An innovative in silico model of the oral mucosa reveals the impact of extracellular spaces on chemical permeation through epithelium
arxiv(2024)
摘要
In pharmaceutical therapeutic design or toxicology, accurately predicting the
permeation of chemicals through human epithelial tissues is crucial, where
permeation is significantly influenced by the tissue's cellular architecture.
Current mathematical models for multi-layered epithelium such as the oral
mucosa only use simplistic 'bricks and mortar' geometries and therefore do not
account for the complex cellular architecture of these tissues at the
microscale level, such as the extensive plasma membrane convolutions that
define the extracellular spaces between cells. Chemicals often permeate tissues
via this paracellular route, meaning that permeation is underestimated. To
address this, measurements of human buccal mucosal tissue were conducted to
ascertain the width and tortuosity of extracellular spaces across the
epithelium. Using mechanistic mathematical modelling, we show that the
convoluted geometry of extracellular spaces significantly impacts chemical
permeation and that this can be approximated, provided that extracellular
tortuosity is accounted for. We next developed an advanced physically-relevant
in silico model of oral mucosal chemical permeation using partial differential
equations, fitted to chemical permeation in vitro assays on tissue-engineered
human oral mucosa. Tissue geometries were measured and captured in silico, and
permeation examined and predicted for chemicals with different physicochemical
properties. The effect of altering the extracellular space to mimic permeation
enhancers was also assessed by perturbing the in silico model. This novel in
vitro-in silico approach has the potential to expedite pharmaceutical
innovation for testing oromucosal chemical permeation, providing a more
accurate, physiologically-relevant model which can reduce animal testing with
early screening based on chemical properties.
更多查看译文
AI 理解论文
溯源树
样例
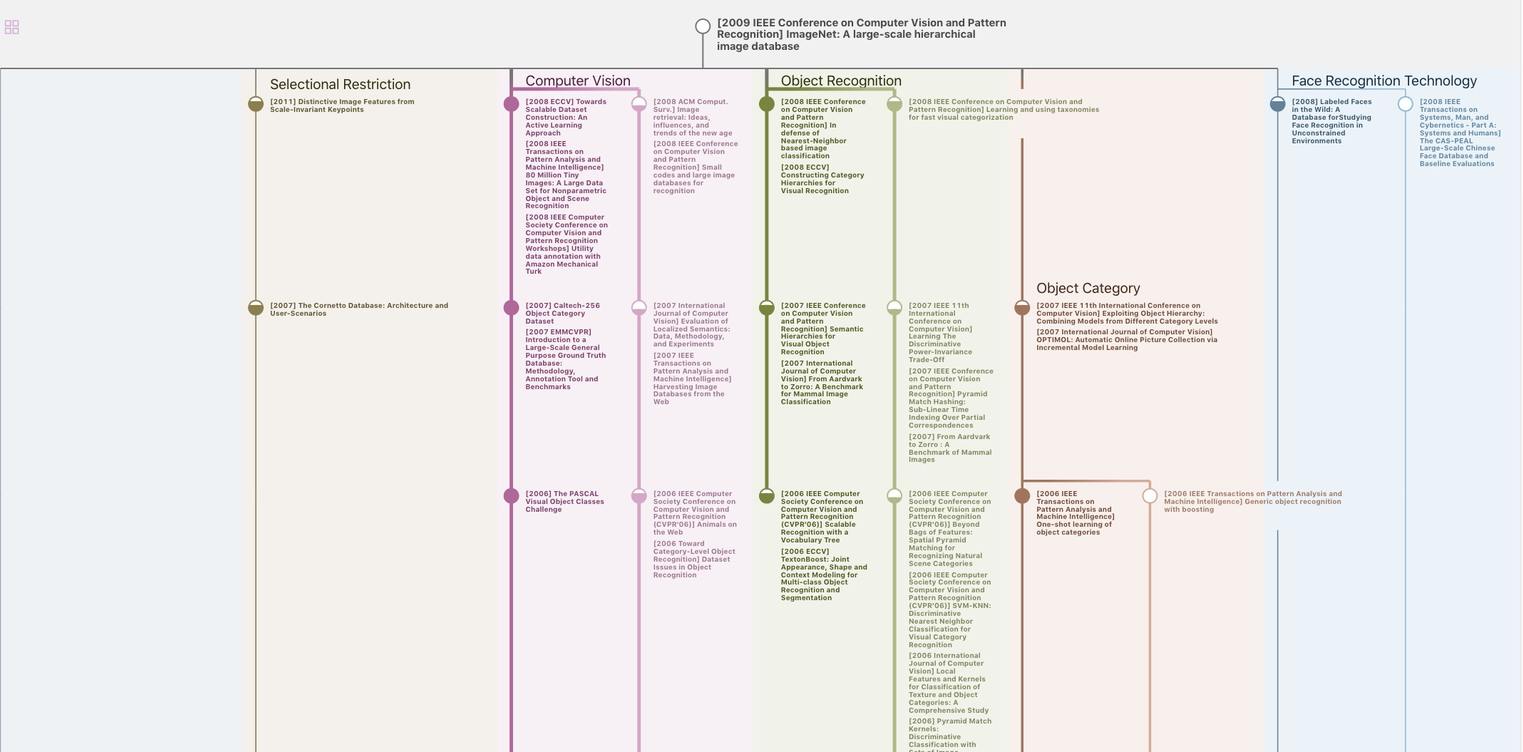
生成溯源树,研究论文发展脉络
Chat Paper
正在生成论文摘要