ZSG-ADR and GSQA: An unseen fault data generation and evaluation methods for the traction motors
MECHANICAL SYSTEMS AND SIGNAL PROCESSING(2024)
摘要
The collected fault data of the traction motors oftentimes contain limited fault types and fault degrees under specific conditions. This incompletion in data makes it challenging for existing intelligent fault diagnosis methods. In this paper, a method termed zero -shot generation with attribute -supervised disentangled representation (ZSG-ADR) is proposed to generate unseen fault samples for the traction motors in high-speed trains. First, attribute -supervised constraint terms are designed to encourage each attribute latent vector to learn the semantics of fault samples in traction motors. Second, a mutual information(MI) estimator is built to disentangle the learned attributes. In this way, a one-to-one correspondence between each attribute latent vector and fault semantics is established and an interpretable representation model is learned. In addition, to evaluate the performance of the proposed method, a series of comprehensive evaluation indexes named generated signal quality assessment (GSQA), including a new disentanglement index and an effectiveness metric is proposed. It is validated both on the real-time platform and the physical platform. Extensive experimental results suggest that the proposed method could learn a disentangled and interpretable fault sample representation and generate unseen fault samples with controllable fault types, fault degrees, and working conditions. Compared to the existing methods, the proposed method is most effective in dealing with incomplete fault datasets. Thus, it provides a solution for the problem of low diagnosis accuracy caused by incomplete data on the traction motor.
更多查看译文
关键词
Zero-shot generation,Fault data generation,Disentangled representation,Fault semantic,Generated signal quality assessment (GSQA),Traction motors
AI 理解论文
溯源树
样例
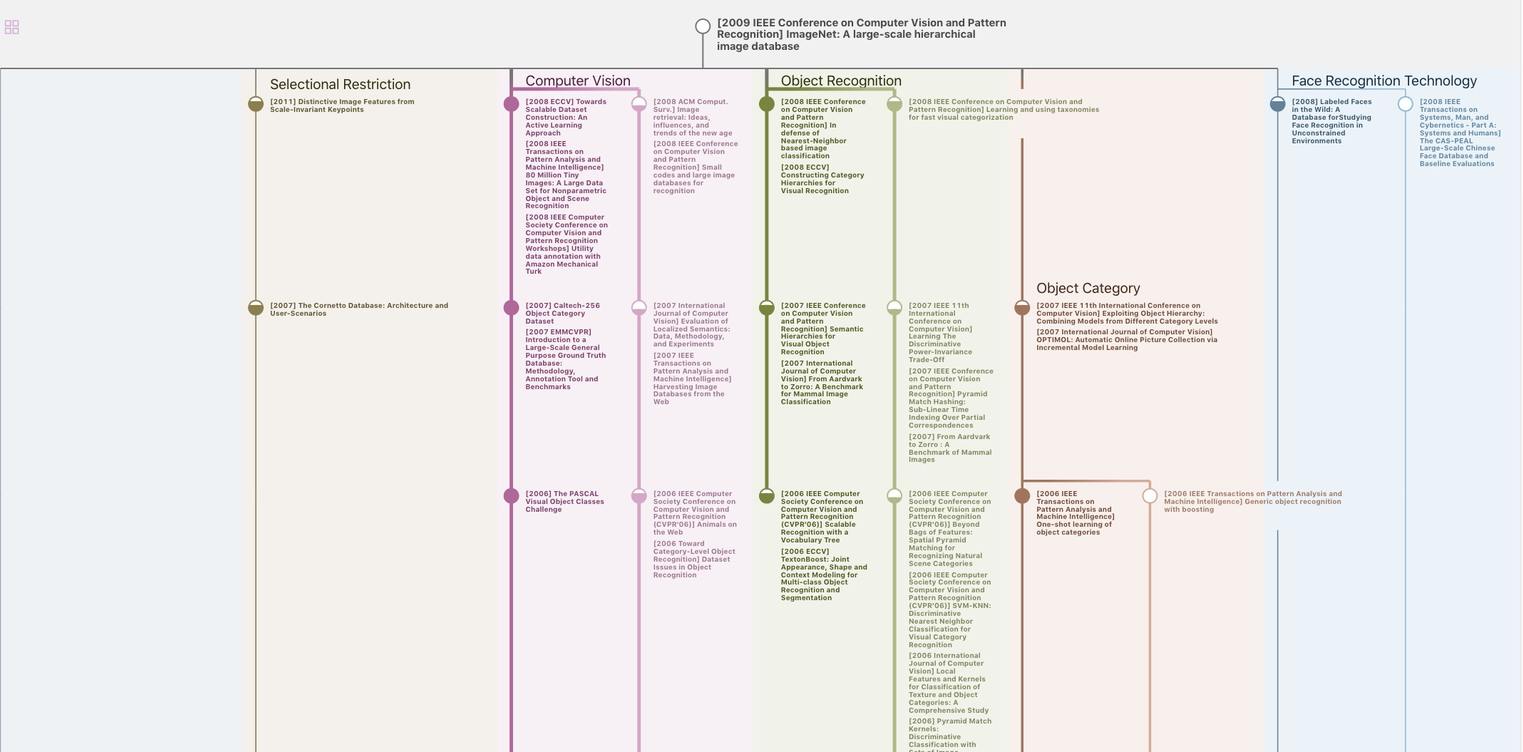
生成溯源树,研究论文发展脉络
Chat Paper
正在生成论文摘要