Evaluation of Deep Learning Model for Detecting Electronic Components in Few-Shot Learning Scenarios.
2023 IEEE Latin American Conference on Computational Intelligence (LA-CCI)(2023)
摘要
The increasing waste of electronic equipment causes adverse environmental impacts and emphasizes the importance of component reuse for a sustainable circular economy. This study uses few-shot learning to explore variations of machine learning models, specifically deep learning, to detect toroidal inductors on circuit boards. A customized database with 50 labeled images of toroidal inductors was developed and made available online due to the limitation of available data. Three detection models were trained and evaluated using different amounts of training images (10, 20, and 30 images) and proportional epochs. The results show that models trained with 20 and 30 images achieved satisfactory performance, with an accuracy of 96.8% and 97.1%, and mAP50 of 98.2% and 99%, respectively. However, the model trained with only ten training images showed inferior results, with an accuracy of 6.2% and recall of 28.6%. The results indicate that using 20 to 30 images in training can be an efficient strategy for detecting components on circuit boards and that the number of training images correlates differently with the model’s performance. Additionally, image quality, proper hyperparameter selection, and choice of detection algorithm are crucial factors in obtaining reliable results in different detection tasks. This study contributes to developing more sustainable and responsible solutions for the reuse of electronic components while emphasizing the importance of considering multiple factors when training detection models to ensure efficient and reliable results.
更多查看译文
关键词
Electronic Component Detection,Component Reuse,Few-shot Learning,Deep Learning
AI 理解论文
溯源树
样例
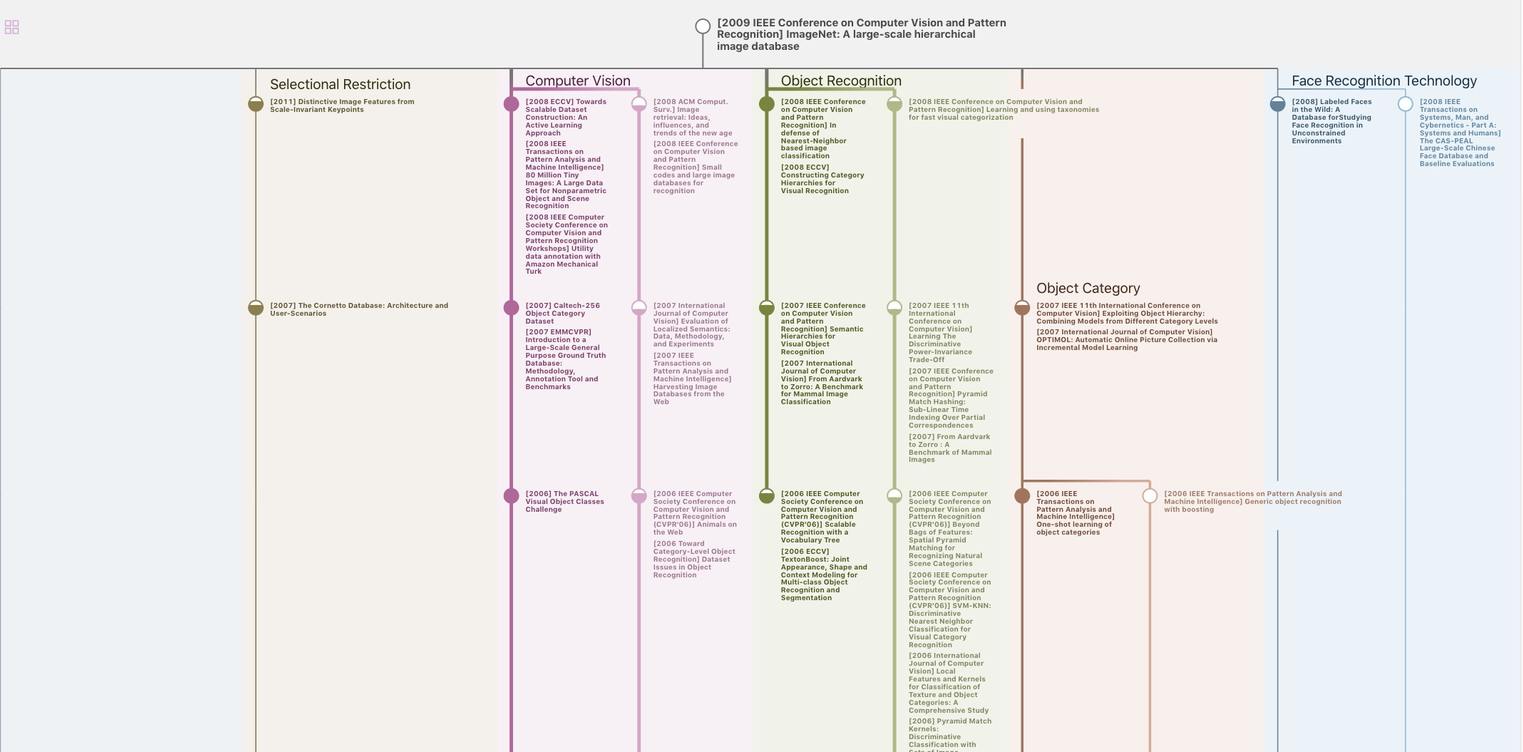
生成溯源树,研究论文发展脉络
Chat Paper
正在生成论文摘要