Overfitting NN loop-filters in video coding.
2023 IEEE International Conference on Visual Communications and Image Processing (VCIP)(2023)
摘要
Overfitting is usually regarded as a negative condition since it impairs the generalisation power of a model. Nevertheless, overfitting a Neural Network (NN) on test data may be advantageous to improve the compression efficiency of image/video coding tools and systems. Previous research has demonstrated the benefits of NN overfitting for post-processing operations, i.e. post-filters, but not yet for actual decoding tools. Generally, the NN is overfitted on test data at the encoder end, and the weight update is coded and sent to the decoder end along the image/video bitstream. The proposed approach follows this strategy. In particular, the overfitting of the Low Operation Point (LOP) loop-filter in NN-based Video Coding (NNVC) software is studied. The overall approach yields Bjøntegaard Delta rate (BD-rate) of -7.74%, -13.73% and -12.49%, for the Y, U and V components, respectively. Out of these coding gains, 1.21%, 6.43% and 5.52%, for the Y, U and V components, are attributed to the overfitting. The boost in the coding gains comes with only 1.5% more complexity, due to the multiplier parameters introduced during the overfitting.
更多查看译文
关键词
Loop-filter,neural network,overfitting,video coding
AI 理解论文
溯源树
样例
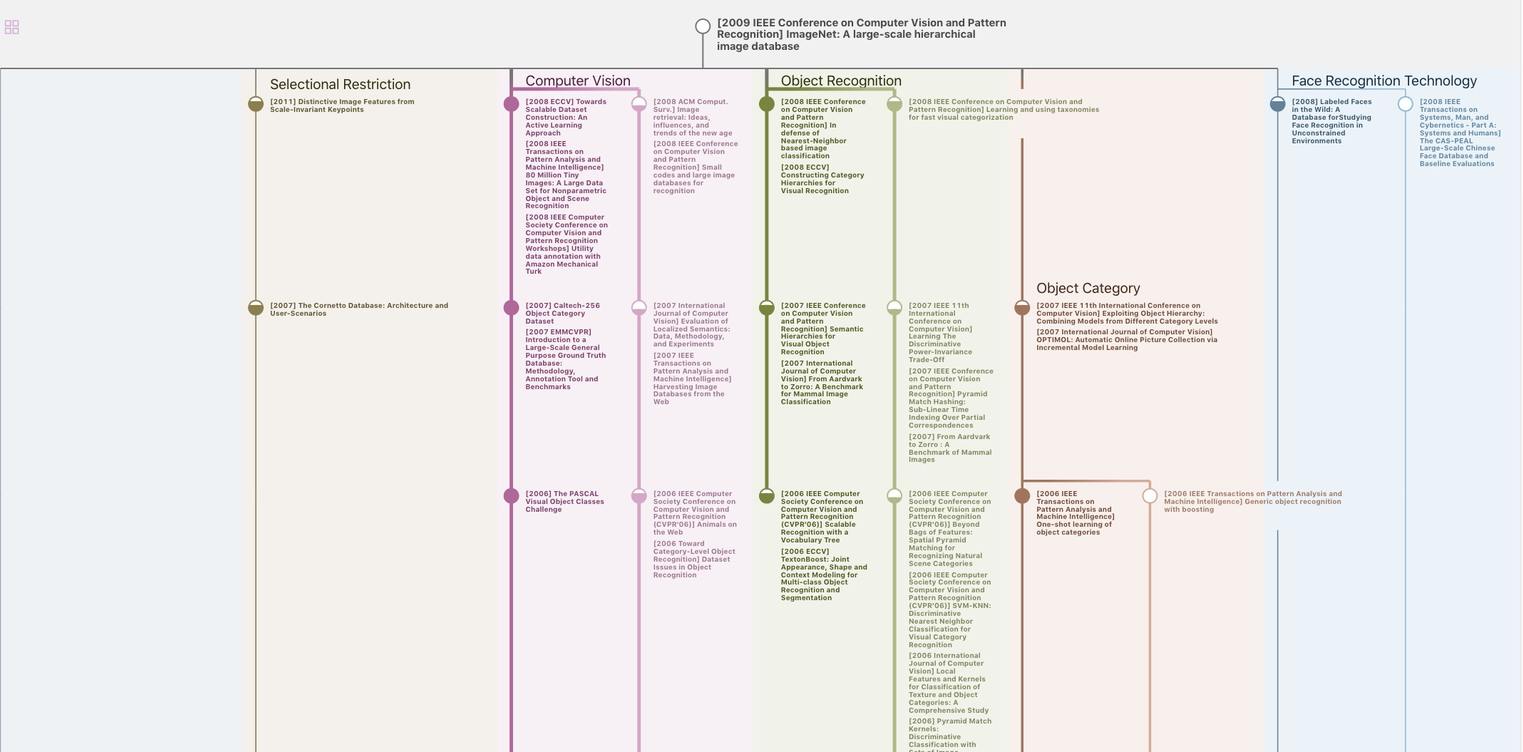
生成溯源树,研究论文发展脉络
Chat Paper
正在生成论文摘要