Federated Learning-based Information Leakage Risk Detection for Secure Medical Internet of ThingsJust Accepted
ACM Transactions on Internet Technology(2023)
摘要
The Medical Internet of Things (MIoT) requires extreme information and communication security, particularly for remote consultation systems. MIoT’s integration of physical and computational components creates a seamless network of medical devices providing high-quality care via continuous monitoring and treatment. However, traditional security methods such as cryptography cannot prevent privacy compromise and information leakage caused by security breaches. To solve this issue, this paper proposes a novel Federated Learning Intrusion Detection System (FLIDS). FLIDS combines Generative Adversarial Network (GAN) and Federated Learning (FL) to detect cyber attacks like Denial of Service (DoS), data modification, and data injection using machine learning. FLIDS shows exceptional performance with over 99% detection accuracy and 1% False Positive Rate (FPR). It saves bandwidth by transmitting 3.8 times fewer bytes compared to central data collection. These results prove FLIDS’ effectiveness in detecting and mitigating security threats in Medical Cyber-Physical Systems (MCPS). The paper recommends scaling up FLIDS to use computing resources from multiple mobile devices for better intrusion detection accuracy and efficiency while reducing the burden on individual devices in MIoT.
更多查看译文
关键词
Federated Learning,Intrusion Detection System,Medical Internet of Things,GAN-based Privacy Protection,Data Leakage,Discriminator Network,Dataset Construction,Training Accuracy,False Positive Rate,Patient Data.
AI 理解论文
溯源树
样例
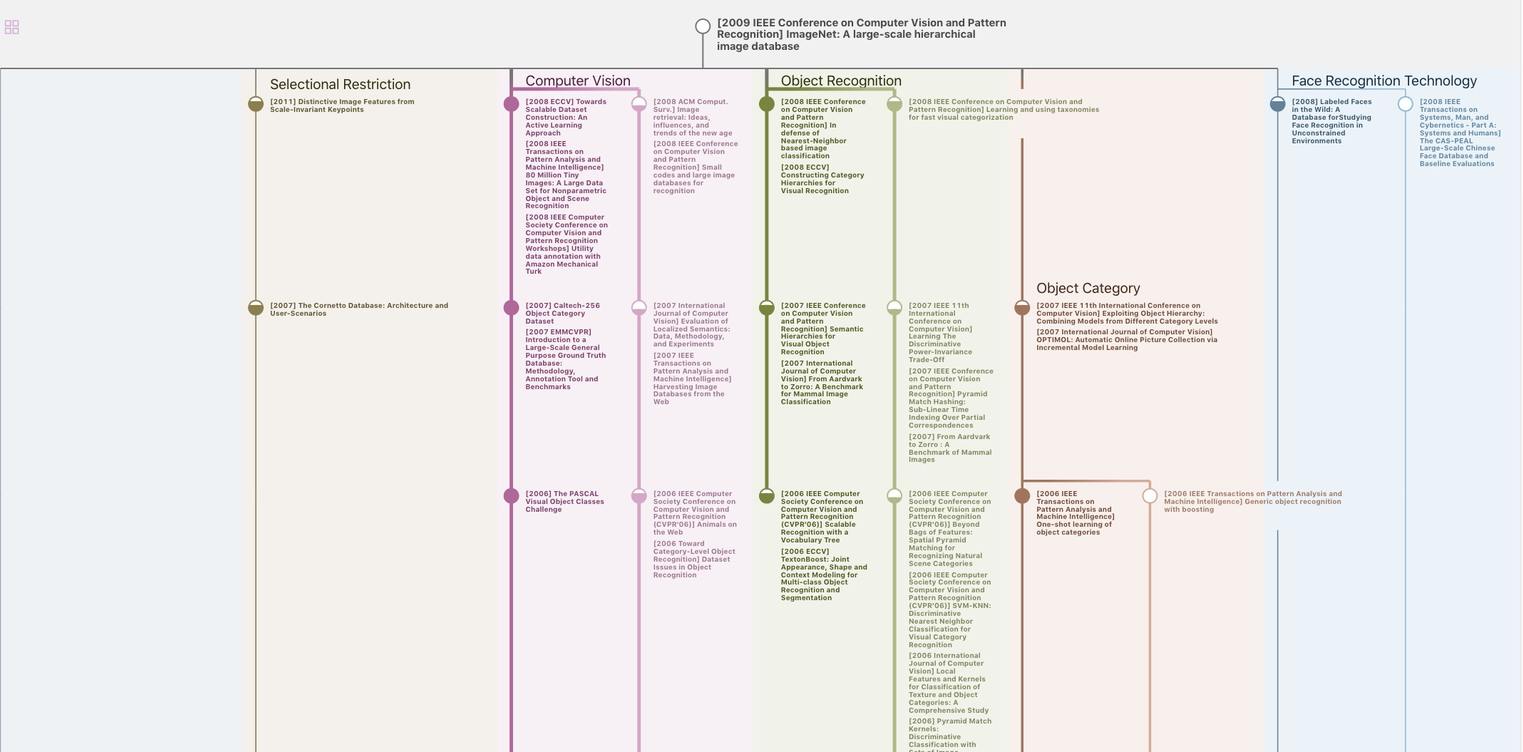
生成溯源树,研究论文发展脉络
Chat Paper
正在生成论文摘要