PolSAR Image Classification Based on Multi-Modal Contrastive Fully Convolutional Network
REMOTE SENSING(2024)
摘要
Deep neural networks have achieved remarkable results in the field of polarimetric synthetic aperture radar (PolSAR) image classification. However, PolSAR is affected by speckle imaging, resulting in PolSAR images usually containing a large amount of speckle noise, which usually leads to the poor spatial consistency of classification results and insufficient classification accuracy. Semantic segmentation methods based on deep learning can realize the task of image segmentation and classification at the same time, producing fine-grained and smooth classification maps. However, these approaches require enormous labeled data sets, which are laborious and time-consuming. Due to these issues, a new multi-modal contrastive fully convolutional network, named MCFCN, is proposed for PolSAR image classification in this paper, which combines multi-modal features of the same pixel as inputs to the model based on a fully convolutional network and accomplishes the classification task using only a small amount of labeled data through contrastive learning. In addition, to describe the PolSAR terrain targets more comprehensively and enhance the robustness of the classifier, a pixel overlapping classification strategy is proposed, which can not only improve the classification accuracy effectively but also enhance the stability of the method. The experiments demonstrate that compared with existing classification methods, the classification results of the proposed method for three real PolSAR datasets have higher classification accuracy.
更多查看译文
关键词
contrastive learning,fully convolutional network (FCN),polarimetric synthetic aperture radar (PolSAR),image classification
AI 理解论文
溯源树
样例
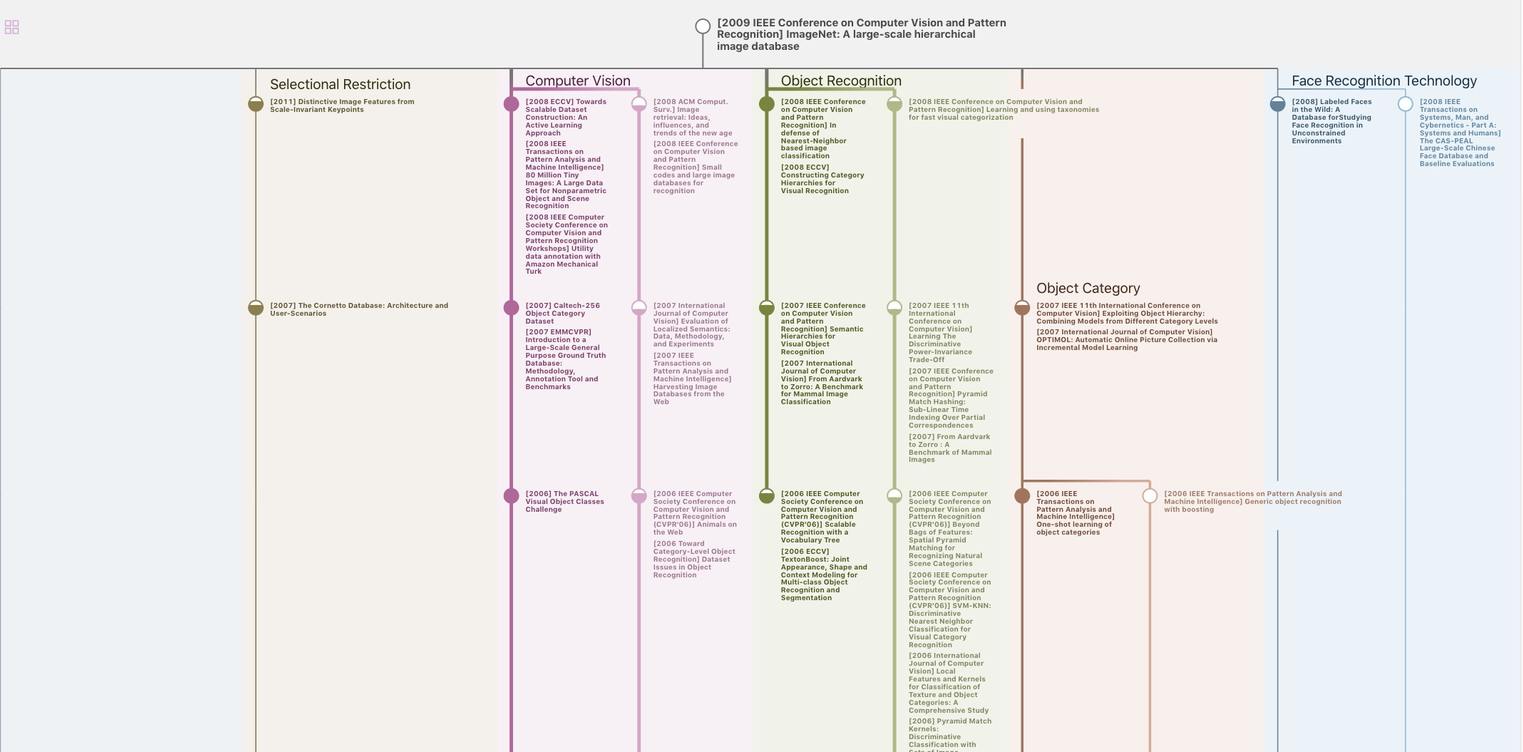
生成溯源树,研究论文发展脉络
Chat Paper
正在生成论文摘要