A joint optimization approach for personalized recommendation diversification
Lecture Notes in Computer Science(2018)
摘要
© Springer International Publishing AG, part of Springer Nature 2018. In recommendation systems, items of interest are often classified into categories such as genres of movies. Existing research has shown that diversified recommendations can improve real user experience. However, most existing methods do not consider the fact that users’ levels of interest (i.e., user preferences) in different categories usually vary, and such user preferences are not reflected in the diversified recommendations. We propose an algorithm that considers user preferences for different categories when recommending diversified results, and refer to this problem as personalized recommendation diversification. In the proposed algorithm, a model that captures user preferences for different categories is optimized jointly toward both relevance and diversity. To provide the proposed algorithm with informative training labels and effectively evaluate recommendation diversity, we also propose a new personalized diversity measure. The proposed measure overcomes limitations of existing measures in evaluating recommendation diversity: existing measures either cannot effectively handle user preferences for different categories, or cannot evaluate both relevance and diversity at the same time. Experiments using two real-world datasets confirm the superiority of the proposed algorithm, and show the effectiveness of the proposed measure in capturing user preferences.
更多查看译文
关键词
personalized recommendation diversification,joint optimization approach
AI 理解论文
溯源树
样例
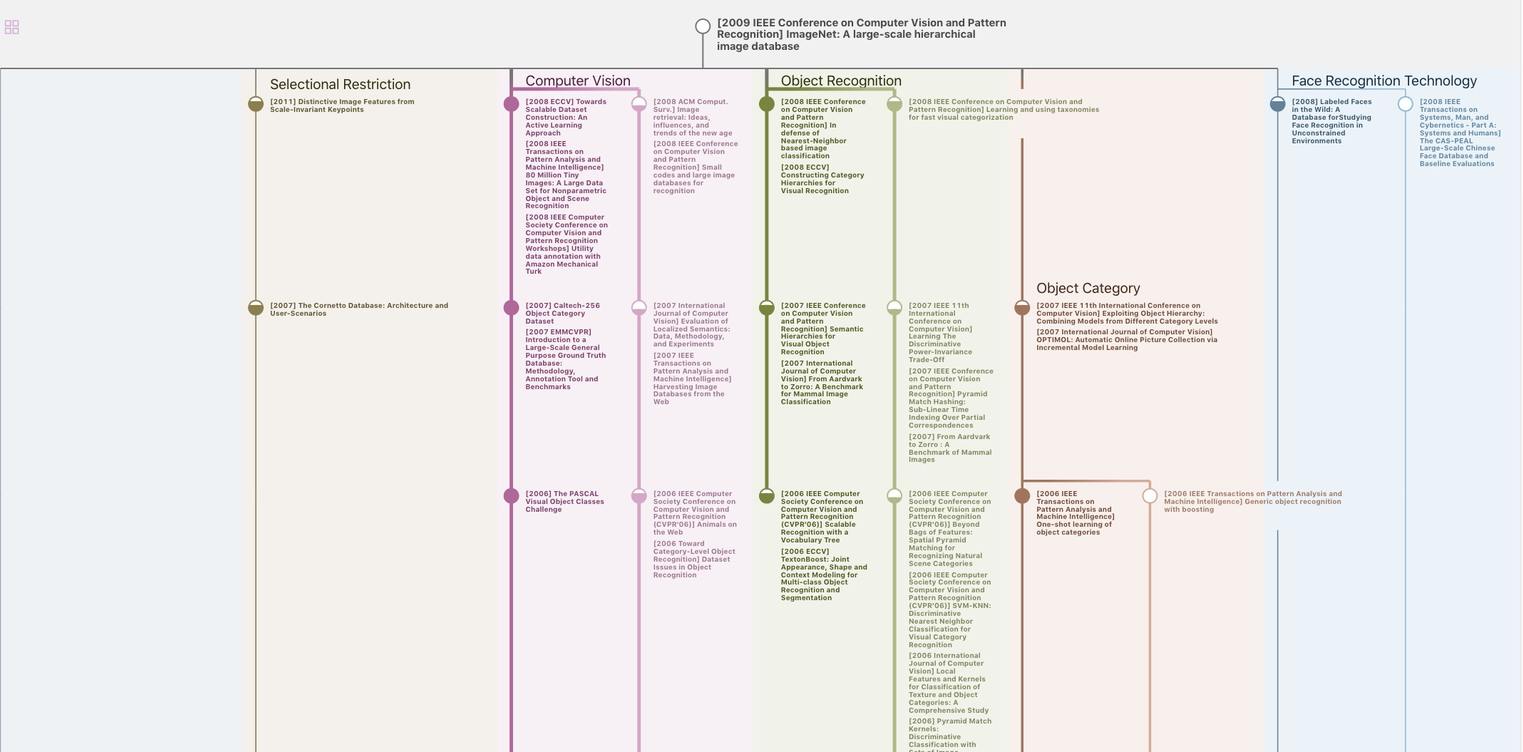
生成溯源树,研究论文发展脉络
Chat Paper
正在生成论文摘要