Data-driven model to predict the residual drift of precast concrete columns
JOURNAL OF BUILDING ENGINEERING(2024)
Abstract
Precast concrete columns (PCCs) are deemed to have self-centering capacity to reduce residual drift (RD), which means improved post-earthquake safety and trimmed seismic repair cost. However, the RD of PCCs is not well controlled through a design process due to the lack of prediction methods with an acceptable agreement with existing test results. This study aims to develop a data-driven model to accurately and reliably predict the RD of PCCs using the XGBoost algorithm. A RD database was established including 1136 data points with 43 features extracted from 177 flexural-dominant PCC specimens. Feature selection and hyperparameter tuning were adopted to optimize a XGBoost model based on the RD database, and a series of performance indicators were used to evaluate the proposed XGBoost model with feature selection, which was further compared with five existing empirical formulas. SHapley Additive exPlanations (SHAP) analysis was conducted to interpret the proposed model. Results showed that ten features are identified noticeably influential by the feature selection and considered in the proposed XGBoost model. The proposed model presents excellent prediction performance with high accuracy, lower variance, and acceptable guarantee rates, and it far outperforms the existing empirical formulas. According to the SHAP analysis results, new findings concerning the effects of the features on the RD of PCCs can be obtained, and there is high dependency between some couples of the selected features. This study provides a powerful tool to control the RD by fully taking advantage of selfcentering capacity in the seismic design of PCCs.
MoreTranslated text
Key words
Precast concrete columns,Residual drift,Machine learning (ML),eXtreme gradient boosting (XGBoost),SHapley additive exPlanations (SHAP)
AI Read Science
Must-Reading Tree
Example
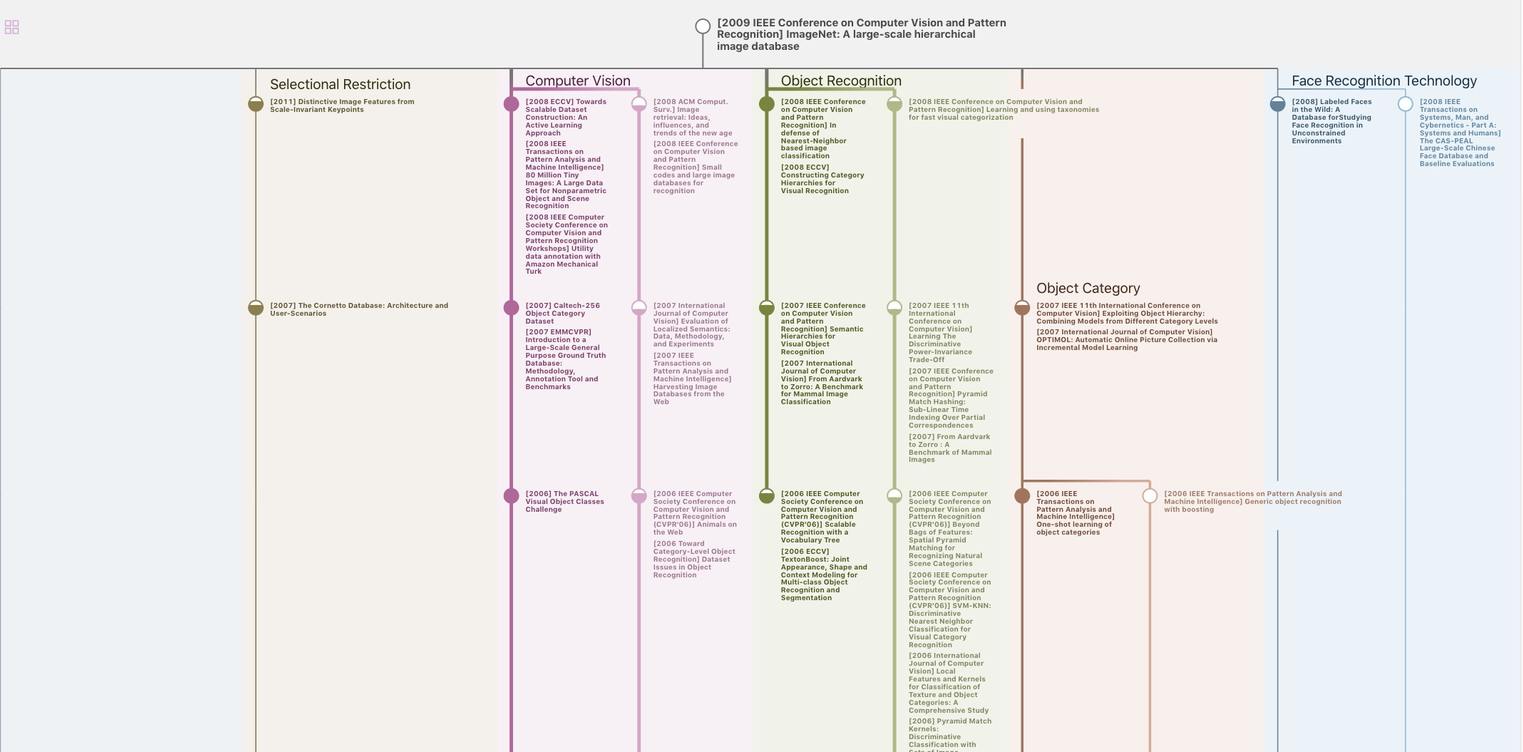
Generate MRT to find the research sequence of this paper
Chat Paper
Summary is being generated by the instructions you defined