Automatic classification of neurological voice disorders using wavelet scattering features
SPEECH COMMUNICATION(2024)
摘要
Neurological voice disorders are caused by problems in the nervous system as it interacts with the larynx. In this paper, we propose to use wavelet scattering transform (WST)-based features in automatic classification of neurological voice disorders. As a part of WST, a speech signal is processed in stages with each stage consisting of three operations - convolution, modulus and averaging - to generate low -variance data representations that preserve discriminability across classes while minimizing differences within a class. The proposed WST-based features were extracted from speech signals of patients suffering from either spasmodic dysphonia (SD) or recurrent laryngeal nerve palsy (RLNP) and from speech signals of healthy speakers of the Saarbruecken voice disorder (SVD) database. Two machine learning algorithms (support vector machine (SVM) and feed forward neural network (NN)) were trained separately using the WST-based features, to perform two binary classification tasks (healthy vs. SD and healthy vs. RLNP) and one multi -class classification task (healthy vs. SD vs. RLNP). The results show that WST-based features outperformed state-of-the-art features in all three tasks. Furthermore, the best overall classification performance was achieved by the NN classifier trained using WST-based features.
更多查看译文
关键词
Wavelet scattering transform,Support vector machine,Neural network,Neurological voice disorder,Spasmodic dysphonia,MFCC
AI 理解论文
溯源树
样例
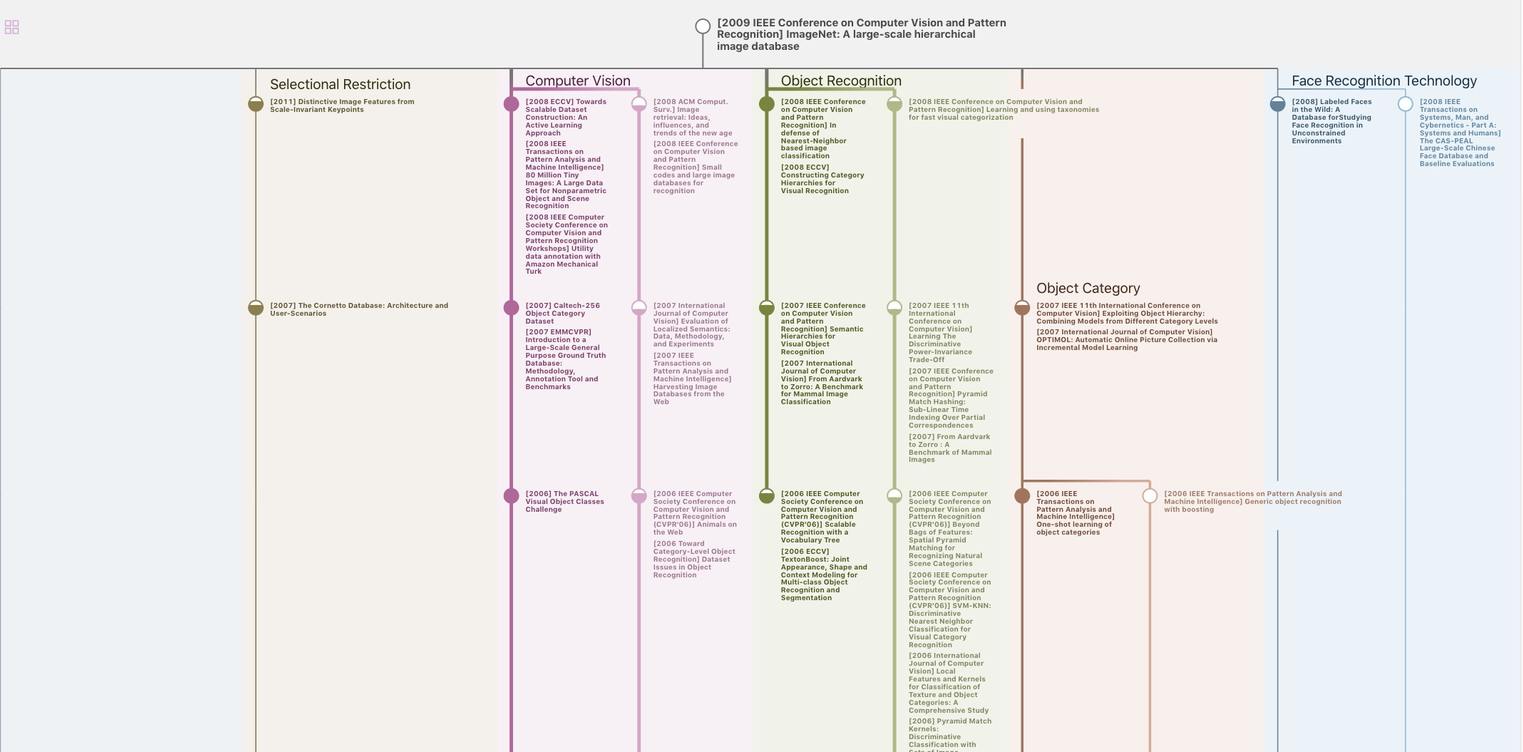
生成溯源树,研究论文发展脉络
Chat Paper
正在生成论文摘要