Interpretable machine learning model for prediction of overall survival in laryngeal cancer
ACTA OTO-LARYNGOLOGICA(2024)
摘要
Background: The mortality rates of laryngeal squamous cell carcinoma cancer (LSCC) have not significantly decreased in the last decades. Objectives: We primarily aimed to compare the predictive performance of DeepTables with the state-of-the-art machine learning (ML) algorithms (Voting ensemble, Stack ensemble, and XGBoost) to stratify patients with LSCC into chance of overall survival (OS). In addition, we complemented the developed model by providing interpretability using both global and local model-agnostic techniques. Methods: A total of 2792 patients in the Surveillance, Epidemiology, and End Results (SEER) database diagnosed with LSCC were reviewed. The global model-agnostic interpretability was examined using SHapley Additive exPlanations (SHAP) technique. Likewise, individual interpretation of the prediction was made using Local Interpretable Model Agnostic Explanations (LIME). Results: The state-of-the-art ML ensemble algorithms outperformed DeepTables. Specifically, the examined ensemble algorithms showed comparable weighted area under receiving curve of 76.9, 76.8, and 76.1 with an accuracy of 71.2%, 70.2%, and 71.8%, respectively. The global methods of interpretability (SHAP) demonstrated that the age of the patient at diagnosis, N-stage, T-stage, tumor grade, and marital status are among the prominent parameters. Conclusions: A ML model for OS prediction may serve as an ancillary tool for treatment planning of LSCC patients.
更多查看译文
关键词
Machine learning,deep learning,DeepTables,laryngeal cancer,laryngeal squamous cell carcinoma,XGBoost,voting ensemble,stacked ensemble,sEER,overall survival
AI 理解论文
溯源树
样例
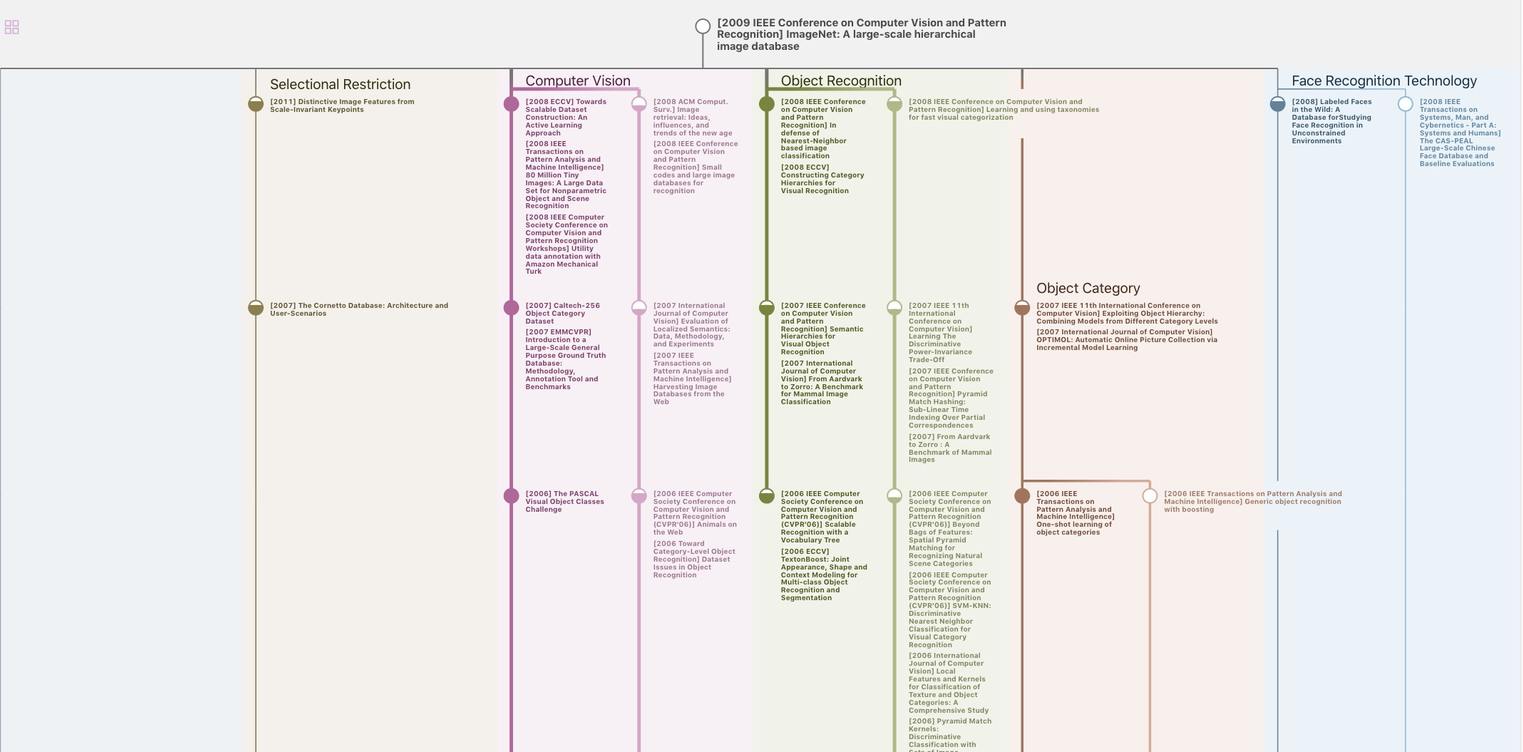
生成溯源树,研究论文发展脉络
Chat Paper
正在生成论文摘要