Lightweight-VGG: A Fast Deep Learning Architecture Based on Dimensionality Reduction and Nonlinear Enhancement for Hyperspectral Image Classification
REMOTE SENSING(2024)
摘要
In the past decade, deep learning methods have proven to be highly effective in the classification of hyperspectral images (HSI), consistently outperforming traditional approaches. However, the large number of spectral bands in HSI data can lead to interference during the learning process. To address this issue, dimensionality reduction techniques can be employed to minimize data redundancy and improve HSI classification performance. Hence, we have developed an efficient lightweight learning framework consisting of two main components. Firstly, we utilized band selection and principal component analysis to reduce the dimensionality of HSI data, thereby reducing redundancy while retaining essential features. Subsequently, the pre-processed data was input into a modified VGG-based learning network for HSI classification. This method incorporates an improved dynamic activation function for the multi-layer perceptron to enhance non-linearity, and reduces the number of nodes in the fully connected layers of the original VGG architecture to improve speed while maintaining accuracy. This modified network structure, referred to as lightweight-VGG (LVGG), was specifically designed for HSI classification. Comprehensive experiments conducted on three publicly available HSI datasets consistently demonstrated that the LVGG method exhibited similar or better performance compared to other typical methods in the field of HSI classification. Our approach not only addresses the challenge of interference in deep learning methods for HSI classification, but also offers a lightweight and efficient solution for achieving high classification accuracy.
更多查看译文
关键词
hyperspectral image classification,dimensionality reduction,multi-layer perceptron,lightweight network
AI 理解论文
溯源树
样例
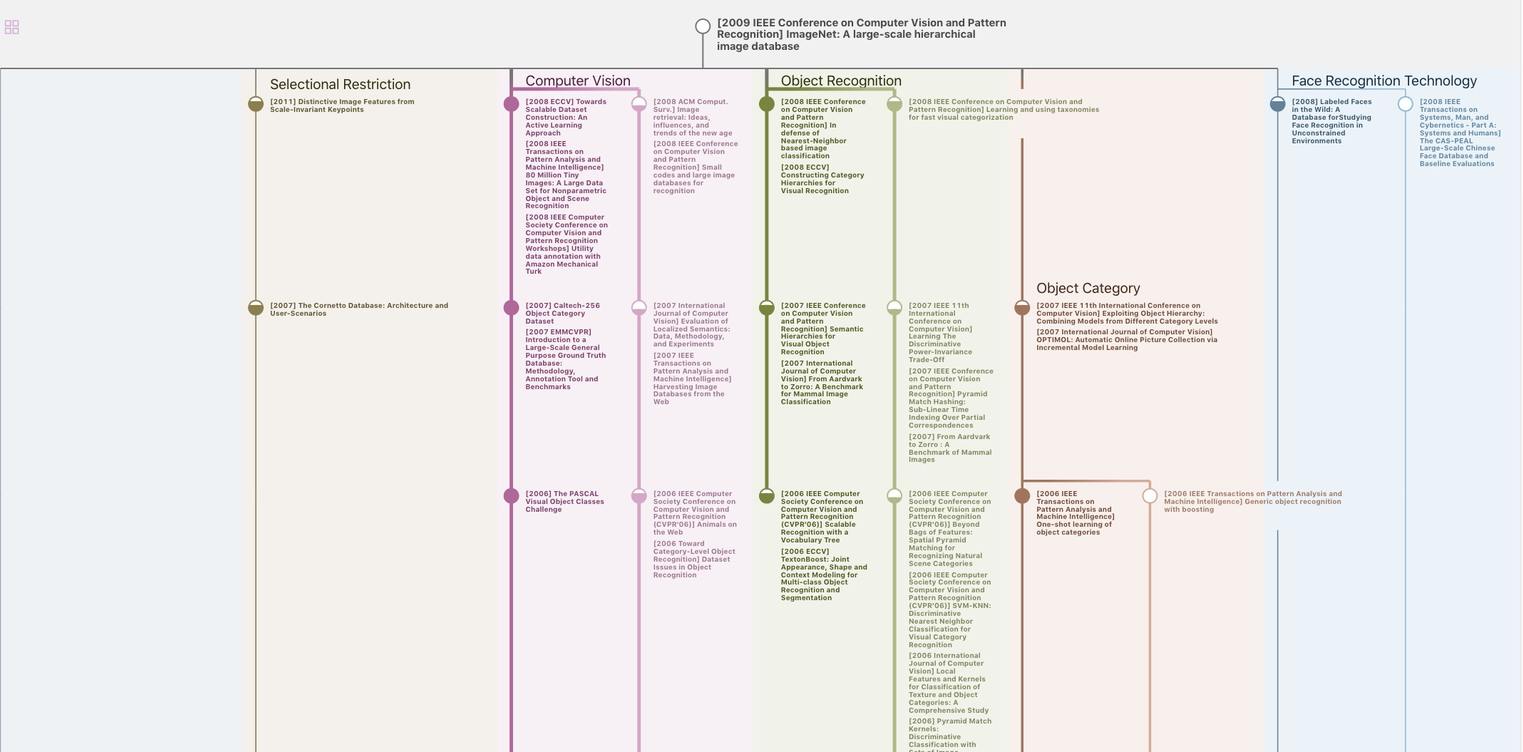
生成溯源树,研究论文发展脉络
Chat Paper
正在生成论文摘要