Radiomic profiling for insular diffuse glioma stratification with distinct biologic pathway activities
CANCER SCIENCE(2024)
摘要
Current literature emphasizes surgical complexities and customized resection for managing insular gliomas; however, radiogenomic investigations into prognostic radiomic traits remain limited. We aimed to develop and validate a radiomic model using multiparametric magnetic resonance imaging (MRI) for prognostic prediction and to reveal the underlying biological mechanisms. Radiomic features from preoperative MRI were utilized to develop and validate a radiomic risk signature (RRS) for insular gliomas, validated through paired MRI and RNA-seq data (N = 39), to identify core pathways underlying the RRS and individual prognostic radiomic features. An 18-feature-based RRS was established for overall survival (OS) prediction. Gene set enrichment analysis (GSEA) and weighted gene coexpression network analysis (WGCNA) were used to identify intersectional pathways. In total, 364 patients with insular gliomas (training set, N = 295; validation set, N = 69) were enrolled. RRS was significantly associated with insular glioma OS (log-rank p = 0.00058; HR = 3.595, 95% CI:1.636-7.898) in the validation set. The radiomic-pathological-clinical model (R-P-CM) displayed enhanced reliability and accuracy in prognostic prediction. The radiogenomic analysis revealed 322 intersectional pathways through GSEA and WGCNA fusion; 13 prognostic radiomic features were significantly correlated with these intersectional pathways. The RRS demonstrated independent predictive value for insular glioma prognosis compared with established clinical and pathological profiles. The biological basis for prognostic radiomic indicators includes immune, proliferative, migratory, metabolic, and cellular biological function-related pathways. We believe that our study makes a significant contribution to the literature because it introduced a prognostic radiomic risk signature (RRS), which allowed the noninvasive stratification of insular glioma patients and complemented the molecular diagnosis-based glioma classification. Furthermore, it revealed the factors that influence prognostic radiomic traits, providing insights that offer strategies for targeted therapy and tailored management of insular gliomas.image
更多查看译文
关键词
biological pathway,insular glioma,machine learning,prognosis,radiomic features
AI 理解论文
溯源树
样例
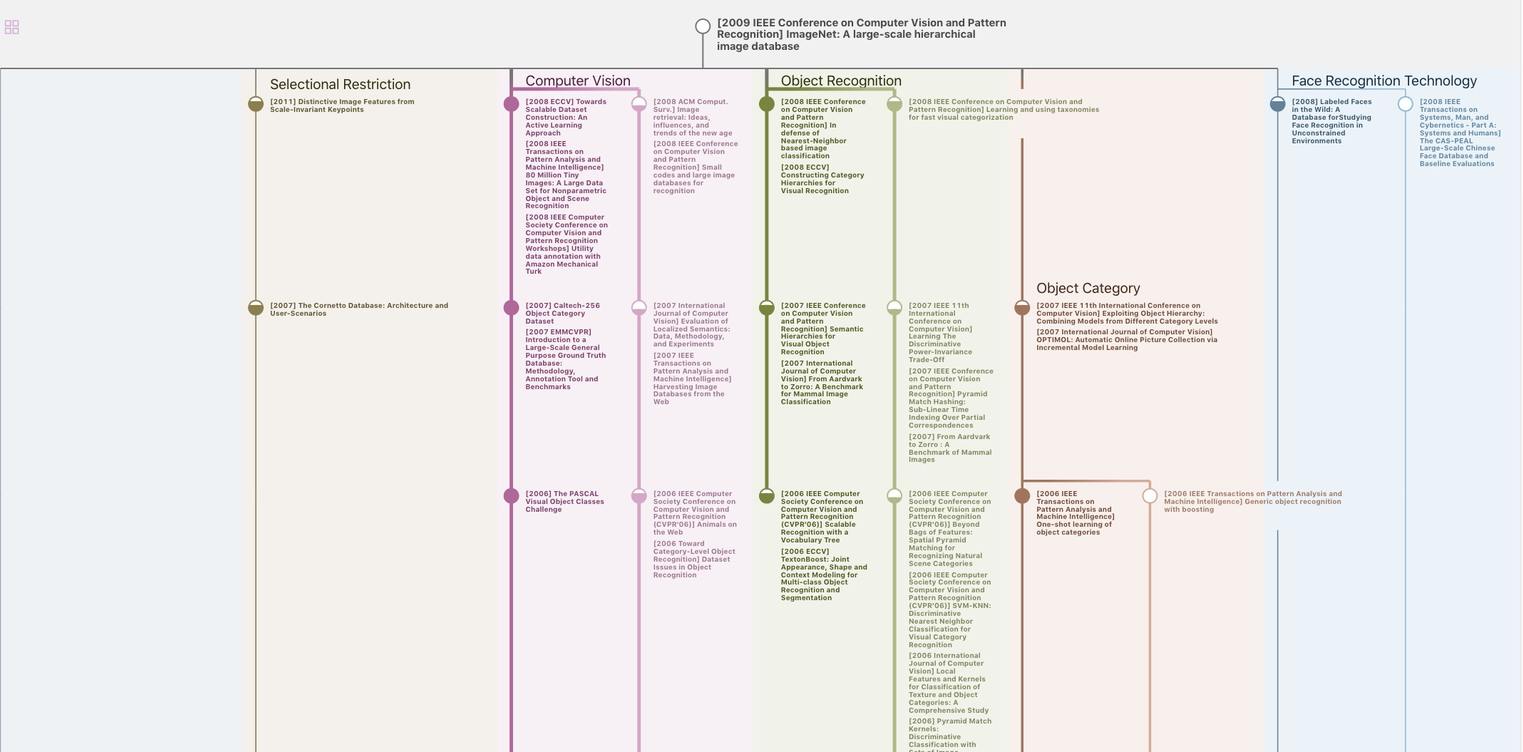
生成溯源树,研究论文发展脉络
Chat Paper
正在生成论文摘要