Data-Driven Control Based on Information Concentration Estimator and Regularized Online Sequential Extreme Learning Machine
SYMMETRY-BASEL(2024)
摘要
Due to the complexity of digital equipment and systems, it is quite difficult to obtain a precise mechanism model in practice. For an unknown discrete-time nonlinear system, in this paper, a semi-parametric model is used to describe this discrete-time nonlinear system, and this semi-parametric model contains a parametric uncertainty part and a nonparametric uncertainty part. Based on this semi-parametric model, a novel data-driven control algorithm based on an information concentration estimator and regularized online sequential extreme learning machine (ReOS-ELM) is designed. The information concentration estimator estimates the parametric uncertainty part; The training data of ReOS-ELM network is obtained, based on symmetry and information concentration estimator, then the training of ReOS-ELM network and the estimate of nonparametric uncertainty part using ReOS-ELM network are carried out online, successively. A stability analysis and three simulation examples were performed, and the simulation results show that the proposed data-driven control algorithm is effective in improving the control accuracy.
更多查看译文
关键词
data-driven control,information concentration estimator,extreme learning machine,unknown discrete-time systems
AI 理解论文
溯源树
样例
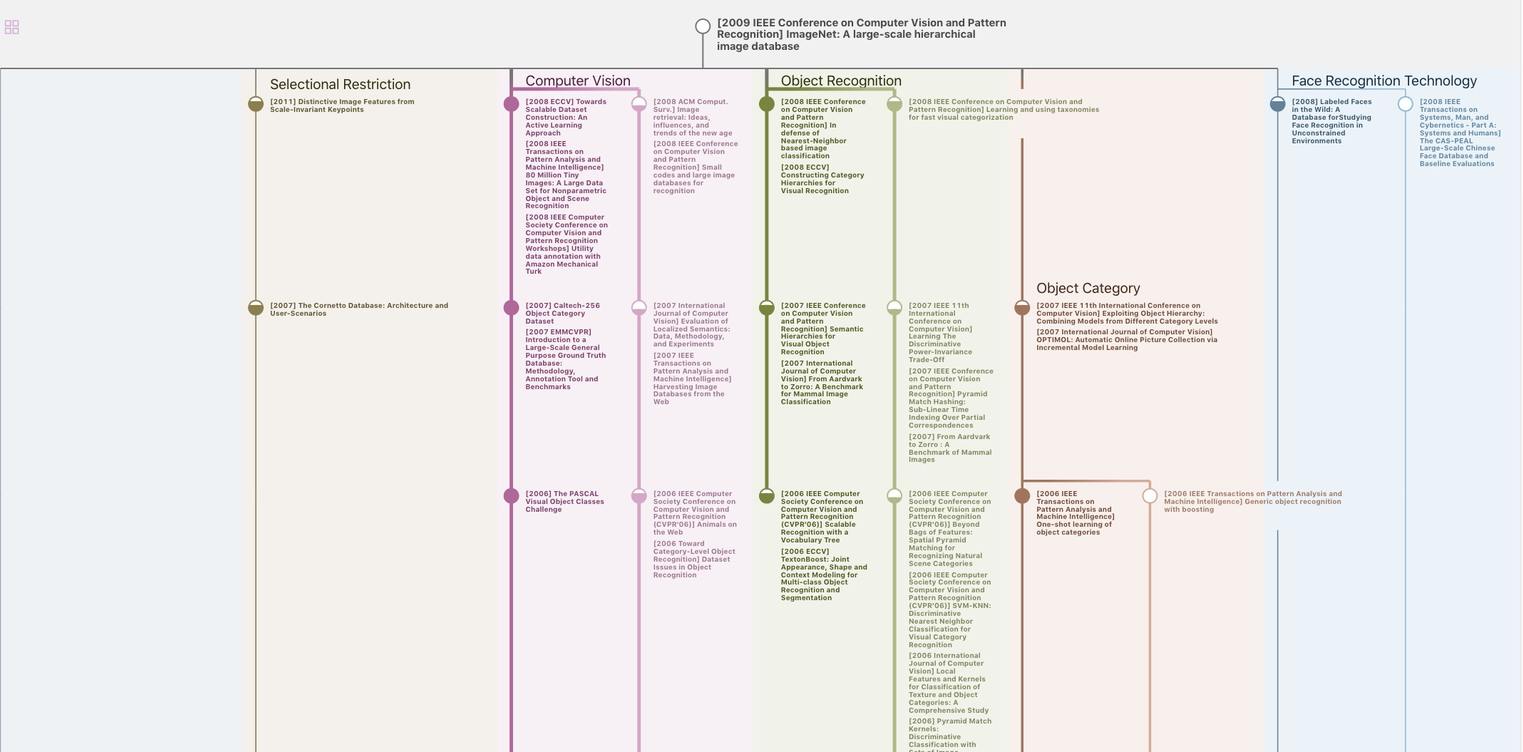
生成溯源树,研究论文发展脉络
Chat Paper
正在生成论文摘要