Representative Feature Learning and Task Decoupling for Few-Shot Object Detection.
2023 7th International Symposium on Computer Science and Intelligent Control (ISCSIC)(2023)
摘要
In few-shot object detection (FSOD), transfer-learning approaches are adopted extensively to transfer knowledge from base classes with numerous annotations to novel classes with finite annotations. However, when training with only a small amount of images, two momentous problems arise: 1. The feature distributions of few-shot samples are easily disturbed by noise and randomness, which may cause biased distributions and lead to serious overfitting. 2. Inconsistent preference of features in classification and regression tasks makes it difficult to optimize the model to match the two tasks simultaneously, resulting in a dramatic performance drop. To address the problems, we propose Representative Feature Learning and Task Decoupling (RFLTD). On the one hand, the representative feature learning module captures representative features and calibrates the distribution by effectively distracting class-marginal and class-irrelevant regions, which reduces model overfitting. On the other hand, the Task Decoupling module separates and emphasizes the ROI-Align feature at the channel level, which improves both the classification and regression performance. RFLTD improves the SOTAs with significant margins on PASCAL VOC and MS COCO datasets, demonstrating the effectiveness of the proposed approach.
更多查看译文
关键词
few-shot object detection,feature distribution,representative feature learning,tasks conflict,decouple enhancement
AI 理解论文
溯源树
样例
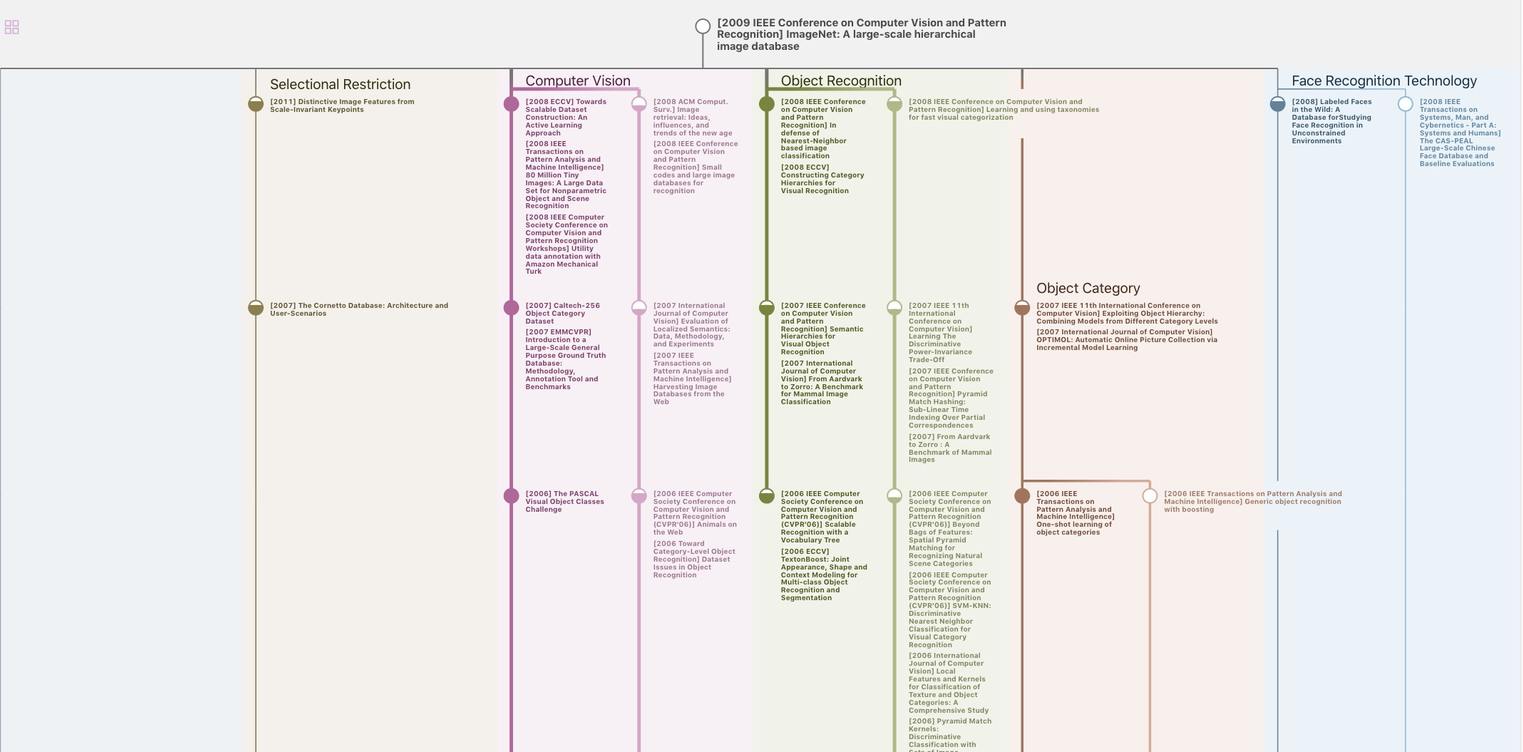
生成溯源树,研究论文发展脉络
Chat Paper
正在生成论文摘要