Concept Drift Detection in Data Stream: Ensemble Learning Method for Detecting Gradual Instances
2023 Asia Meeting on Environment and Electrical Engineering (EEE-AM)(2023)
摘要
Data stream mining faces a significant challenge known as concept drift, especially gradual drift, which can significantly reduce the accuracy of online learning models. To overcome this challenge, it is necessary to detect gradual concept drift precisely and sensitively to make the models adaptive to changes in data distribution. Adapting the model to changes in data distribution before generating new predictions is essential. This article introduces a new method named ERICS+3, an improved version of ERICS, which combines hyperparameters tuning and ensemble learning techniques to create a model for concept drift detection on each data instance of a stream. The experimental results from stream datasets demonstrate that our method is more efficient in detecting gradual concept drift than the original model.
更多查看译文
关键词
concept drift,gradual drift,detection,data stream,ensemble learning,bayesian optimization,voting mechanism
AI 理解论文
溯源树
样例
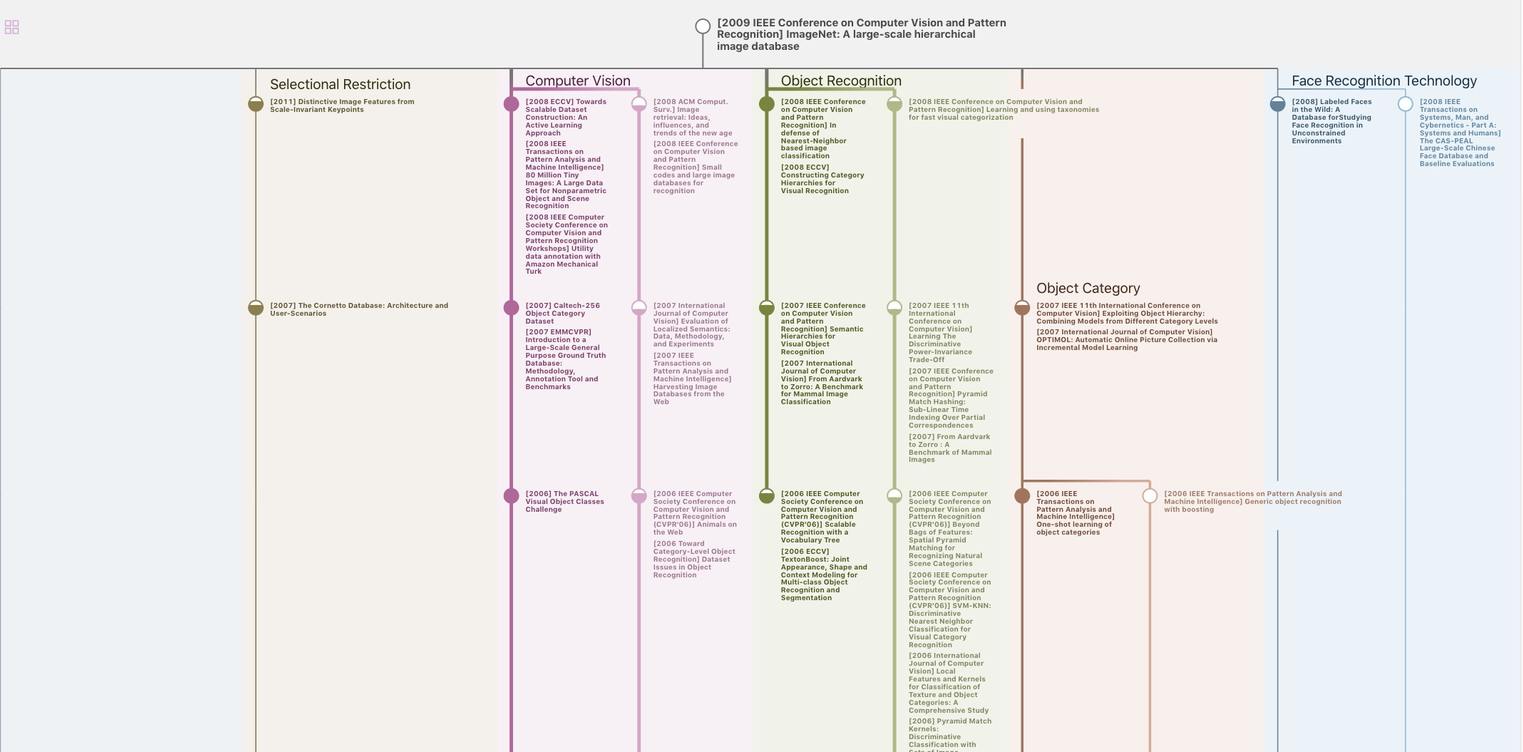
生成溯源树,研究论文发展脉络
Chat Paper
正在生成论文摘要