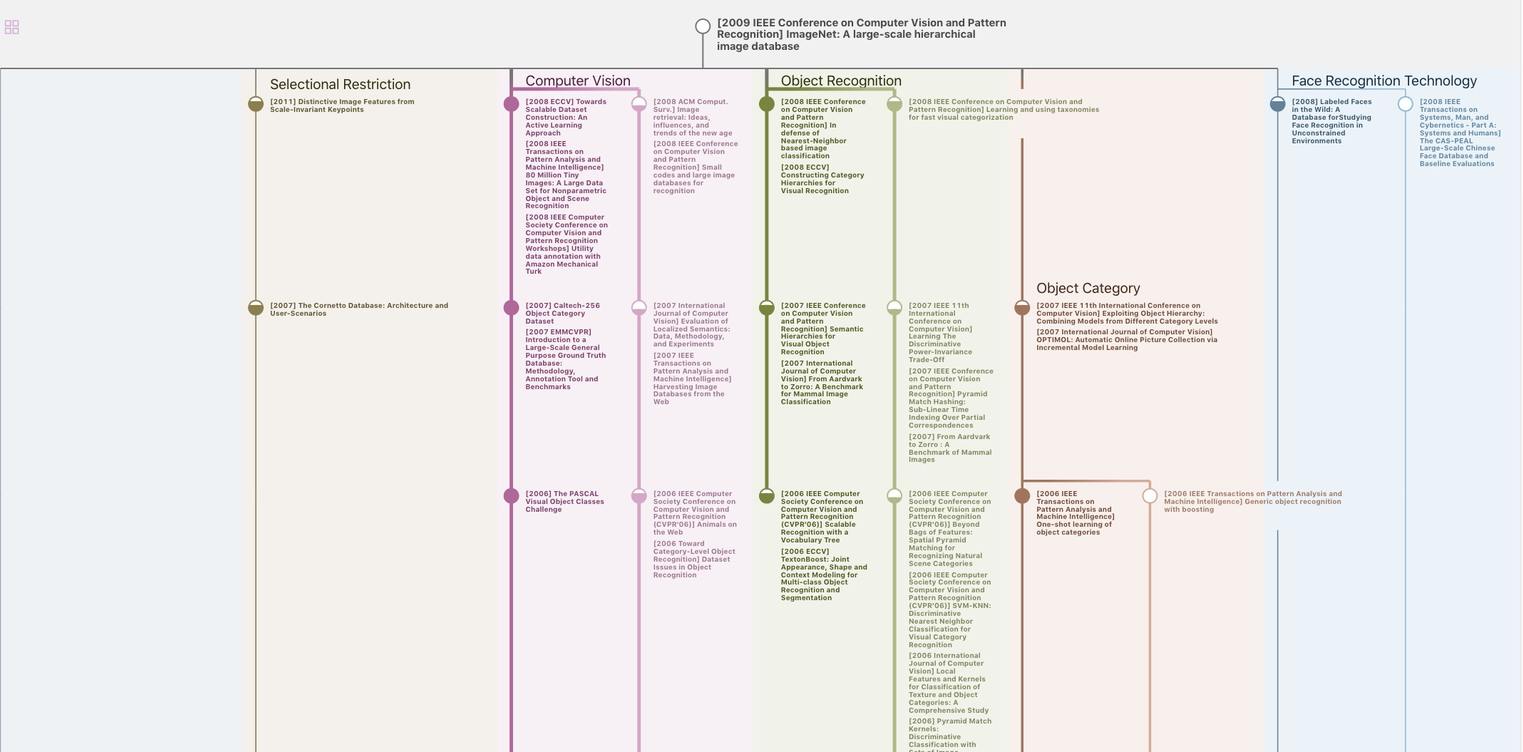
Generate MRT to find the research sequence of this paper
Related Papers
Data Disclaimer
The page data are from open Internet sources, cooperative publishers and automatic analysis results through AI technology. We do not make any commitments and guarantees for the validity, accuracy, correctness, reliability, completeness and timeliness of the page data. If you have any questions, please contact us by email: report@aminer.cn
Chat Paper