Multi-stream P&U adaptive graph convolutional networks for skeleton-based action recognition
The Journal of Supercomputing(2024)
摘要
In recent years, action recognition has been an essential branch of video understanding and a hot research direction. Among them, the graph convolutional network (GCN) is widely used in skeleton-based action recognition and has achieved remarkable performance. However, in practical situations, recognizing human action often depends on the movement of a part of the joints. In the existing GCN-based methods, the size of a single frame of the skeleton graph is fixed, and all joints of the human body will participate in the whole operation process, so the critical joints in the moving process cannot be flexibly selected. Therefore, this paper takes the adaptive graph convolutional network (AGCN) as the baseline and uses the graph-pooling method to select the critical joints in the human moving process. We design two new networks: Pooling-AGCN and U-AGCN and use them to form the multi-stream P&U AGCNs for action recognition. Extensive experiments show the complementarity between the two networks and that the method proposed in this paper outperforms the recent work on the three large-scale public datasets (NTU-RGB+D 60, NTU-RGB+D 120, Kinetics-Skeleton).
更多查看译文
关键词
Action recognition,Skeleton-based,Graph convolution network,Graph pooling,Multi-stream
AI 理解论文
溯源树
样例
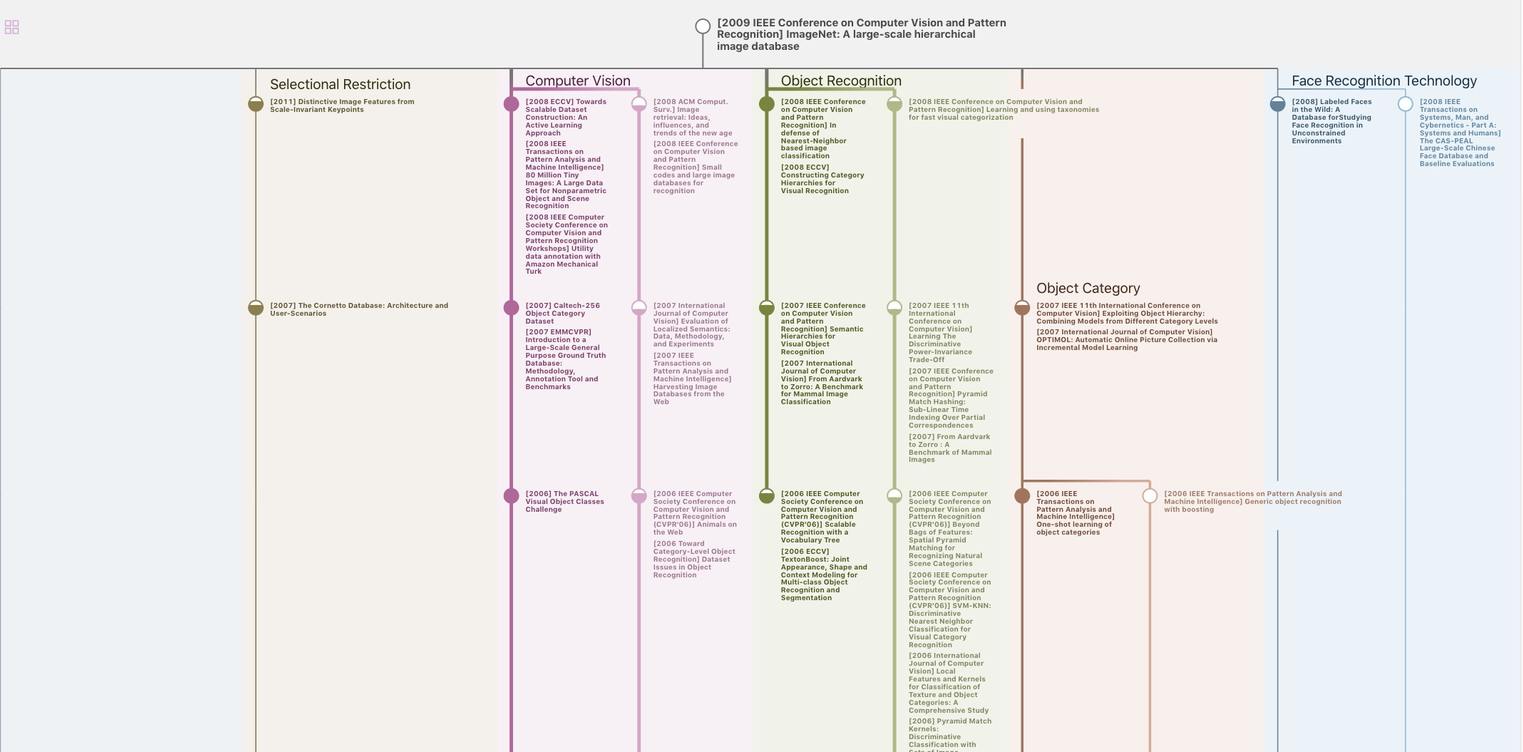
生成溯源树,研究论文发展脉络
Chat Paper
正在生成论文摘要