A Synergistic MOEA Algorithm with GANs for Complex Data Analysis
MATHEMATICS(2024)
摘要
The multi-objective evolutionary algorithm optimization (MOEA) is a challenging but critical approach for tackling complex data analysis problems. However, prevailing MOEAs often rely on single strategies to obtain optimal solutions, leading to concerns such as premature convergence and insufficient population diversity, particularly in high-dimensional data scenarios. In this paper, we propose a novel adversarial population generation algorithm, APG-SMOEA, which synergistically combines the benefits of MOEAs and Generative Adversarial Networks (GANs) to address these limitations. In order to balance the efficiency and quality of offspring selection, we introduce an adaptive population entropy strategy, which includes control parameters based on population entropy and a learning pool for storing and retrieving optimal solutions. Additionally, we attempt to alleviate the training complexity and model collapse problems common in GANs with APG-SMOEA. Experimental results on benchmarks demonstrate that the proposed algorithm is superior to the existing algorithms in terms of solution quality and diversity of low-dimensional or high-dimensional complex data.
更多查看译文
关键词
multi-objective optimization,evolutionary algorithm,generative adversarial network,complex data analysis
AI 理解论文
溯源树
样例
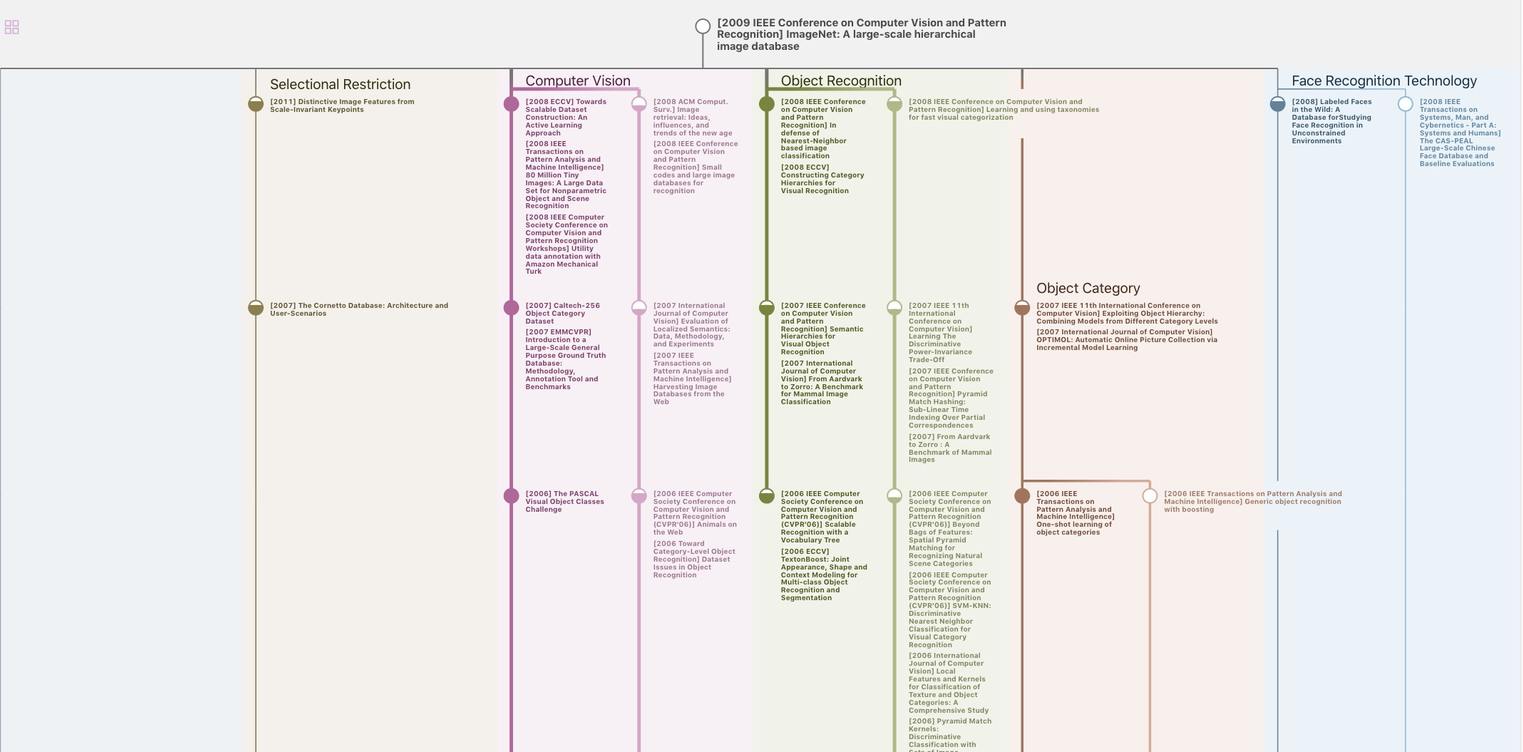
生成溯源树,研究论文发展脉络
Chat Paper
正在生成论文摘要