Transformer Fault Diagnosis Method Based on SCA-VMD and Improved GoogLeNet
Applied Sciences(2024)
摘要
Aiming at the influence of the fundamental frequency and its harmonics in transformer vibration signals on fault signals, which cause a low fault identification rate and degradation of classification model performance, a new strategy is proposed for fault diagnosis using periodic map spectrum feature maps as input features. In this study, the optimal decomposition parameters were first found adaptively using the VMD improved by the positive cosine optimisation algorithm; then, the transformer vibration signal was modally decomposed, and the periodic map spectrum features were plotted according to the differences in the energy distribution of the different modal components at different frequencies. Finally, the GoogLeNet classification model with the improved attention mechanism assigned different weights to the feature maps in both spatial and channel dimensions to improve the classification accuracy and achieve transformer fault diagnosis. The experimental results verified the validity of the adopted feature map and the proposed model, and the accuracy was significantly improved to 99.04% compared with the traditional GoogLeNet, which is valuable for engineering applications.
更多查看译文
关键词
fault diagnosis,sine-cosine optimisation,adaptive modal decomposition,periodic map spectrum,improving GoogLeNet
AI 理解论文
溯源树
样例
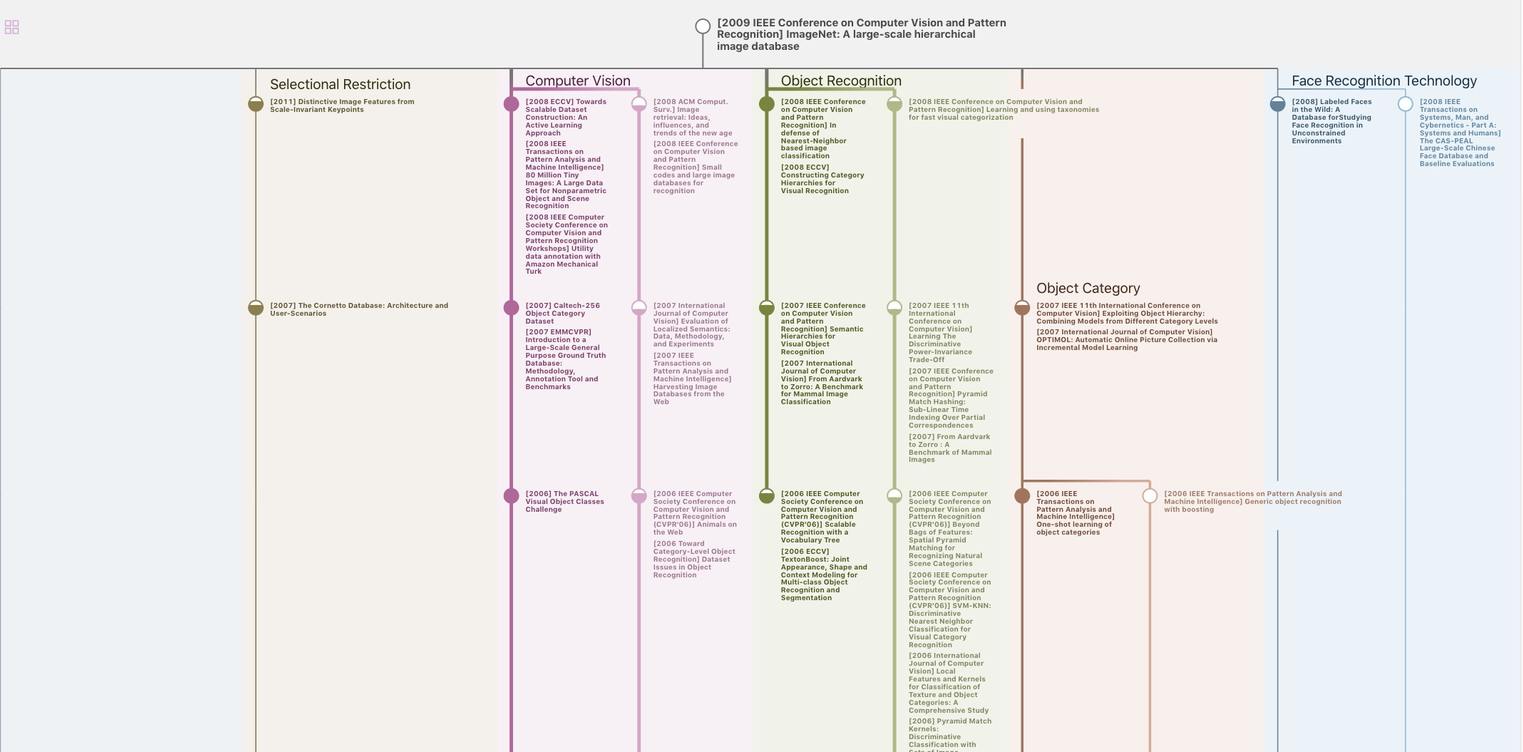
生成溯源树,研究论文发展脉络
Chat Paper
正在生成论文摘要