Diabetic Retinopathy Detection using Squeezenet
2023 2nd International Conference on Automation, Computing and Renewable Systems (ICACRS)(2023)
摘要
Diabetic retinopathy (DR), which causes lesions on the retina and impairs vision, is a common complication of diabetes mellitus. It can cause blindness if it is not caught in time. With early identification and treatment of DR, the risk of vision loss can be considerably decreased. Ophthalmologists must manually diagnose DR retina fundus images, which is more expensive, time-consuming, and error-prone than computer-aided diagnosis technologies. Recently, deep learning has become one of the most well-liked techniques for enhancing performance, especially in classifying and interpreting medical pictures. Deep learning models can detect specific lesions or abnormalities associated with diabetic retinopathy such as microaneurysms, hemorrhages, exudates, and neovascularization. By training CNNs on annotated retinal images with labeled lesions, these models can learn to identify and localize the presence of these abnormalities, aiding in early detection and diagnosis. The images have been converted to grayscale using an image batch processor and the images are resized are the pre-processing steps. Using deep learning algorithms like Squeezenet, diabetic retinopathy images are classified into No DR indications, mild NPDR, moderate NPDR, Severe NPDR, extremely severe NPDR, PDR, and Advanced PDR cases. Squeezenet produced an accuracy of 94.7% without augmentation and had an accuracy of 97 percent. Then the pre-processed images are undergone.
更多查看译文
关键词
Diabetic retinopathy,Retino images,Classification,Squeezenet
AI 理解论文
溯源树
样例
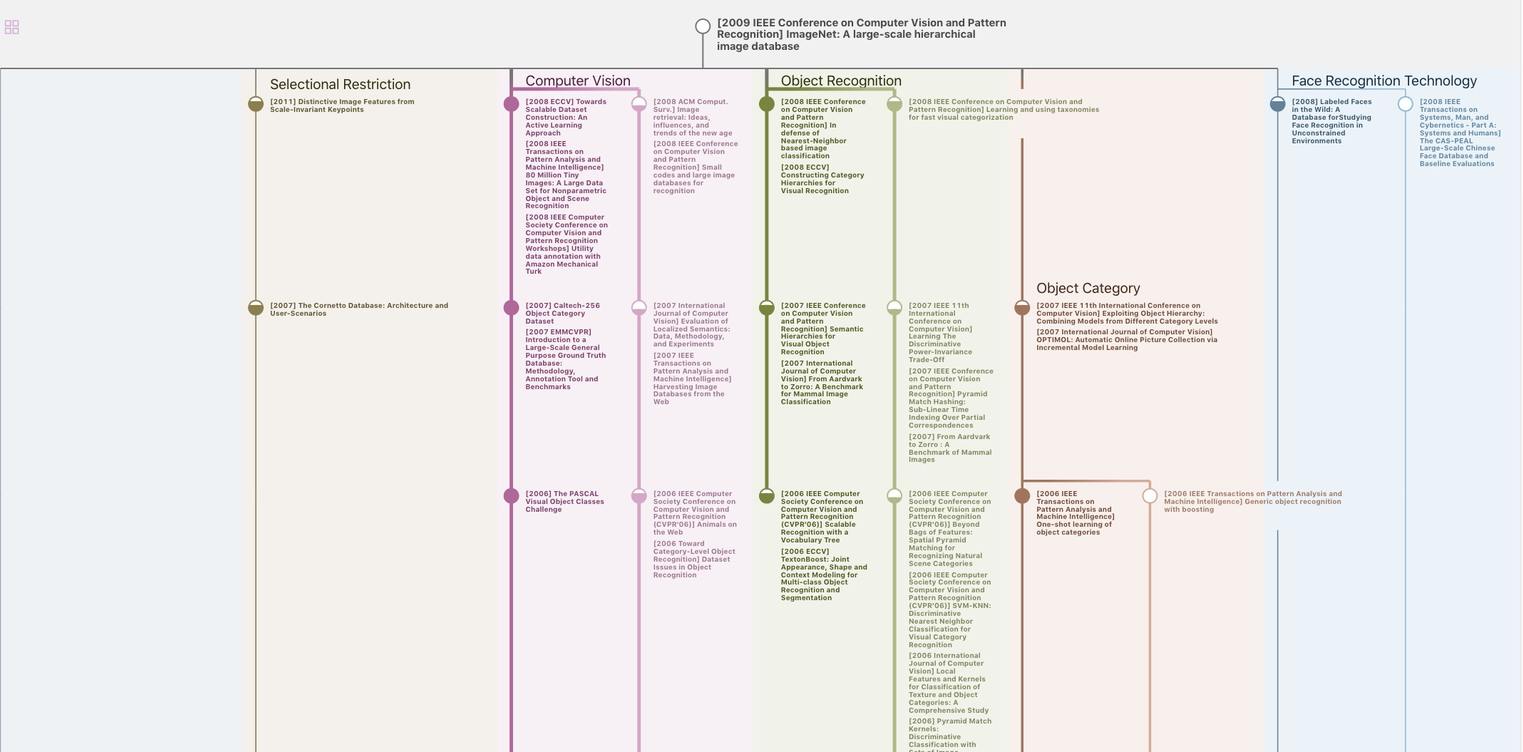
生成溯源树,研究论文发展脉络
Chat Paper
正在生成论文摘要