Enhancing Molecular Property Prediction with Auxiliary Learning and Task-Specific Adaptation
CoRR(2024)
摘要
Pretrained Graph Neural Networks have been widely adopted for various
molecular property prediction tasks. Despite their ability to encode structural
and relational features of molecules, traditional fine-tuning of such
pretrained GNNs on the target task can lead to poor generalization. To address
this, we explore the adaptation of pretrained GNNs to the target task by
jointly training them with multiple auxiliary tasks. This could enable the GNNs
to learn both general and task-specific features, which may benefit the target
task. However, a major challenge is to determine the relatedness of auxiliary
tasks with the target task. To address this, we investigate multiple strategies
to measure the relevance of auxiliary tasks and integrate such tasks by
adaptively combining task gradients or by learning task weights via bi-level
optimization. Additionally, we propose a novel gradient surgery-based approach,
Rotation of Conflicting Gradients (𝚁𝙲𝙶𝚛𝚊𝚍), that learns to align
conflicting auxiliary task gradients through rotation. Our experiments with
state-of-the-art pretrained GNNs demonstrate the efficacy of our proposed
methods, with improvements of up to 7.7
incorporating auxiliary tasks along with target task fine-tuning can be an
effective way to improve the generalizability of pretrained GNNs for molecular
property prediction.
更多查看译文
AI 理解论文
溯源树
样例
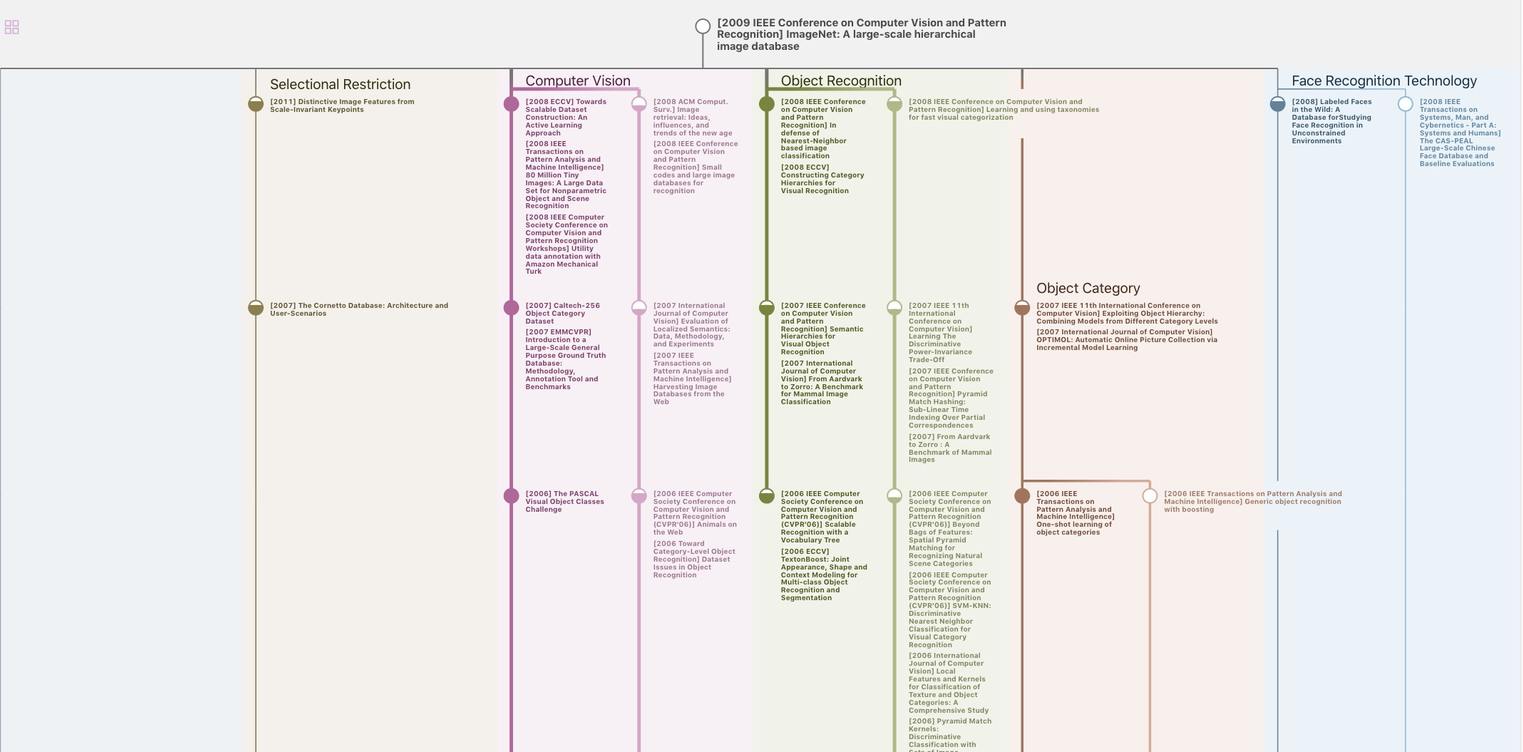
生成溯源树,研究论文发展脉络
Chat Paper
正在生成论文摘要