Dynamic Bi-layer Graph Learning for Context-aware Sequential RecommendationJust Accepted
ACM Transactions on Recommender Systems(2023)
摘要
Sequential recommendations have received great attention in recent years due to their wide application in e-commerce, trip planning, and online education. Contexts reveal the intention of a user in a transaction such as consuming or purchasing an item, and highly affect her behaviour. However, existing techniques cannot fully capture the context-sensitive and dynamic sequential user behaviours for effective and efficient sequential recommendation. To address this limitation, we propose a novel Dynamic Bi-layer Graph learning (DBIG)-based framework for sequential recommendation, which learns the sequence dynamics of user behaviours and contexts. Specifically, we first propose a context graph to identify the relevant contexts to a target user. Then, we propose a transaction context graph that predicts the probability of a transaction happening under certain contexts. In addition, we apply BERT (Bidirectional Encoder Representations from Transformers) to our graph embedding for understanding the user sequential behaviours in bidirectional ways and generating recommendations. Finally, we propose a cluster-structure-based algorithm, which incrementally maintains the DBIG model and BERT over dynamic transaction updates. We evaluate our DBIG-based framework by conducting extensive experiments over five public datasets. The quantitative results demonstrate the superiority of our approach in terms of effectiveness and efficiency.
更多查看译文
关键词
Sequential Recommendation,Dynamic Bi-layer Graph
AI 理解论文
溯源树
样例
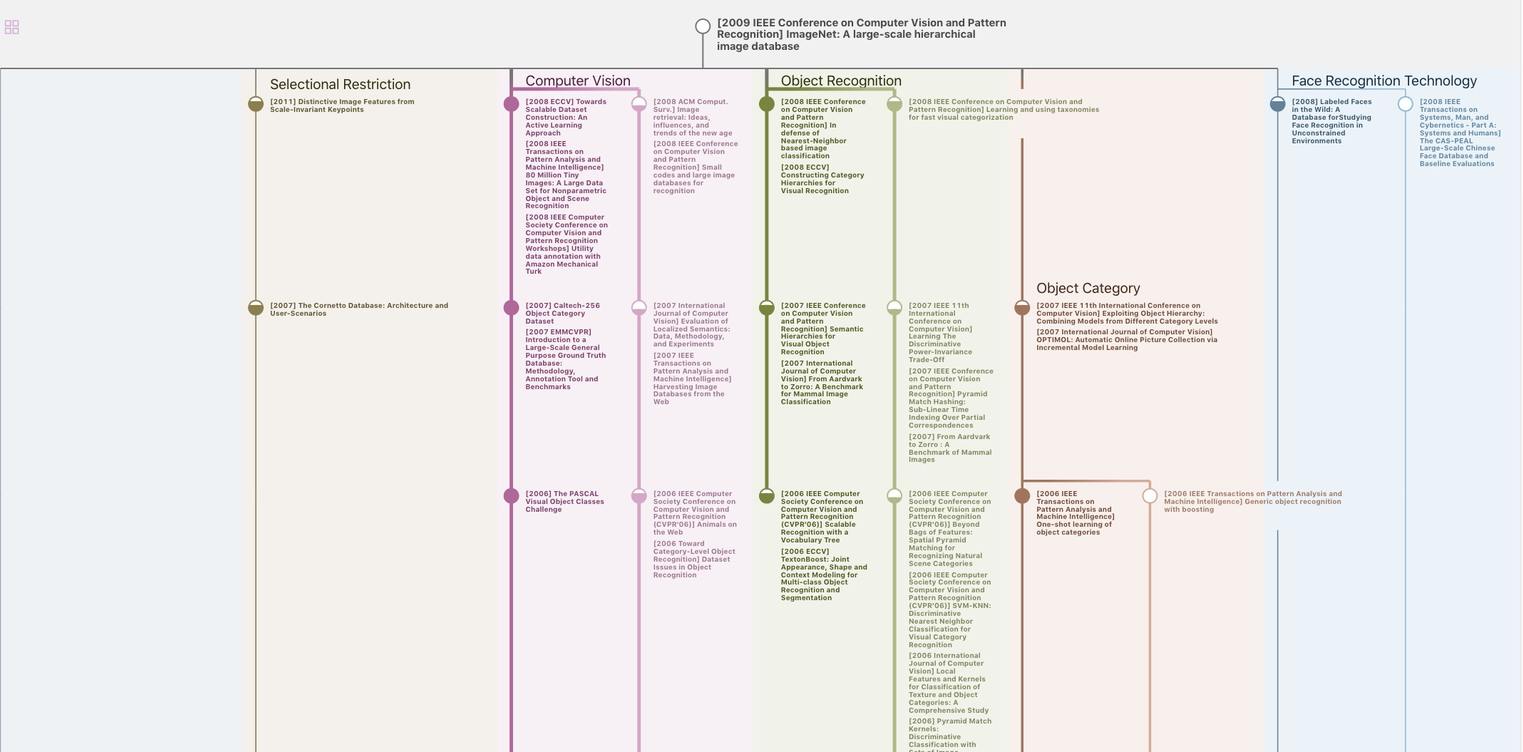
生成溯源树,研究论文发展脉络
Chat Paper
正在生成论文摘要