MFGAN: Multimodal Fusion for Industrial Anomaly Detection Using Attention-Based Autoencoder and Generative Adversarial Network
Sensors(2024)
摘要
Anomaly detection plays a critical role in ensuring safe, smooth, and efficient operation of machinery and equipment in industrial environments. With the wide deployment of multimodal sensors and the rapid development of Internet of Things (IoT), the data generated in modern industrial production has become increasingly diverse and complex. However, traditional methods for anomaly detection based on a single data source cannot fully utilize multimodal data to capture anomalies in industrial systems. To address this challenge, we propose a new model for anomaly detection in industrial environments using multimodal temporal data. This model integrates an attention-based autoencoder (AAE) and a generative adversarial network (GAN) to capture and fuse rich information from different data sources. Specifically, the AAE captures time-series dependencies and relevant features in each modality, and the GAN introduces adversarial regularization to enhance the model’s ability to reconstruct normal time-series data. We conduct extensive experiments on real industrial data containing both measurements from a distributed control system (DCS) and acoustic signals, and the results demonstrate the performance superiority of the proposed model over the state-of-the-art TimesNet for anomaly detection, with an improvement of 5.6% in F1 score.
更多查看译文
关键词
industrial anomaly detection,multimodal fusion,attention-based autoencoder,generative adversarial network
AI 理解论文
溯源树
样例
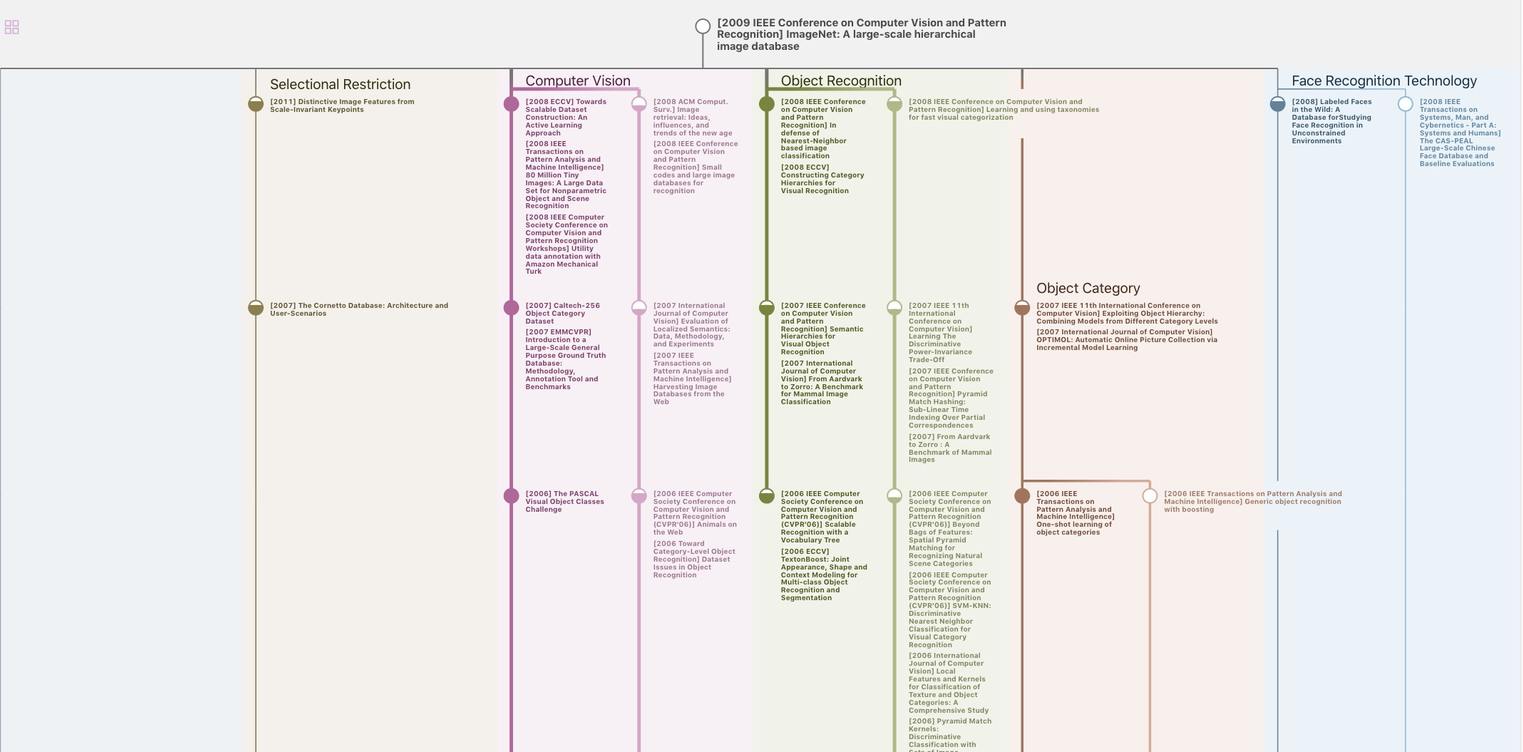
生成溯源树,研究论文发展脉络
Chat Paper
正在生成论文摘要