Explainable Reinforcement Learning: A Survey and Comparative ReviewJust Accepted
ACM Computing Surveys(2022)
摘要
Explainable reinforcement learning (XRL) is an emerging subfield of explainable machine learning that has attracted considerable attention in recent years. The goal of XRL is to elucidate the decision-making process of reinforcement learning (RL) agents in sequential decision-making settings. Equipped with this information, practitioners can better understand important questions about RL agents (especially those deployed in the real world), such as what the agents will do and why. Despite increased interest, there exists a gap in the literature for organizing the plethora of papers — especially in a way that centers the sequential decision-making nature of the problem. In this survey, we propose a novel taxonomy for organizing the XRL literature that prioritizes the RL setting. We propose three high-level categories: feature importance, learning process and Markov decision process, and policy-level. We overview techniques according to this taxonomy, highlighting challenges and opportunities for future work. We conclude by using these gaps to motivate and outline a roadmap for future work.
更多查看译文
关键词
explainable reinforcement learning,interpretability,explainability
AI 理解论文
溯源树
样例
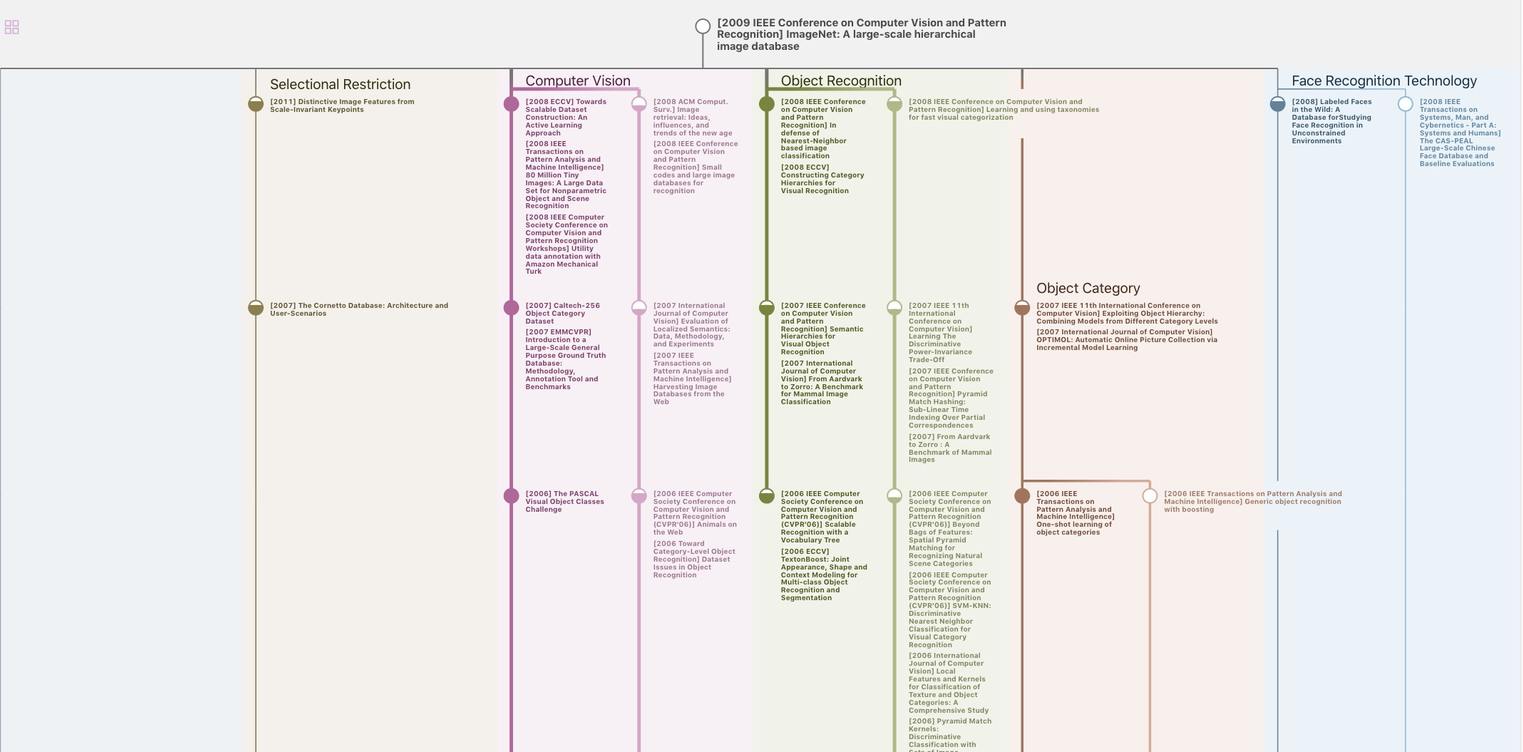
生成溯源树,研究论文发展脉络
Chat Paper
正在生成论文摘要