Ensemble Learning Using Weighted Convolutional Neural Networks for Parkinson’s Disease
2022 IEEE Nuclear Science Symposium and Medical Imaging Conference (NSS/MIC)(2022)
摘要
In recent years, there has been a growing interest on Computer-Aided-Diagnosis (CAD) systems based on Deep Learning to disentangle how neurodegenerative disorders such as Parkinson’s Disease (PD) behave. Although these models are quite accurate, they are often criticized for their lack of interpretability, high complexity and tendency to overfitting (as a result of the amount of input features –intensity voxels from brain scans– in comparison with the reduced number of input samples). Though there are some proposals that are trying to solve these limitations, such as data augmentation and/or interpretable models, it would be interesting, from the point of view of neuroimaging, to develop a CAD model able to 1) split the problem of analyzing the full brain scans and 2) combine the contribution of smaller Regions of Interest (ROIs). This could help us not only to reduce the complexity of the neural networks focused on the analysis of each ROI, but also as a way to quantify the contribution of each region to CAD decisions. In this context, we have proposed an ensemble learning methodology with penalization of non-reliable input sources focused on the analysis of 7 ROIs (including: accumbens, amygdala, anterior cingulate cortex, cingulate, striatum, substantia nigra and thalamus). This method is able to combine the resulting predictions obtained for each ROI by means of a Weighted Majority Voting decision schema using the scores derived from a two-sample Welch’s test. As shown by our results, this proposal achieves classification rates of 97.5% and is an easily adaptable solution for the analysis of other pathologies.
更多查看译文
关键词
deep learning,ensemble learning,convolutional neural networks,neuroimaging,Parkinson’s Disease,signal processing
AI 理解论文
溯源树
样例
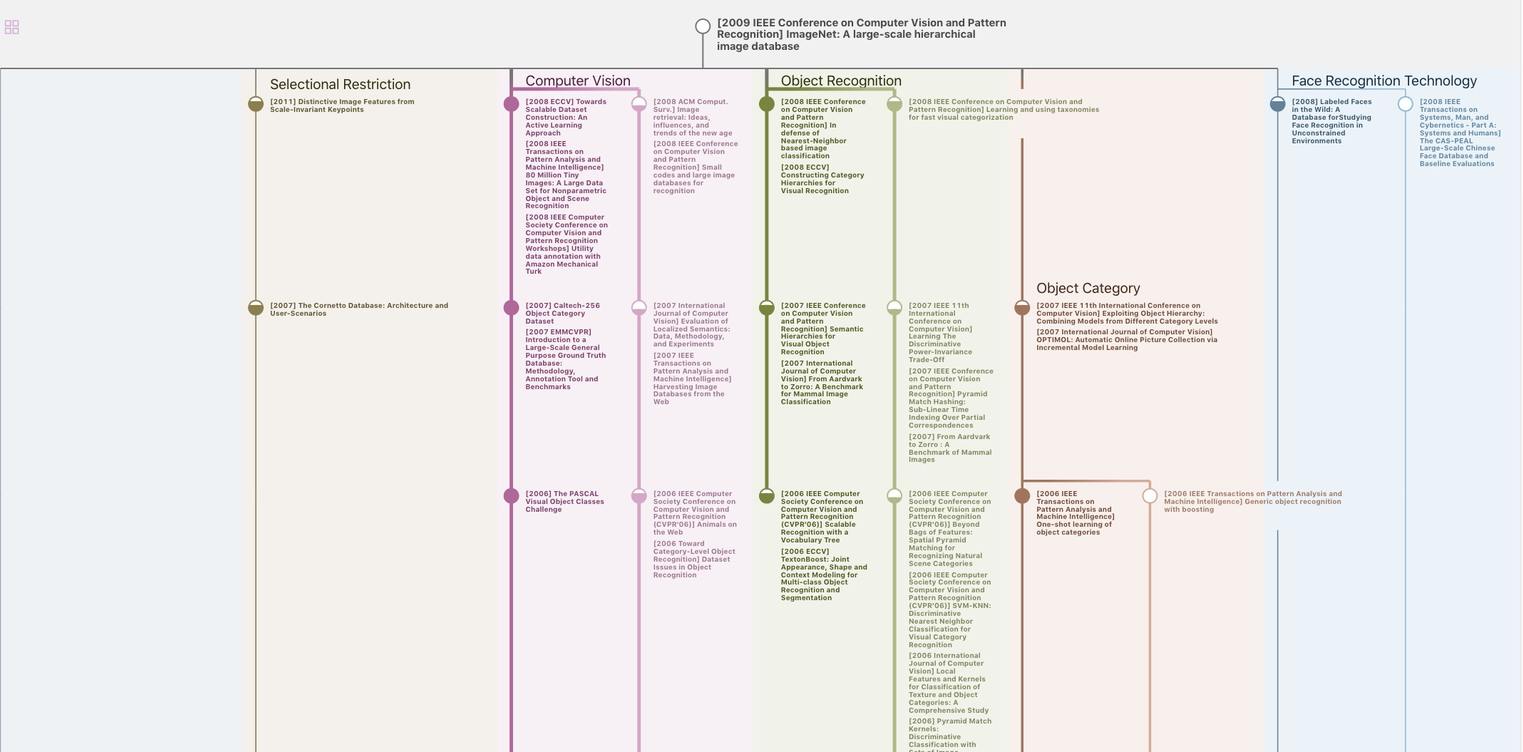
生成溯源树,研究论文发展脉络
Chat Paper
正在生成论文摘要