Fully Automated Detection and Segmentation Pipeline for the Bone Marrow of the Lytic Bone of Multiple Myeloma Patients
2023 IEEE EMBS Special Topic Conference on Data Science and Engineering in Healthcare, Medicine and Biology(2023)
摘要
Monitoring the changes in bone marrow during therapy for multiple myeloma patients is a crucial task. Osteolytic lesions can cause deformation of the bones, affecting the robustness of traditional segmentation tools. A two-model deep learning analysis is explored in this study. A detection model reduces pixel imbalances between the background and the bone marrow pixels, achieving a mAP of 0.878±0.005. A residual U-Net segments the bone marrow, yielding a DSC of 0.856±0.003. The proposed deep learning-based segmentation pipeline allows accurate and fast annotation of the bone marrow in multiple myeloma patients.Clinical Relevance: The proposed deep learning-based pipeline for segmentation has the potential to fully automate the time-consuming process of delineating bone marrow in multiple myeloma patients, significantly improving patient monitoring.
更多查看译文
关键词
Bone Marrow,Multiple Myeloma,Multiple Myeloma Patients,Segmentation Pipeline,Dice Similarity Coefficient,Skeletal Dysplasia,Bone Marrow Of Patients,Osteolytic Lesions,Marrow Of Patients,Bone Marrow Changes,Attention Mechanism,Intersection Over Union,Residual Connection,Faster R-CNN,Femoral Shaft,Whole-body Computed Tomography
AI 理解论文
溯源树
样例
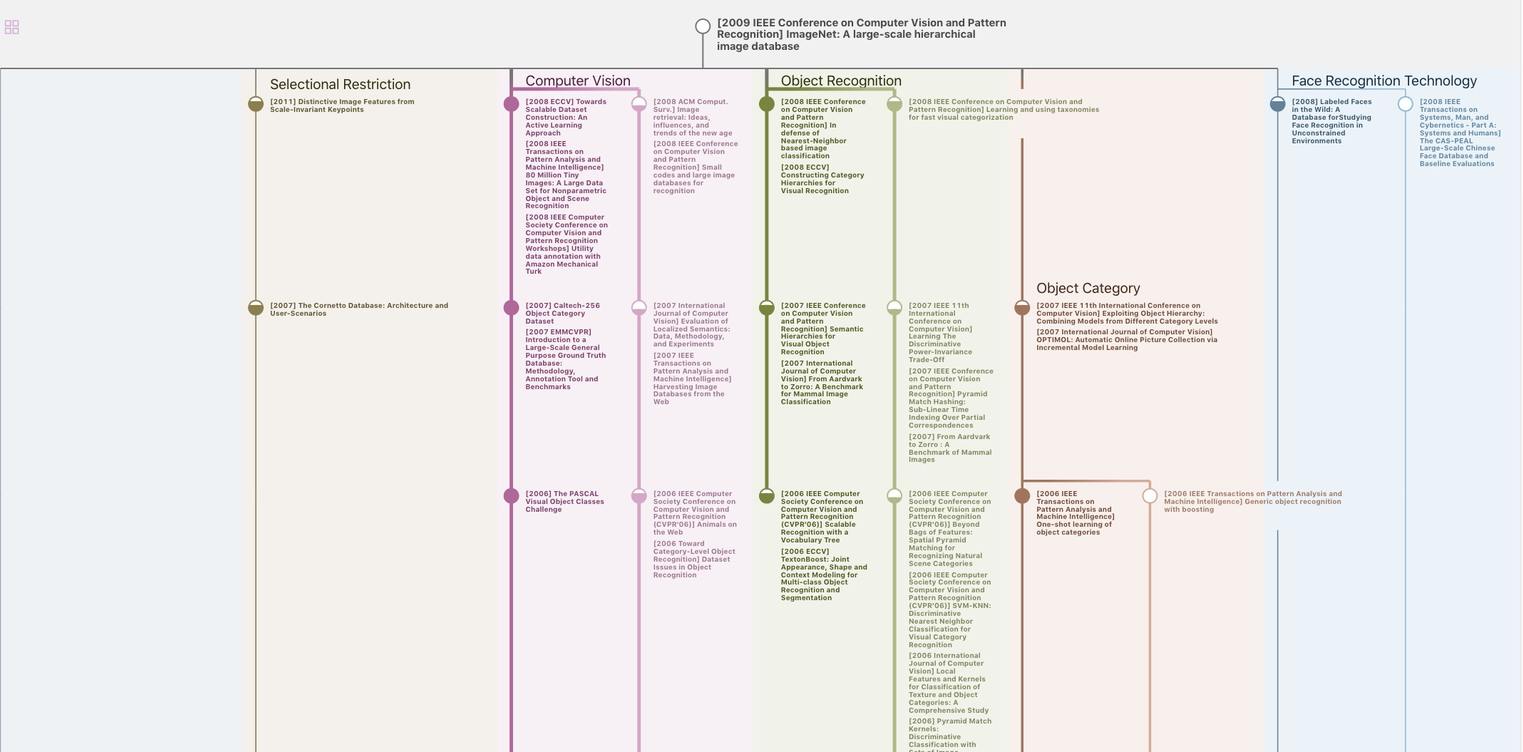
生成溯源树,研究论文发展脉络
Chat Paper
正在生成论文摘要