Enhancing streamflow simulation in large and human-regulated basins: Long short-term memory with multiscale attributes
JOURNAL OF HYDROLOGY(2024)
摘要
Streamflow simulation in human-regulated catchments is a great challenge for both process-based hydrological models and deep learning (DL) methods, mainly because human-regulation rules are difficult to parameterize in these models. In this study, we investigate the roles of river and catchment attributes in DL for streamflow prediction. We evaluate a typical DL method, i.e., long short-term memory (LSTM), and evaluate its performance in 25 large catchments across the Yellow River Basin where human activities are intensive, especially with large numbers of dams and reservoirs influencing streamflow processes. For the LSTM forcing data, we compare two forcing datasets: the Fifth Generation of European Reanalysis (ERA5-Land) and meteorological station-based data. The results show that the LSTM forced by ERA5-Land achieves improved performance, as its mean Kling-Gupta efficiency (KGE) is 0.21 relative to the mean KGE of 0.08 from the meteorological station forced LSTM. Integrating different types of hydrological attributes (catchment and river characteristics) can substantially improve LSTM performance even for catchments with dams and reservoirs. The river-reach attributes show the largest contribution to the LSTM model improvement. Moreover, LSTM with multiscale attributes outperforms a global process-based hydrological model (LISFLOOD) in the middle and lower reaches of the Yellow River Basin. Our study indicates that multiscale attributes are promising pivots for DL methods to improve streamflow prediction in human-regulated basins.
更多查看译文
关键词
LSTM,LISFLOOD,Multiscale static attributes,Streamflow simulation,Human activities,Yellow River Basin
AI 理解论文
溯源树
样例
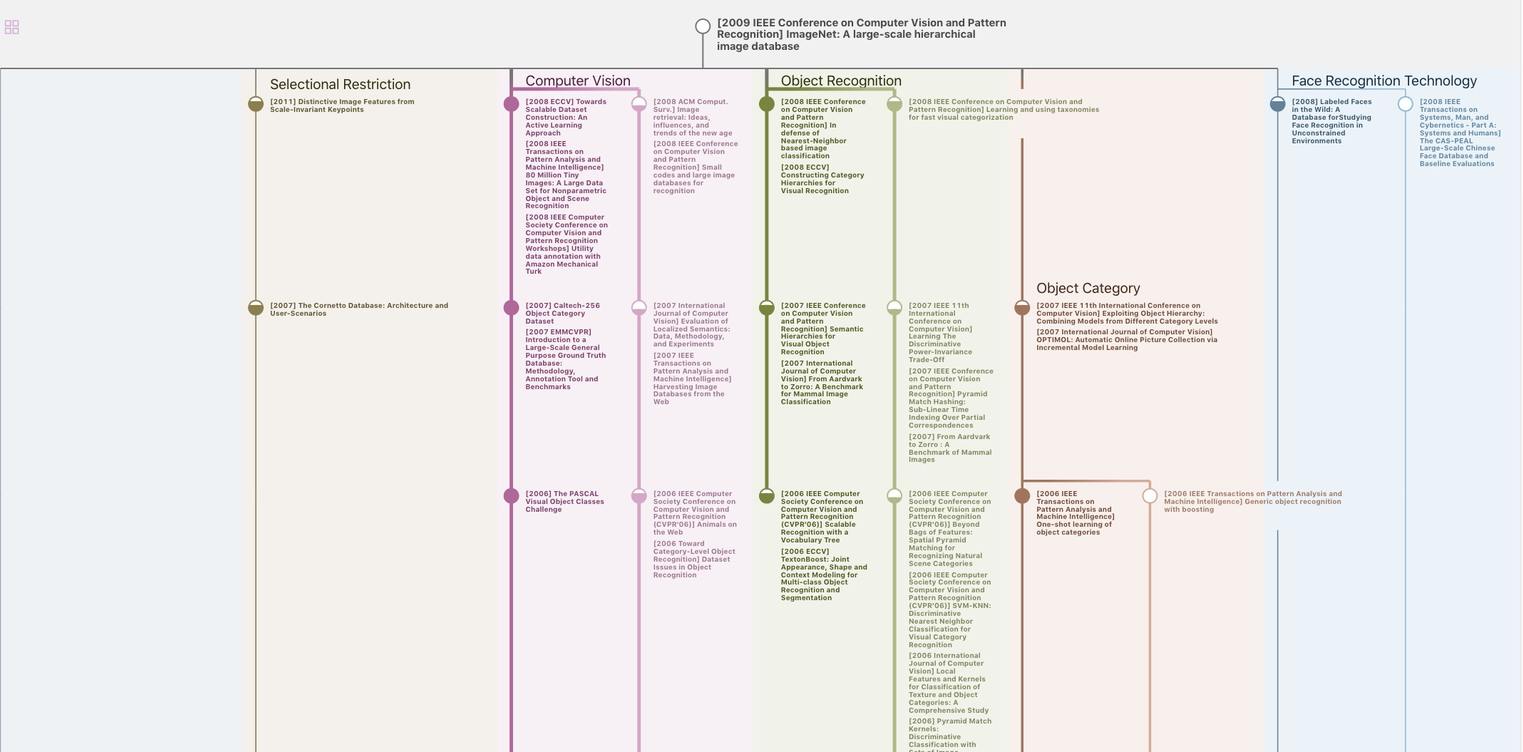
生成溯源树,研究论文发展脉络
Chat Paper
正在生成论文摘要