Enhancing wind speed forecasting through synergy of machine learning, singular spectral analysis, and variational mode decomposition
ENERGY(2024)
摘要
Due to technological advancements, wind energy has emerged as a prominent renewable power source. However, the intermittent nature of wind poses challenges in accurately predicting wind speeds, affecting the operation of electric systems. With the Brazilian electricity market transition to hourly electricity pricing, there is a growing need for forecasting tools to enhance wind farm operation and maintenance schedules. To address this issue, this research explores the performance of forecasting models enhanced with a decomposition stage to mitigate wind speed forecast errors. The study considers two decomposition techniques: singular spectral analysis in the time domain and variational mode decomposition in the frequency domain, combined in a hybrid structure incorporating multi -stage decomposition and time series prediction. The study focuses on hourly forecasting horizons of 24, 48, and 72 steps ahead. The autoregressive recurrent neural network achieved a MAPE of 10.92% utilizing decomposition for the 72 -hour forecast from January to December 2015, this represents a 51.16% improvement compared to the original structure. The outcomes of all the assessed models employing decomposition demonstrate a decrease in error in comparison to those without decomposition, thereby confirming the potential of hybrid architectures. Forecasting approaches can contribute to improved wind farm operation planning and maintenance scheduling.
更多查看译文
关键词
Machine learning,Time series decomposition,Variational mode decomposition,Singular spectrum analysis,Hybrid model,Wind speed forecasting
AI 理解论文
溯源树
样例
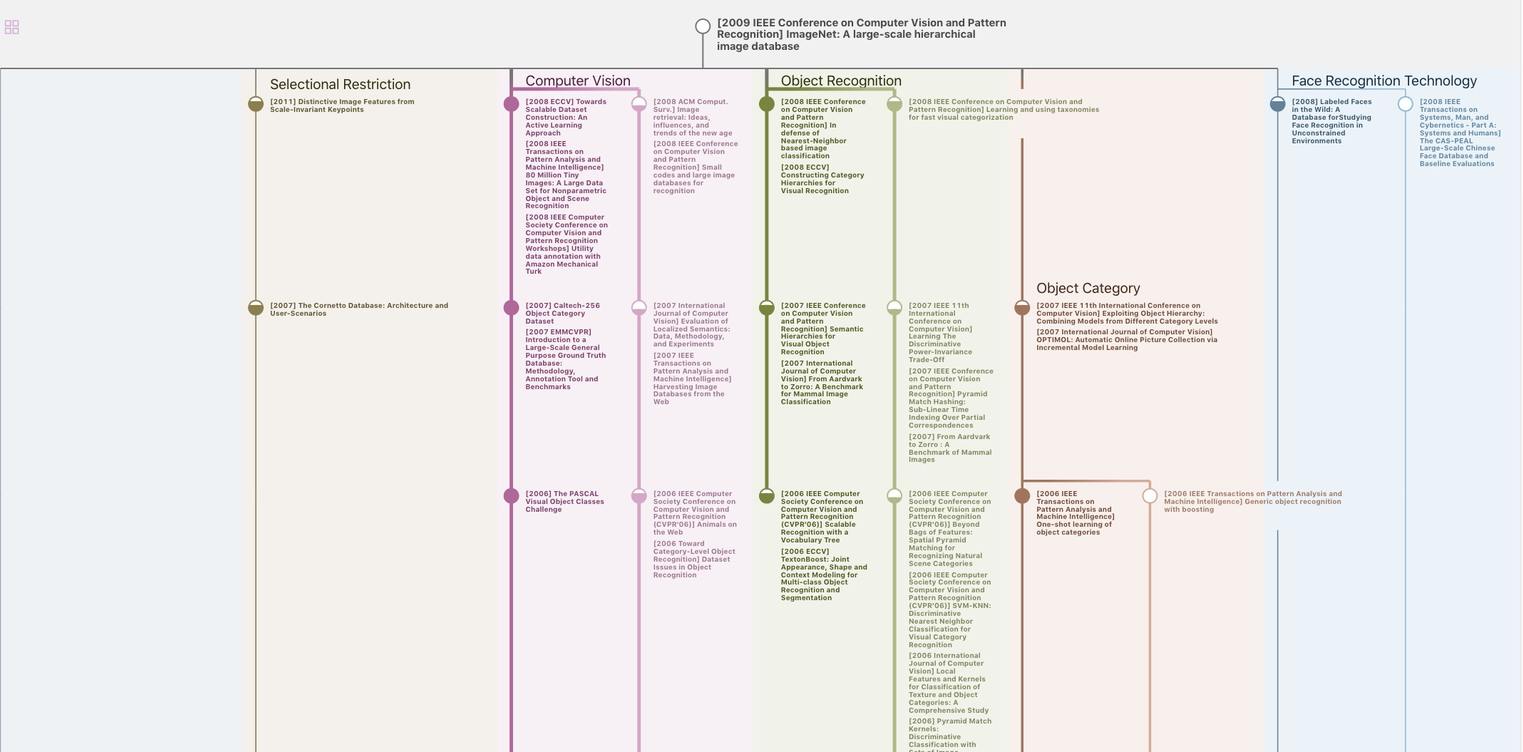
生成溯源树,研究论文发展脉络
Chat Paper
正在生成论文摘要