Security and Safety-Critical Learning-Based Collaborative Control for Multiagent Systems
IEEE TRANSACTIONS ON NEURAL NETWORKS AND LEARNING SYSTEMS(2024)
摘要
This article presents a novel learning-based collaborative control framework to ensure communication security and formation safety of nonlinear multiagent systems (MASs) subject to denial-of-service (DoS) attacks, model uncertainties, and barriers in environments. The framework has a distributed and decoupled design at the cyber-layer and the physical layer. A resilient control Lyapunov function-quadratic programming (RCLF-QP)-based observer is first proposed to achieve secure reference state estimation under DoS attacks at the cyber-layer. Based on deep reinforcement learning (RL) and control barrier function (CBF), a safety-critical formation controller is designed at the physical layer to ensure safe collaborations between uncertain agents in dynamic environments. The framework is applied to autonomous vehicles for area scanning formations with barriers in environments. The comparative experimental results demonstrate that the proposed framework can effectively improve the resilience and robustness of the system.
更多查看译文
关键词
Security,Collaboration,Safety,Denial-of-service attack,Vehicle dynamics,Uncertainty,Task analysis,Denial-of-service (DoS) attacks,learning-based control,multiagent systems (MASs),safety-critical formation control
AI 理解论文
溯源树
样例
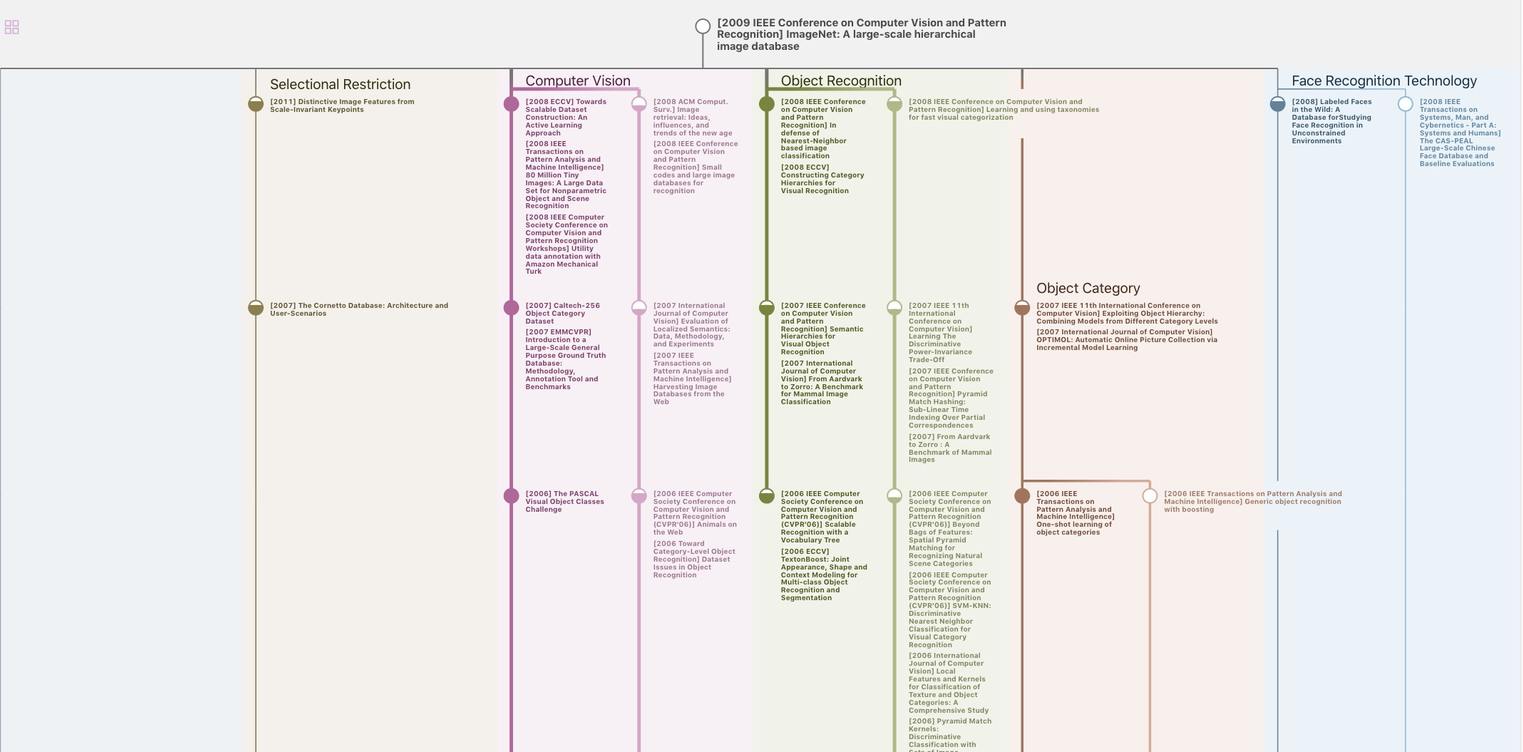
生成溯源树,研究论文发展脉络
Chat Paper
正在生成论文摘要