Utility-aware Privacy Perturbation for Training DataJust Accepted
ACM Transactions on Knowledge Discovery from Data(2022)
摘要
Data perturbation under differential privacy constraint is an important approach of protecting data privacy. However, as the data dimensions increase, the privacy budget allocated to each dimension decreases and thus the amount of noise added increases, which eventually leads to lower data utility in training tasks. To protect the privacy of training data while enhancing data utility, we propose an Utility-aware training data Privacy Perturbation scheme based on attribute Partition and budget Allocation (UPPPA). UPPPA includes three procedures, the quantification of attribute privacy and attribute importance, attribute partition, and budget allocation. The quantification of attribute privacy and attribute importance based on information entropy and attribute correlation provide an arithmetic basis for attribute partition and budget allocation. During the attribute partition, all attributes of training data are classified into high and low classes to achieve privacy amplification and utility enhancement. During the budget allocation, a γ -privacy model is proposed to balance data privacy and data utility so as to provide privacy constraint and guide budget allocation. Three comprehensive sets of real-world data are applied to evaluate the performance of UPPPA. Experiments and privacy analysis show that our scheme can achieve the tradeoff between privacy and utility.
更多查看译文
关键词
Training data privacy,Data perturbation,Data utility.
AI 理解论文
溯源树
样例
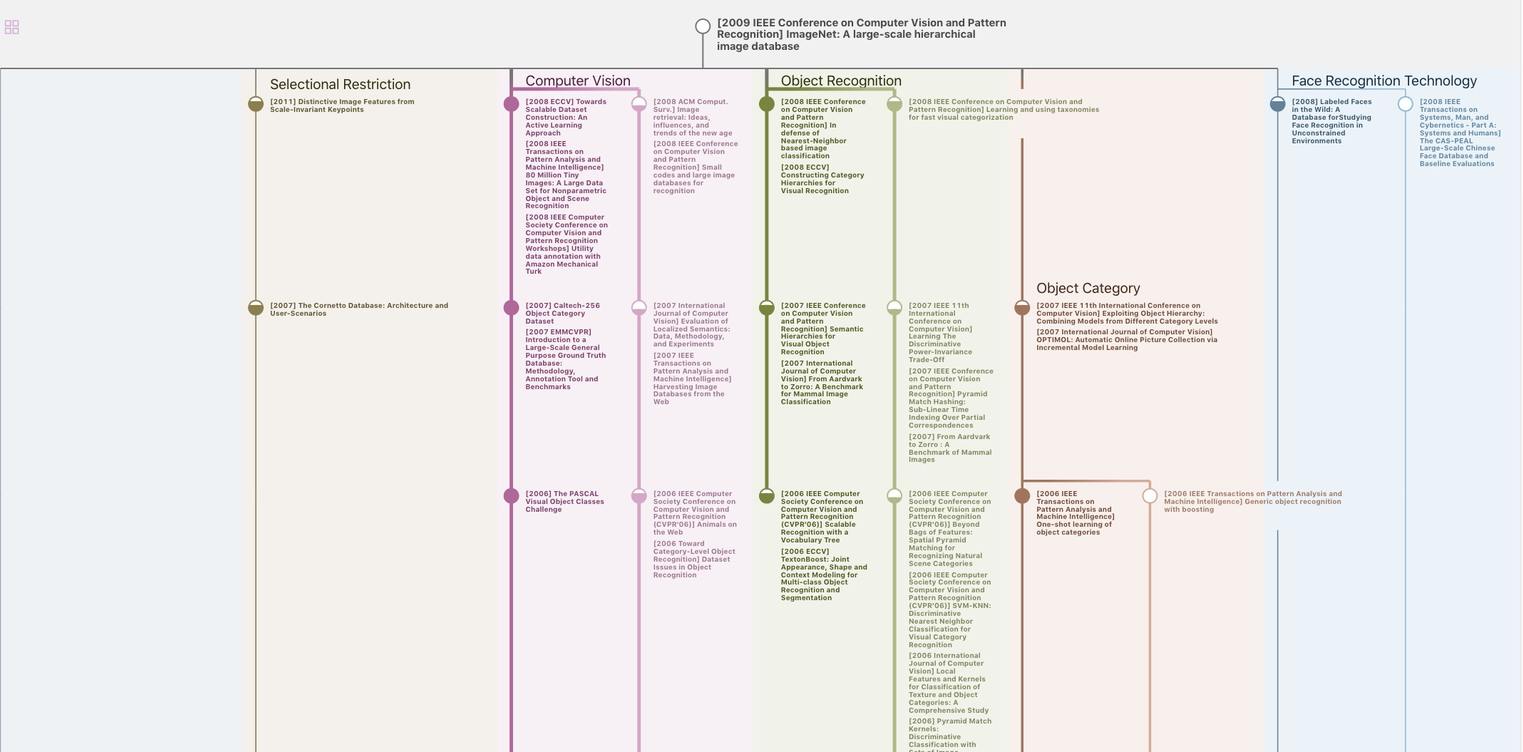
生成溯源树,研究论文发展脉络
Chat Paper
正在生成论文摘要