Multilevel Modelling of Parkinson’s Disease Symptom Progression in 123I FP-CIT SPECT
2022 IEEE Nuclear Science Symposium and Medical Imaging Conference (NSS/MIC)(2022)
摘要
Parkinson’s disease (PD) is the second most prevalent neurodegenerative disorder. It is due to the reduction in the neurotransmitter dopamine by the loss of dopamine neurons. Thanks to new high-precision markers, it is possible to study PD before the development of symptoms and thus to model the progression of the disease. In this study, we apply a non-linear decomposition of 123I FP-CIT SPECT imaging in order to unveil the sources of variability, and relate them to symptomatology and disease progression. Isomap was compared to PCA, and multi-level modelling was used to assess the relation of the resulting composite imaging features and different symptomatology biomarkers and neuropsychological scales. The main source of variability was found to be the intensity at the striata, significantly linked to progression scales such as UPDRS or SCOPA. On the other hand, the modelling found additional sources of variance related to rigidity, tremor or other relevant symptoms.
更多查看译文
关键词
Hierarchical Regression,Symptoms Of Disease Progression,Composition Characteristics,Main Source Of Variation,Loss Of Dopamine Neurons,Prevalent Neurodegenerative Disorder,Accurate Measurement,Cerebrospinal Fluid,Caudate,Nonlinear Model,Class Analysis,Bayesian Information Criterion,Basal Ganglia,Unsupervised Learning,Linear Mixed Effects,Variance In The Dataset,Largest Variation,Computer-aided Diagnosis,Balanced Accuracy,Nonlinear Techniques,Linear Decomposition,Image Decomposition,Diagnosis Of Parkinson’s Disease
AI 理解论文
溯源树
样例
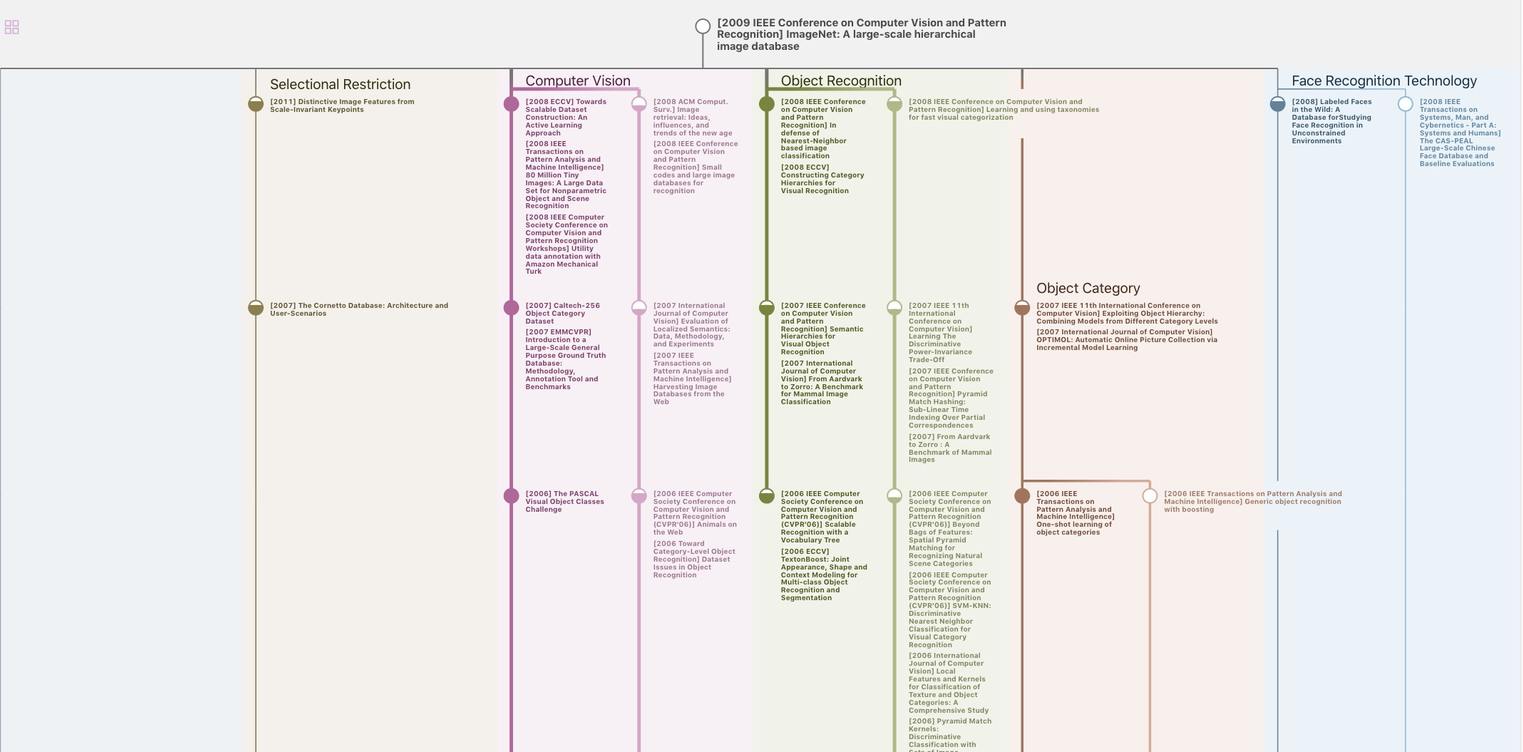
生成溯源树,研究论文发展脉络
Chat Paper
正在生成论文摘要