Mean–standard-deviation-based electric vehicle routing problem with time windows using Lagrangian relaxation and extended alternating direction method of multipliers-based decomposition algorithm
Soft Computing(2024)
摘要
In response to the challenges of global warming to sustainable development, electric vehicles (EVs) have become an important part of the supply chain. Numerous studies have focused on the electric vehicle routing problem with time windows (EVRPTW) to design an optimal routing plan for EVs and meet customers’ demands within th e specified time windows. However, most studies have been conducted in deterministic situations and neglected the uncertain travel time in reality. Therefore, this study proposes a novel mean–standard-deviation-based EVRPTW model and describes uncertain travel time with the expected travel time, travel time variance, and reliable parameters. Besides, the proposed model considers the partial recharge policy and capacitated recharge stations in an uncertain environment, and proposes a time-discrete state-space-time-energy representation network to better simulate real-world situations. To effectively solve the proposed model, a new Lagrangian relaxation and extended alternating direction method of multipliers (LR–EADMM)-based decomposition algorithm is proposed. In the LR–EADMM, dynamic penalty parameters and subgradient method are employed to update the Lagrangian multipliers, strengthen the algorithmic convergence, and improve the quality of solutions. Furthermore, to validate the performance of the proposed algorithm, several comparison experiments are conducted with the Gurobi optimizer and two baseline algorithms. With reliable parameter 0, the experimental results show that the average upper bounds obtained by LR–EADMM is 0.78% greater than the best-known solutions. With reliable parameters 1 and 2, the average gap between lower and upper bounds obtained by LR–EADMM is 8.08% smaller than that obtained by baseline algorithm in solving small instances, and the average upper bounds obtained by LR–EADMM is, respectively, 3.01% and 5.71% better than those obtained by two baseline algorithms in solving large instances.
更多查看译文
关键词
Electric vehicle routing problem with time windows,Uncertain travel time,Lagrangian relaxation,Extended alternating direction method of multipliers
AI 理解论文
溯源树
样例
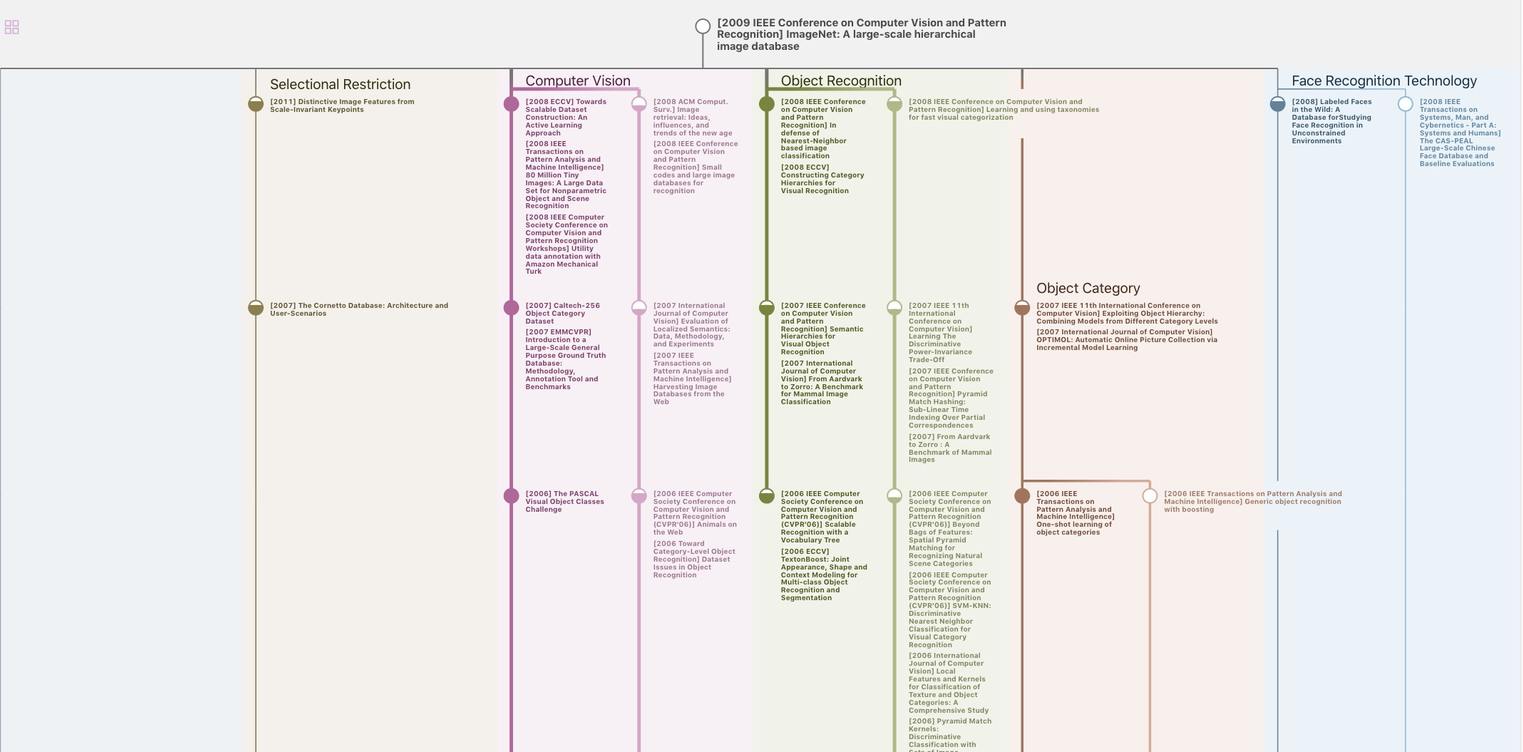
生成溯源树,研究论文发展脉络
Chat Paper
正在生成论文摘要