Fracture Zone Extraction Method Based on Three-Dimensional Convolutional Neural Network Combined with PointSIFT
LASER & OPTOELECTRONICS PROGRESS(2023)
摘要
This paper presents a fracture zone extraction method for complex terrain areas using a combination of a three-dimensional convolutional neural network (3D-CNN) and PointSIFT. The PointSIFT module encodes spatial orientation information of the original point cloud data to aggregate point cloud features, resulting in reconstructed point cloud data with different scale features. Subsequently, a 3D-CNN model is developed, with a 3D convolutional module serving as the primary component, to extract deep-level features from the reconstructed point cloud data. The extracted point cloud features are then fed into a fully connected layer for the categorization of the point clouds, addressing the challenge associated with fracture zone extraction. Comparative evaluations with the tensor decomposition method and deep neural network method are performed on two datasets. The results demonstrate that the proposed fracture zone extraction method achieves a lower classification error, thus confirming the superiority of the method in effectively extracting fracture zones from point cloud data.
更多查看译文
关键词
light detection and ranging,fracture zone extraction,deep learning,pointSIFT,three-dimensional convolution
AI 理解论文
溯源树
样例
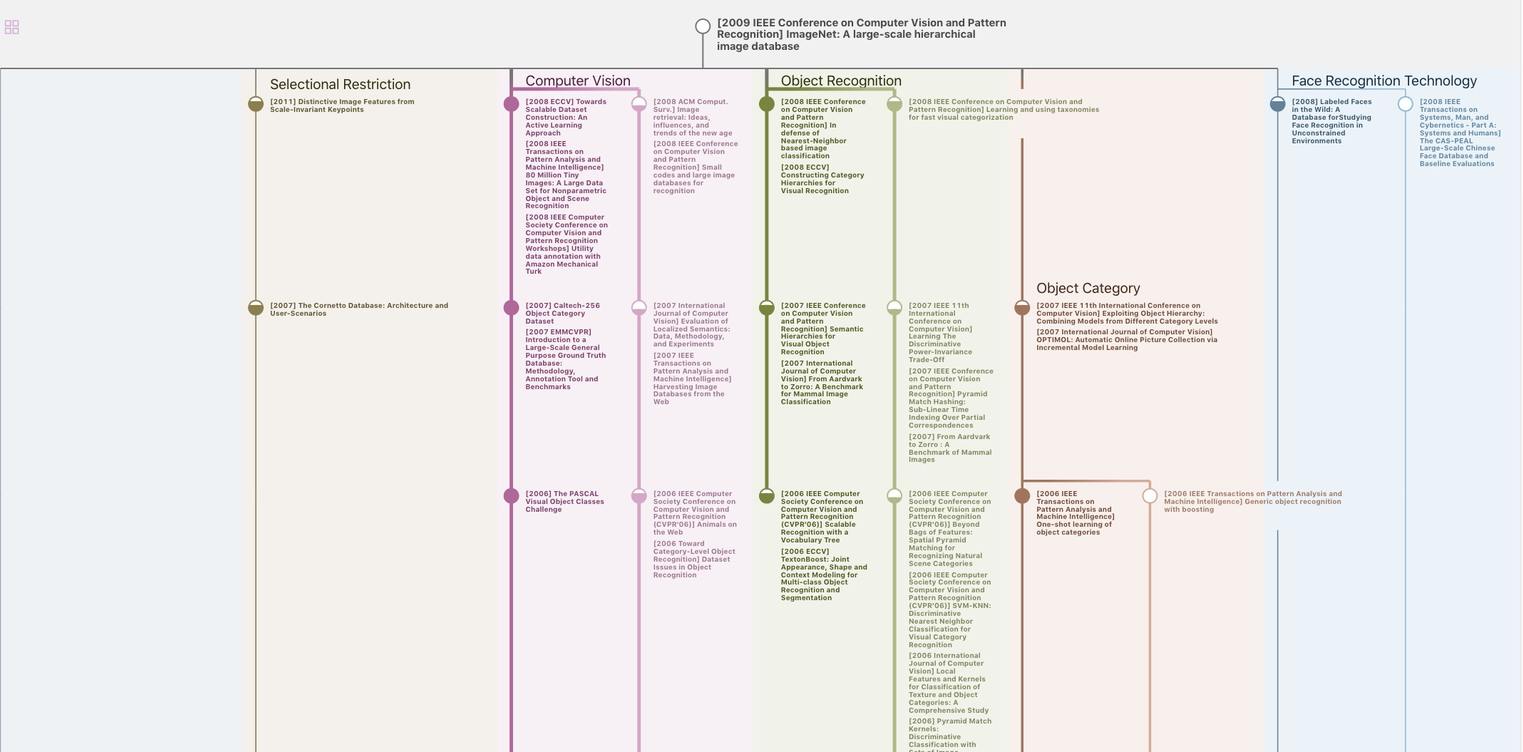
生成溯源树,研究论文发展脉络
Chat Paper
正在生成论文摘要