Byzantine-Resilient Federated Learning Leveraging Confidence Score to Identify Retinal Disease.
2023 International Conference on Digital Image Computing: Techniques and Applications (DICTA)(2023)
摘要
Federated learning is a distributed machine learning paradigm that enables multiple actors to collaboratively train a common model without sharing their local data, thus addressing data privacy issues, especially in sensitive domains such as healthcare. However, federated learning is vulnerable to poisoning attacks, where malicious (Byzantine) clients can manipulate their local updates to degrade the performance or compromise the privacy of the global model. To mitigate this problem, this paper proposes a novel method that reduces the influence of malicious clients based on their confidence. We evaluate our method on the Retinal OCT dataset consisting of age-related macular degeneration and diabetic macular edema, using InceptionV3 and VGG19 architecture. The proposed technique significantly improves the global model’s precision, recall, F1 score, and area under the receiver operating characteristic curve (AUC-ROC) for both InceptionV3 and VGG19. For InceptionV3, precision rises from 0.869 to 0.906, recall rises from 0.836 to 0.889, and F1 score rises from 0.852 to 0.898. For VGG19, precision rises from 0.958 to 0.963, recall rises from 0.917 to 0.941, and F1 score rises from 0.937 to 0.952.
更多查看译文
关键词
Computer Vision,Federated learning,Deep Learning,Medical Image Processing,Data poisoning,Retinal OCT
AI 理解论文
溯源树
样例
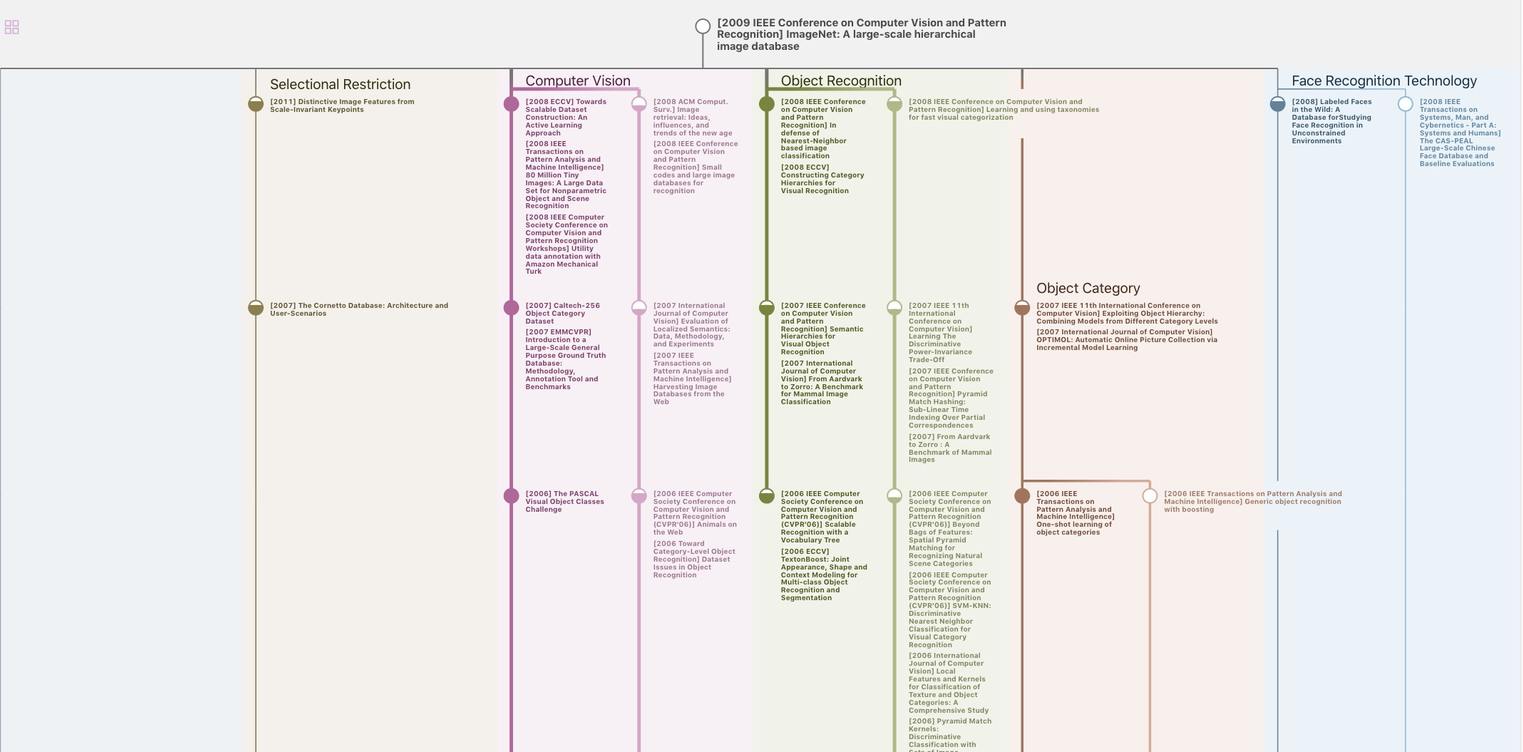
生成溯源树,研究论文发展脉络
Chat Paper
正在生成论文摘要