Language models enable zero-shot prediction of RNA secondary structures including pseudoknots
biorxiv(2024)
摘要
Current deep learning-based models for predicting RNA secondary structures face challenges in achieving high generalization ability. At the same time, a vast repository of unlabeled non-coding RNA (ncRNA) sequences remains untapped for structure prediction tasks. To address this challenge, we trained RNA-km, a foundation language model that enables zero-shot prediction of RNA secondary structures including pseudoknots. For the end, we incorporated specific modifications into the language model training process, including k-mer masking strategy and relative positional encoding. RNA-km are trained on 23 million ncRNA sequences in a self-supervised manner, gaining the advantages of high generalization ability. For a target RNA sequence, we make a zero-shot secondary structure prediction with the attention maps provided by RNA-km and a specified minimum-cost flow algorithm. Our results on popular benchmark datasets demonstrate that RNA-km exhibits high generalization abilities, excelling in zero-shot predictions for RNA secondary structures. In addition, the attention maps provided by the model capture intricate structural relationships, as evidenced by accurate pseudoknot predictions and precise identification of long-distance base pairs. We anticipate that RNA-km enhances the predictive capacity and robustness of existing models, thereby improving their ability to accurately predict structures for novel RNA sequences.
### Competing Interest Statement
The authors have declared no competing interest.
更多查看译文
AI 理解论文
溯源树
样例
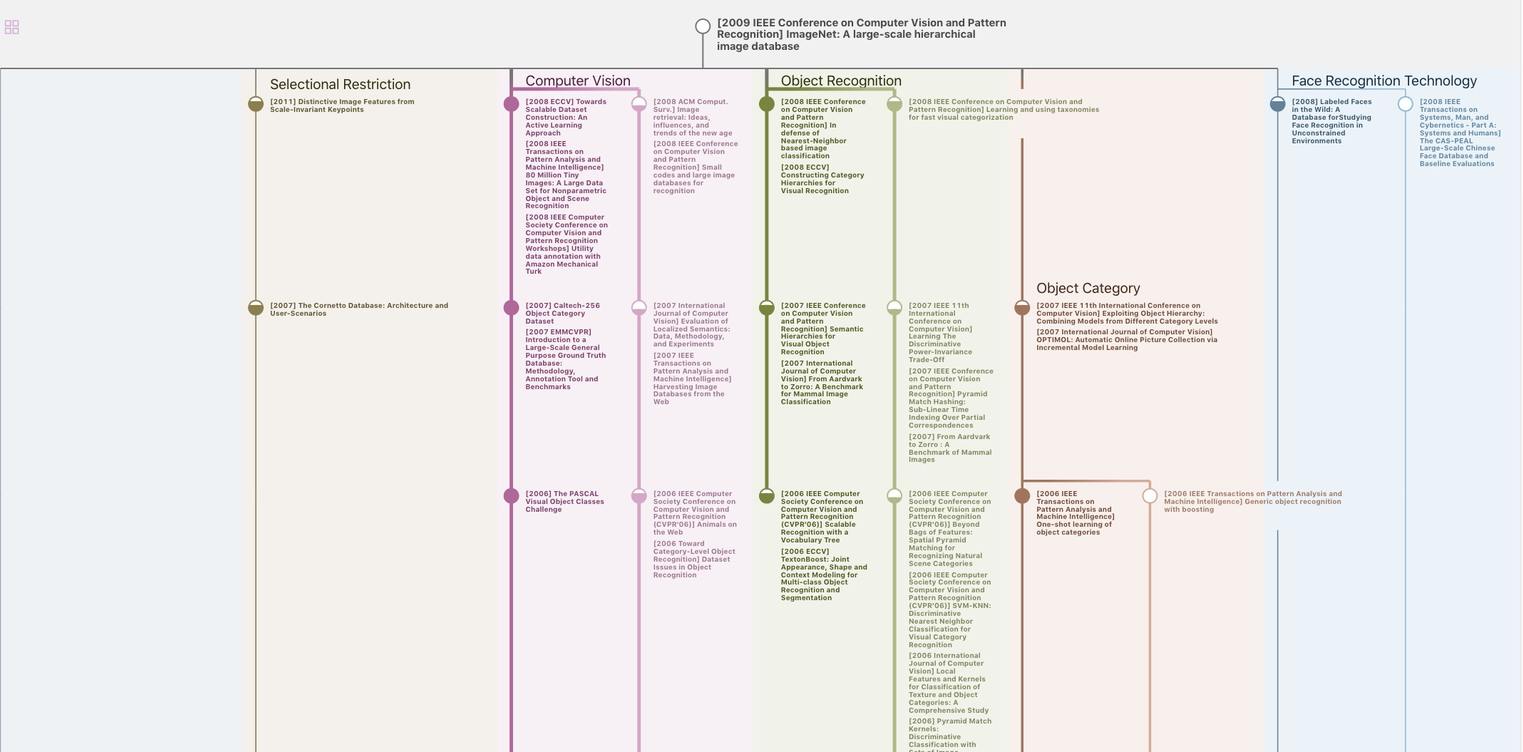
生成溯源树,研究论文发展脉络
Chat Paper
正在生成论文摘要