Expert with Clustering: Hierarchical Online Preference Learning Framework
arXiv (Cornell University)(2024)
摘要
Emerging mobility systems are increasingly capable of recommending options tomobility users, to guide them towards personalized yet sustainable systemoutcomes. Even more so than the typical recommendation system, it is crucial tominimize regret, because 1) the mobility options directly affect the lives ofthe users, and 2) the system sustainability relies on sufficient userparticipation. In this study, we consider accelerating user preference learningby exploiting a low-dimensional latent space that captures the mobilitypreferences of users. We introduce a hierarchical contextual bandit frameworknamed Expert with Clustering (EWC), which integrates clustering techniques andprediction with expert advice. EWC efficiently utilizes hierarchical userinformation and incorporates a novel Loss-guided Distance metric. This metricis instrumental in generating more representative cluster centroids. In arecommendation scenario with N users, T rounds per user, and K options,our algorithm achieves a regret bound of O(N√(Tlog K) + NT). This boundconsists of two parts: the first term is the regret from the Hedge algorithm,and the second term depends on the average loss from clustering. The algorithmperforms with low regret, especially when a latent hierarchical structureexists among users. This regret bound underscores the theoretical andexperimental efficacy of EWC, particularly in scenarios that demand rapidlearning and adaptation. Experimental results highlight that EWC cansubstantially reduce regret by 27.57offers a data-efficient approach to capturing both individual and collectivebehaviors, making it highly applicable to contexts with hierarchicalstructures. We expect the algorithm to be applicable to other settings withlayered nuances of user preferences and information.
更多查看译文
关键词
Regret Analysis,Context-Aware Recommender Systems,User Modeling,Bandit Optimization,Collaborative Filtering
AI 理解论文
溯源树
样例
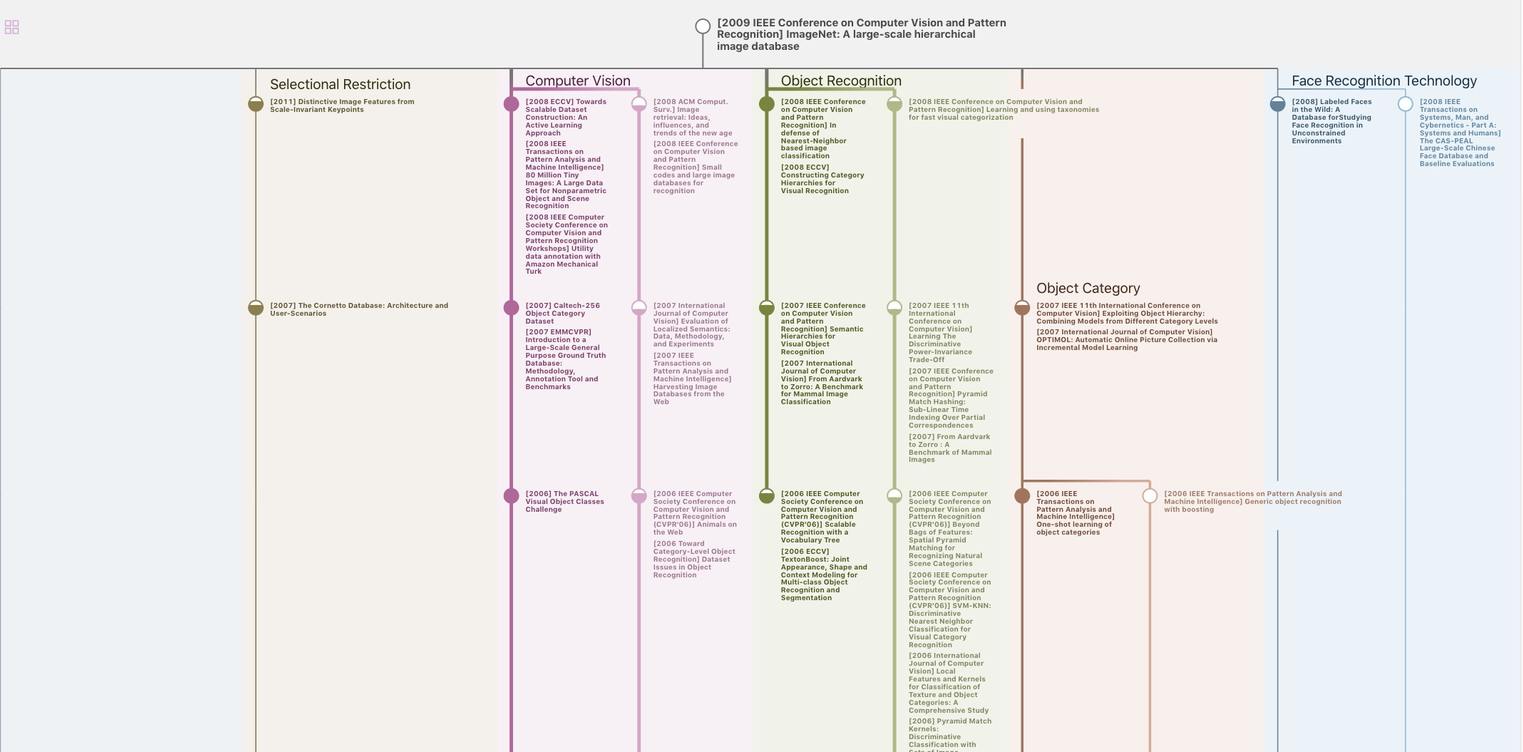
生成溯源树,研究论文发展脉络
Chat Paper
正在生成论文摘要