Hi-Core: Hierarchical Knowledge Transfer for Continual Reinforcement Learning
CoRR(2024)
摘要
Continual reinforcement learning (CRL) empowers RL agents with the ability to
learn from a sequence of tasks, preserving previous knowledge and leveraging it
to facilitate future learning. However, existing methods often focus on
transferring low-level knowledge across similar tasks, which neglects the
hierarchical structure of human cognitive control, resulting in insufficient
knowledge transfer across diverse tasks. To enhance high-level knowledge
transfer, we propose a novel framework named Hi-Core (Hierarchical knowledge
transfer for Continual reinforcement learning), which is structured in two
layers: 1) the high-level policy formulation which utilizes the powerful
reasoning ability of the Large Language Model (LLM) to set goals and 2) the
low-level policy learning through RL which is oriented by high-level goals.
Moreover, the knowledge base (policy library) is constructed to store policies
that can be retrieved for hierarchical knowledge transfer. Experiments
conducted in MiniGrid have demonstrated the effectiveness of Hi-Core in
handling diverse CRL tasks, outperforming popular baselines.
更多查看译文
AI 理解论文
溯源树
样例
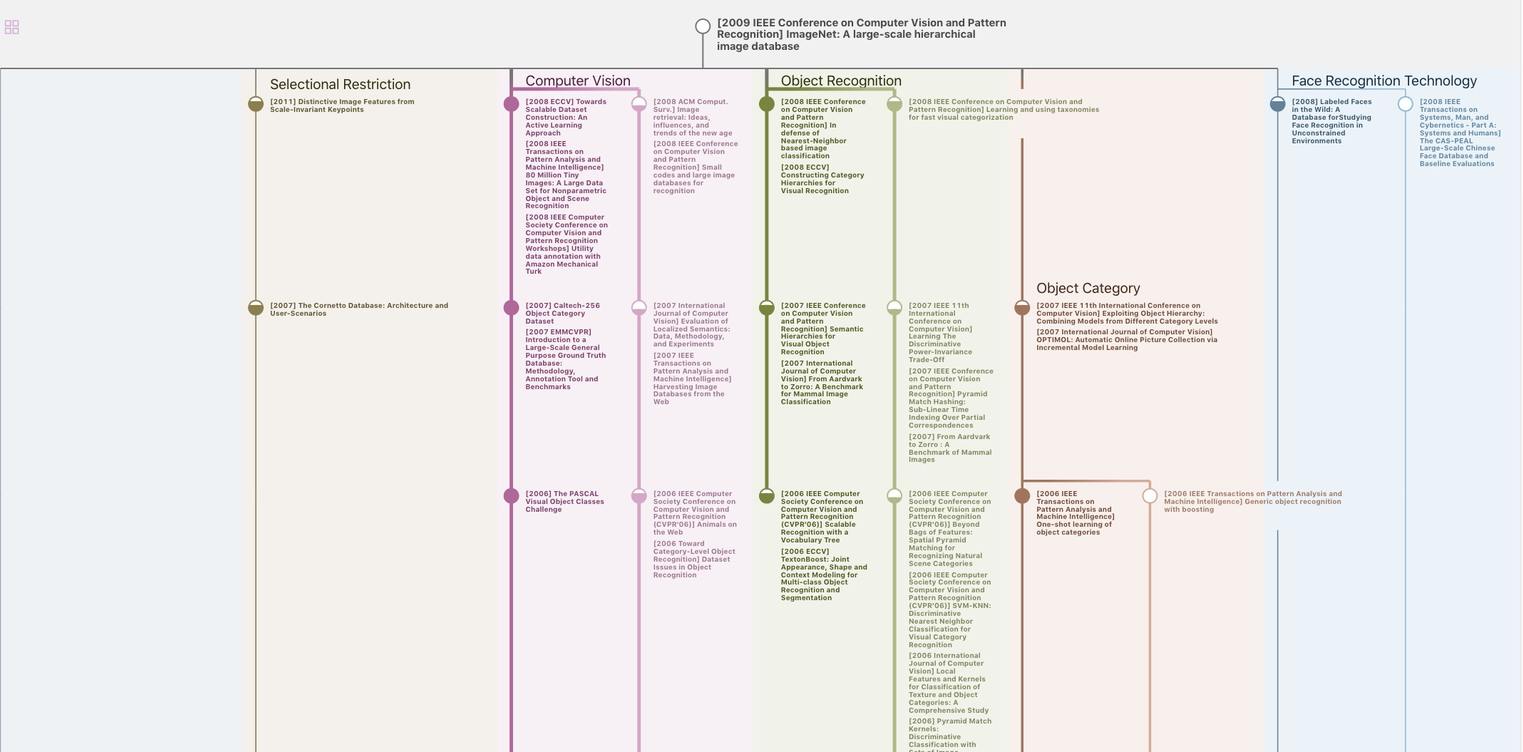
生成溯源树,研究论文发展脉络
Chat Paper
正在生成论文摘要