Optimizing magnetoelastic properties by machine learning and high-throughput micromagnetic simulation
Rare Metals(2024)
摘要
Magnetoelastic couplings in giant magnetostrictive materials (GMMs) attract significant interests due to their extensive applications in the fields of spintronics and energy harvesting devices. Understanding the role of the selection of materials and the response to external fields is essential for attaining desired functionality of a GMM. Herein, machine learning (ML) models are conducted to predict saturation magnetostrictions ( λ s ) in RFe 2 -type (R = rare earth) GMMs with different compositions. According to ML-predicted composition– λ s relations, it is discovered that the values of λ s higher than 1100 × 10 –6 are almost situated in the composition space surrounded by 0.26 ≤ x ≤ 0.60 and 1.90 ≤ y ≤ 2.00 for the ternary compounds of Tb x Dy 1- x Fe y . Assisted by ML predictions, the compositions are further narrowed down to the space surrounded by 0.26 ≤ x ≤ 0.32 and 1.92 ≤ y ≤ 1.97 for the excellent piezomagnetic (PM) performance in the Tb x Dy 1- x Fe y -based PM device through our developed high-throughput (HTP) micromagnetic simulation (MMS) algorithm. Accordingly, high sensitivities up to 10.22–13.61 mT·MPa –1 are observed in the optimized range within which the available experimental data fall well. This work not only provides valuable insights toward understanding the mechanism of magnetoelastic couplings, but also paves the way for designing and optimizing high-performance magnetostrictive materials and PM sensing devices. Graphical abstract
更多查看译文
关键词
Magnetostriction,Piezomagnetic effect,Machine learning,Micromagnetic simulation
AI 理解论文
溯源树
样例
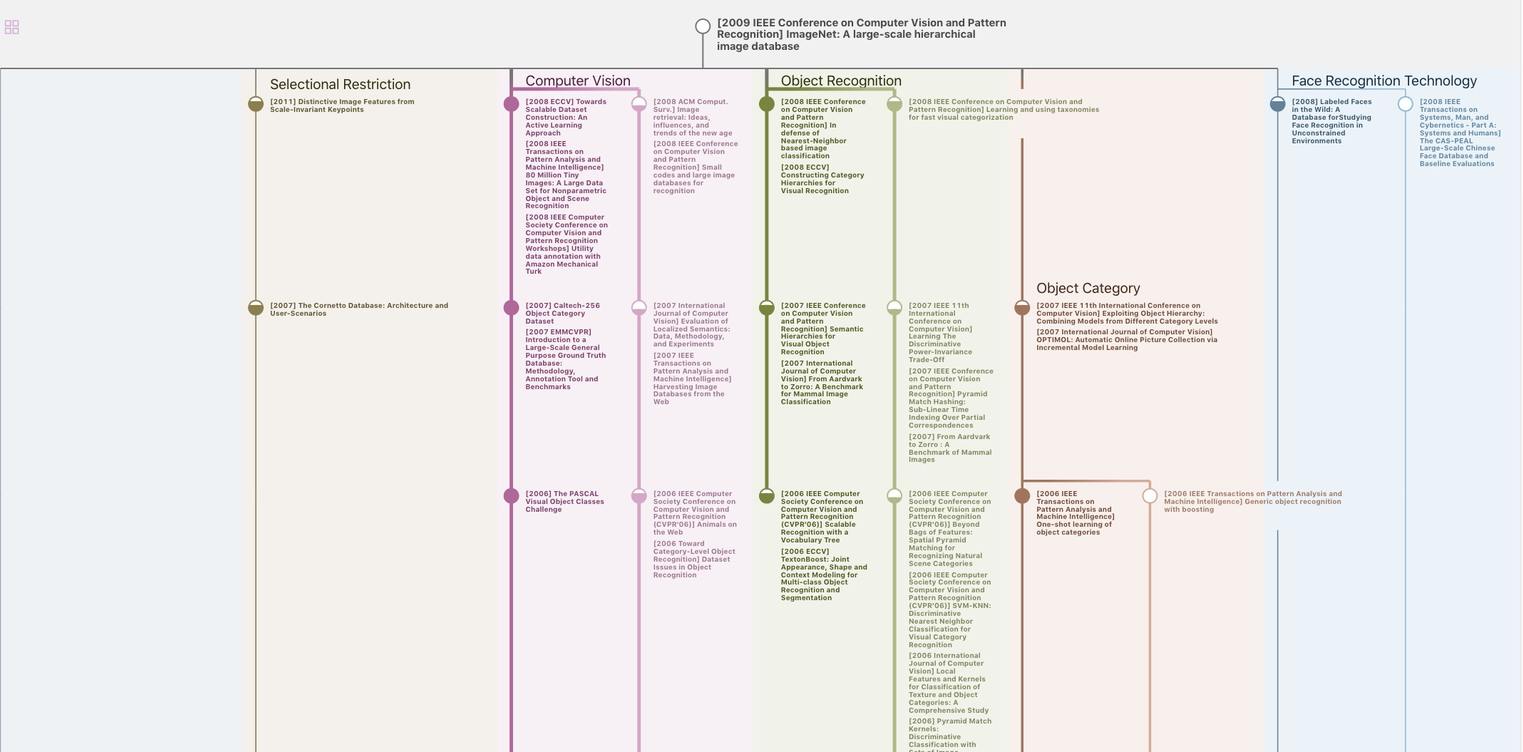
生成溯源树,研究论文发展脉络
Chat Paper
正在生成论文摘要