UX-MAPPER: A User Experience Method to Analyze App Store Reviews.
Simpósio Brasileiro de Fatores Humanos em Sistemas Computacionais(2023)
Departamento Acadêmico de Computação (DACOM) | Amazonas State Treasury Department (SEFAZ/AM) | Instituto de Computação (IComp)
Abstract
The mobile app market has grown over the last decades. With the rise of app stores, users can easily choose an app from thousands, making them less tolerant of low-quality apps. More than ever, users are looking for apps that provide not only valuable functionalities but pleasurable experiences. Hence, User eXperience (UX) became the differential to stand out from competitors. By understanding what factors affect UX, practitioners could focus on factors that lead to positive UX while mitigating those that affect UX negatively. In this context, reviews from app stores emerged as a valuable source of information to investigate such factors. However, analyzing millions of reviews is costly and time-consuming. This paper presents UX-MAPPER, an approach to analyzing app store reviews and supporting practitioners in identifying factors affecting UX. We applied the Design Science Research method to design UX-MAPPER iteratively and grounded on a solid theoretical background. We performed exploratory studies to investigate the problem, a systematic mapping study to identify factors that affect UX, and an empirical study with 14 participants with experience in requirements engineering to determine the relevance and acceptance of our proposal from practitioners’ perspectives. The participants considered it useful to improve the quality of existing apps and explore the reviews of competing apps to identify functionalities and features that users are requesting, liking, or hating. They were also willing to use it when it became available, highlighting our proposal’s usefulness and relevance in software development.
MoreTranslated text
求助PDF
上传PDF
View via Publisher
AI Read Science
AI Summary
AI Summary is the key point extracted automatically understanding the full text of the paper, including the background, methods, results, conclusions, icons and other key content, so that you can get the outline of the paper at a glance.
Example
Background
Key content
Introduction
Methods
Results
Related work
Fund
Key content
- Pretraining has recently greatly promoted the development of natural language processing (NLP)
- We show that M6 outperforms the baselines in multimodal downstream tasks, and the large M6 with 10 parameters can reach a better performance
- We propose a method called M6 that is able to process information of multiple modalities and perform both single-modal and cross-modal understanding and generation
- The model is scaled to large model with 10 billion parameters with sophisticated deployment, and the 10 -parameter M6-large is the largest pretrained model in Chinese
- Experimental results show that our proposed M6 outperforms the baseline in a number of downstream tasks concerning both single modality and multiple modalities We will continue the pretraining of extremely large models by increasing data to explore the limit of its performance
Upload PDF to Generate Summary
Must-Reading Tree
Example
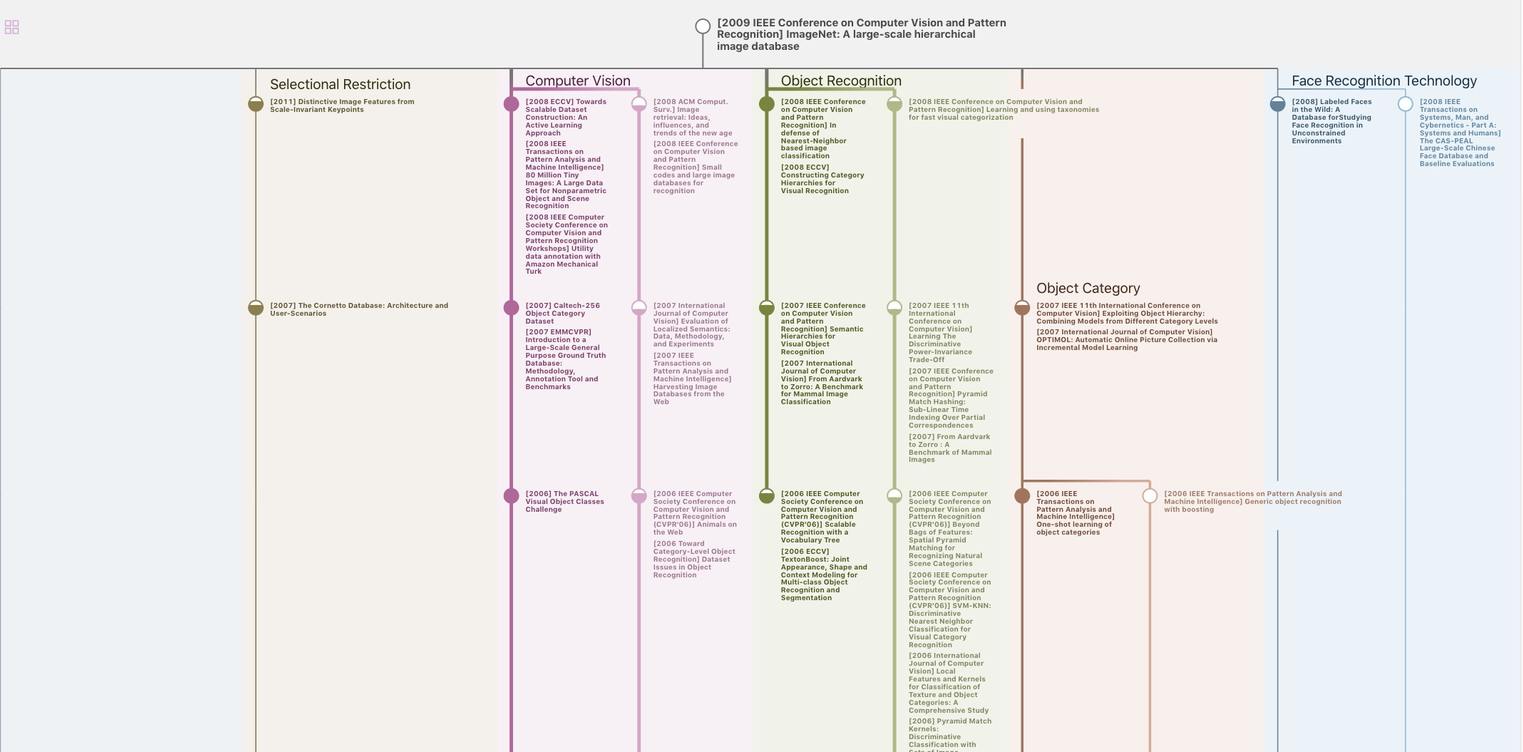
Generate MRT to find the research sequence of this paper
Related Papers
2002
被引用121 | 浏览
2016
被引用30 | 浏览
Modeling User Satisfaction from the Extraction of User Experience Elements in Online Product Reviews
2017
被引用24 | 浏览
2017
被引用23 | 浏览
Data Disclaimer
The page data are from open Internet sources, cooperative publishers and automatic analysis results through AI technology. We do not make any commitments and guarantees for the validity, accuracy, correctness, reliability, completeness and timeliness of the page data. If you have any questions, please contact us by email: report@aminer.cn
Chat Paper
GPU is busy, summary generation fails
Rerequest