DPGS: Data-driven photovoltaic grid-connected system exploiting deep learning and two-stage single-phase inverter
ENERGY REPORTS(2024)
摘要
The increasing demand for clean energy to address the looming energy crisis has led to the widespread use of photovoltaic grid-connected technology, particularly in microgrids. To fully harness solar energy, this study proposes a data-driven strategy for photovoltaic maximum power point tracking with adaptive adjustment to environmental dynamics. Exploiting deep learning and incremental adjustment, our data-driven photovoltaicgrid systems (DPGS) upgrade the traditional perturbation and observation (P&O) MPPT to a dynamic evolutionary scheme. DPGS gathers the photovoltaic panel's output voltage and current, calculates the current power, and then outputs the appropriate reference voltage based on the power difference. The photovoltaic voltage is then adjusted using a data-driven strategy. In this study, a double-hidden layer deep learning network is utilized to output the prediction control signal of the first-stage circuit while continuously modifying the weight matrix and optimizing the tracking parameters of DPGS. Besides, a two-stage single-phase grid-connected photovoltaic inverter is designed to handle environmental dynamics. The simulation results validate the reliability of our suggested DPGS. DPGS often responds within 0.4 s, which is 33 % faster than conventional P&O techniques. DPGS has a power ripple rate that is approximately 78 % greater than conventional P&O approaches, at 0.022 %. DPGS has a quicker response time and less power fluctuation under external interference than traditional P&O MPPT. Our study contributes to the efficiency and reliability enhancement of grid-connected photovoltaic systems and has wide application in renewable energy systems.
更多查看译文
关键词
Data -driven photovoltaic -grid systems (DPGS),Maximum power point tracking (MPPT),Online tracking,Deep learning,Two -stage single-phase inverter
AI 理解论文
溯源树
样例
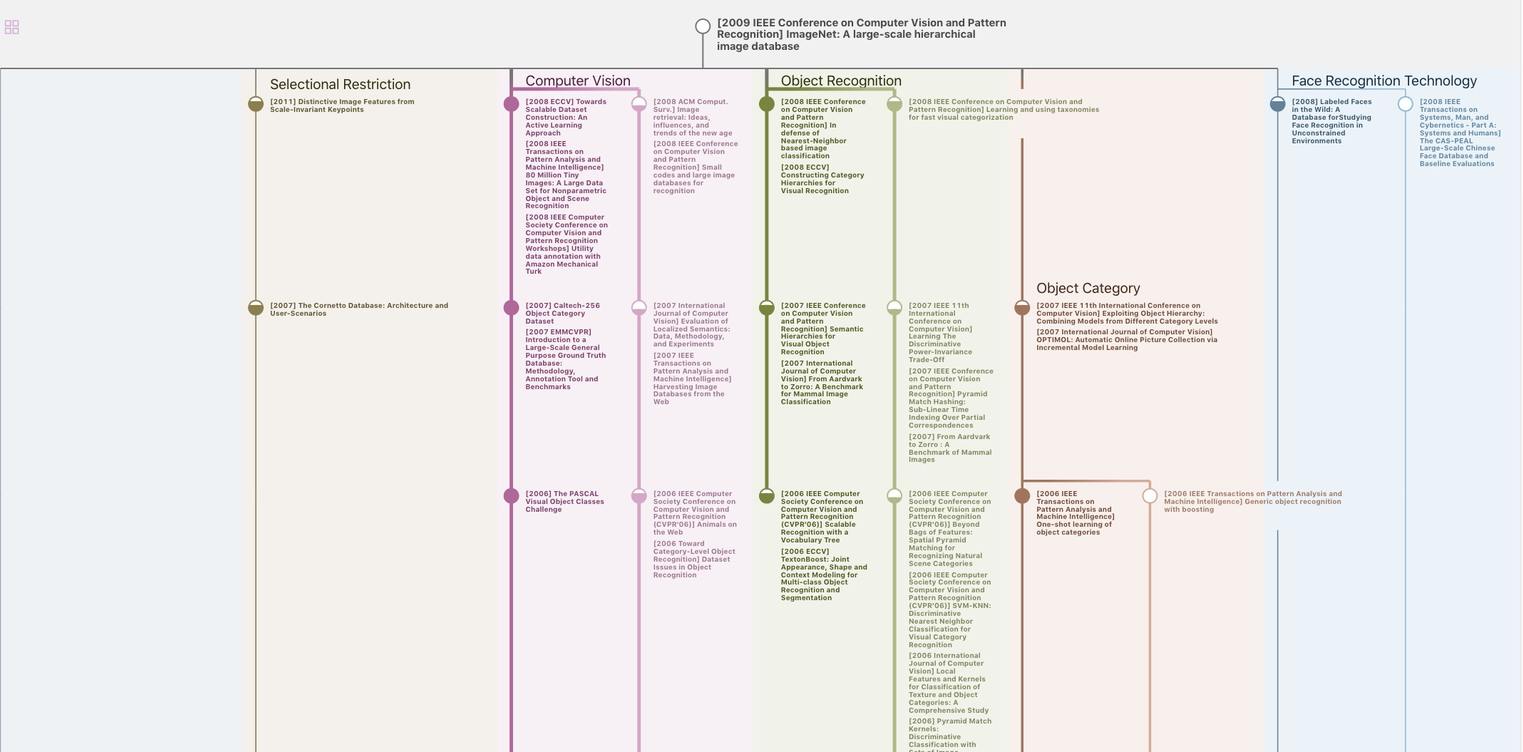
生成溯源树,研究论文发展脉络
Chat Paper
正在生成论文摘要