DEUNet: Dual-encoder UNet for simultaneous denosing and reconstruction of single HDR image
Computers & Graphics(2024)
摘要
High Dynamic Range (HDR) images have more powerful image expression capabilities and better visual quality than Low Dynamic Range (LDR) images, which can sufficiently represent real-world scenes, and will be widely used in the field of film and television in future. However, it is a very challenging task to generate an HDR image from a single-exposure LDR image. In this work, we propose a novel learning-based network, DEUNet, to reconstruct single-frame HDR image with simultaneous denoising and detail reconstruction. The proposed framework consists of two feature extraction branches, which can learn the brightness information and texture information separately for HDR image reconstruction. Each network branch is based on the UNet network structure and the two branches are interacted via spatial feature transformation. As a result, the proposed network can make full use of the multi-scale information at different levels of the image. In addition to the two encoding branches for feature extraction, the proposed network consists of another decoding network for fusing image brightness information and texture information, and a weighting network that selectively preserves most useful information. Compared with state-of-the-art methods, DEUNet can better reduce image noise while reconstructing the details in both the high and the low exposure areas. Experiments have shown that the proposed method achieves state-of-the-art performance on public datasets, indicating the effectiveness of the proposed method in this study.
更多查看译文
关键词
HDR reconstruction,Deep learning,Image denosing,Image enhancement
AI 理解论文
溯源树
样例
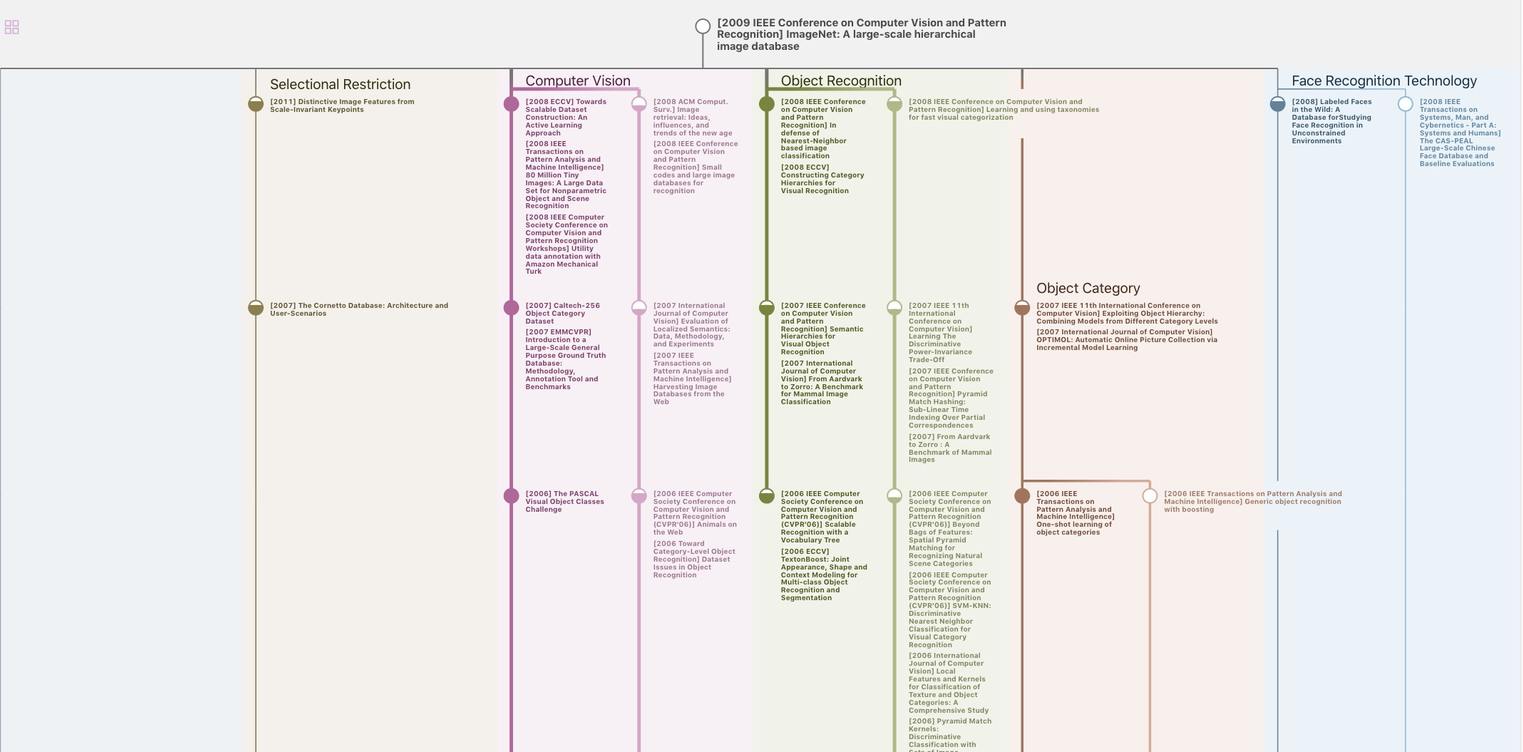
生成溯源树,研究论文发展脉络
Chat Paper
正在生成论文摘要