A CNN-SVR model for NO2 profile prediction based on MAX-DOAS observations: The influence of Chinese New Year overlapping the 2020 COVID-19 lockdown on vertical distributions of tropospheric NO2 in Nanjing, China
JOURNAL OF ENVIRONMENTAL SCIENCES(2024)
摘要
In this study, a hybrid model, the convolutional neural network-support vector regression model, was adopted to achieve prediction of the NO2 profile in Nanjing from January 2019 to March 2021. Given the sudden decline in NO2 in February 2020, the contribution of the Coronavirus Disease-19 (COVID-19) lockdown, Chinese New Year (CNY), and meteorological conditions to the reduction of NO2 was evaluated. NO2 vertical column densities (VCDs) from January to March 2020 decreased by 59.05% and 32.81%, relative to the same period in 2019 and 2021, respectively. During the period of 2020 COVID-19, the average NO2 VCDs were 50.50% and 29.96% lower than those during the pre-lockdown and post-lockdown periods, respectively. The NO2 volume mixing ratios (VMRs) during the 2020 COVID-19 lockdown significantly decreased below 400 m. The NO2 VMRs under the different wind fields were significantly lower during the lockdown period than during the pre-lockdown period. This phenomenon could be attributed to the 2020 COVID-19 lockdown. The NO2 VMRs before and after the CNY were significantly lower in 2020 than in 2019 and 2021 in the same period, which further proves that the decrease in NO2 in February 2020 was attributed to the COVID-19 lockdown. Pollution source analysis of an NO2 pollution episode during the lockdown period showed that the polluted air mass in the Beijing-Tianjin-Hebei was transported southwards under the action of the north wind, and the subsequent unfavorable meteorological conditions (local wind speed of < 2.0 m/sec) resulted in the accumulation of pollutants. (c) 2024 The Research Center for Eco-Environmental Sciences, Chinese Academy of Sciences. Published by Elsevier B.V.
更多查看译文
关键词
MAX-DOAS,CNN-SVR,Nitrogen dioxide,COVID-19,Chinese New Year,Regional transport
AI 理解论文
溯源树
样例
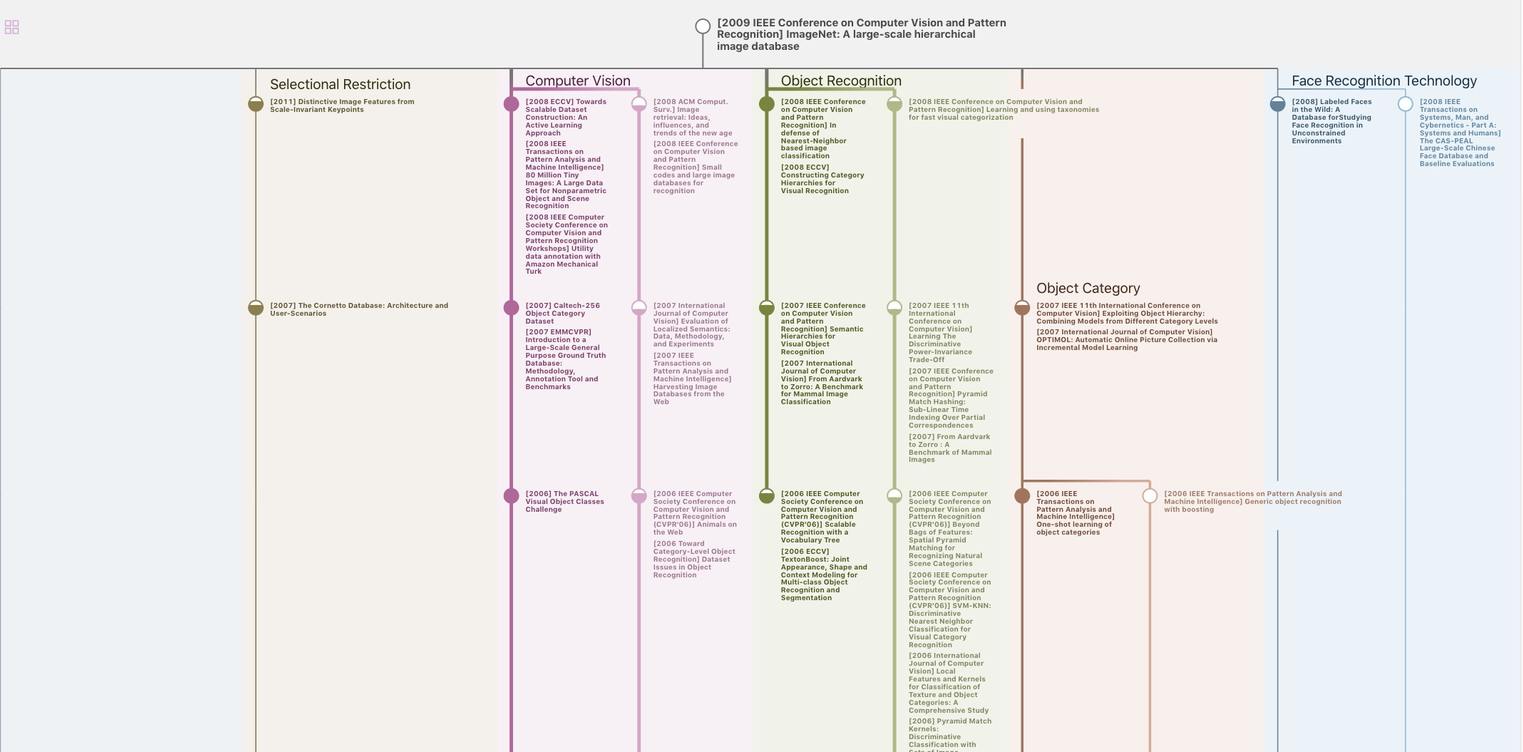
生成溯源树,研究论文发展脉络
Chat Paper
正在生成论文摘要