Multi-directional guidance network for fine-grained visual classification
The Visual Computer(2024)
摘要
Fine-grained images have a high confusion among subclasses. The key to this is finding discriminative regions that can be used for classification. The existing methods mainly use attention mechanisms or high-level linguistic information for classification, which only focus on the feature regions with the highest response and neglect other parts, resulting in inadequate capability for feature representation. Classification based on only a single feature part is not reliable. The fusion mechanism can achieve locating several different parts. However, simple feature fusion strategies do not exploit cross-layer information and lack the use of low-level information. To effectively address this limitation, we propose the multi-directional guidance network. Our network starts with a feature and attention guidance module that forces the network to learn detailed feature representations. Second, we propose a multi-layer guidance module that integrates diverse semantic information. In addition, we introduce a multi-way transfer structure to fuse low-level and high-level semantics in a novel way to improve generalization ability of the network. We have conducted extensive experiments on the FGVC benchmark dataset (CUB-200-2011, Stanford Cars and FGVC Aircraft) to demonstrate the superior performance of the method. Our code will be available at https://github.com/syyang2022/MGN .
更多查看译文
关键词
Fine-grained visual classification,Attention mechanism,Multi-layer interaction
AI 理解论文
溯源树
样例
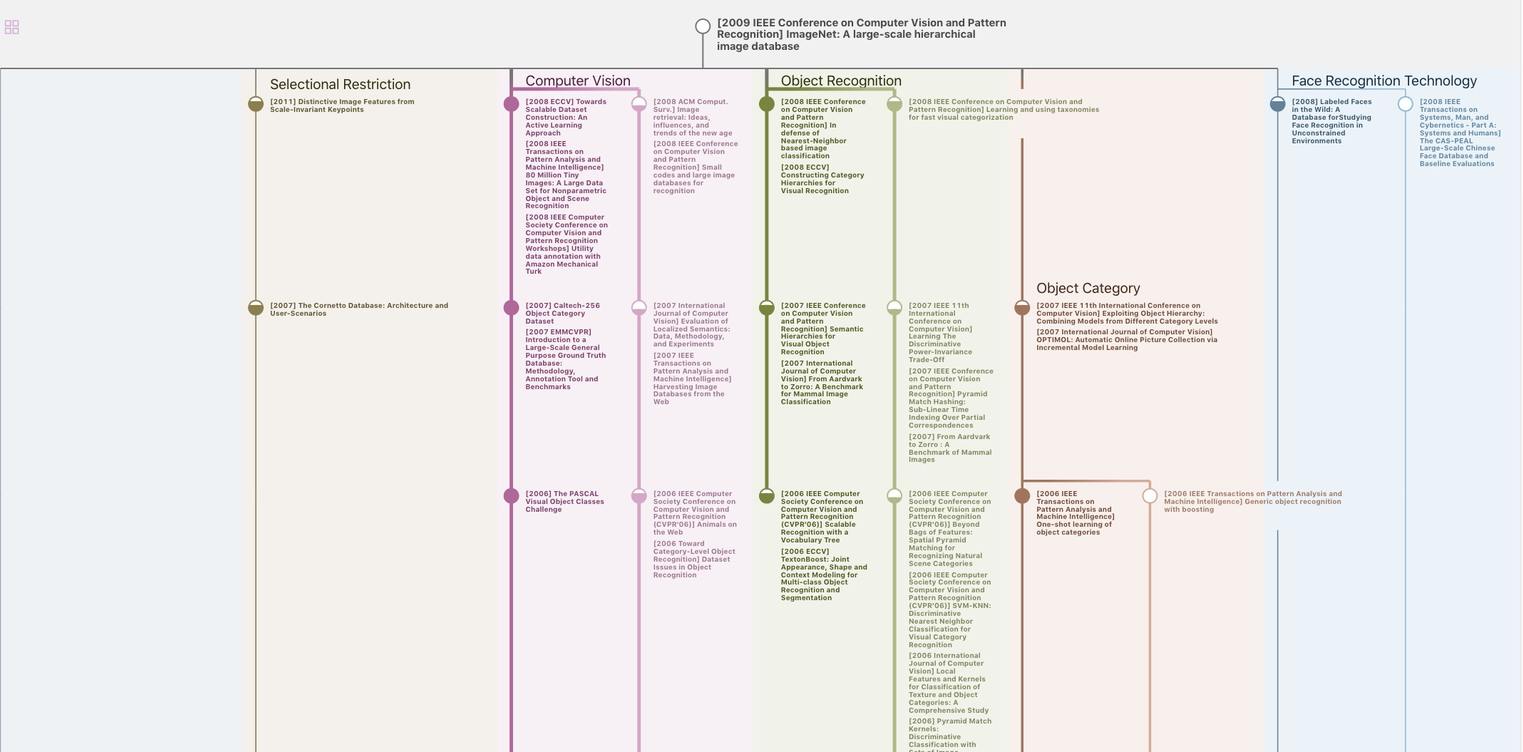
生成溯源树,研究论文发展脉络
Chat Paper
正在生成论文摘要