Ultrasound based machine learning classification to match imaging properties of real tissues to artificial structures
2023 IEEE EMBS Special Topic Conference on Data Science and Engineering in Healthcare, Medicine and Biology(2023)
摘要
Advancements in sensors, computing, and surgical data science have improved medical treatments, particularly minimally invasive procedures (MIPs), offering benefits like faster healing and reduced patient discomfort. However, challenges persist in terms of accuracy, safety, and awareness during MIPs, including difficulties in locating and tracking minimally invasive devices within the body. The research aims to address these challenges by developing sensor-based guidance methods and transformation algorithms, together with machine learning, achieving a mean F1 score of 0.8 in classifying ultrasound images of tested materials. This represents a step toward real-time tissue differentiation, ultimately offering safer and more accurate MIPs.Clinical relevance: This paper enhances MIPs by creating realistic interventional phantoms for training and device testing, reducing errors improving patient safety, and integrating machine learning classifications for real-time guidance.
更多查看译文
关键词
Machine Learning,Minimally Invasive,F1 Score,Ultrasound Imaging,Minimally Invasive Surgery,Computed Tomography,Support Vector Machine,Random Forest,Random Forest Algorithm,Tissue Classification,Quadratic Discriminant Analysis,Gelatinous Layer
AI 理解论文
溯源树
样例
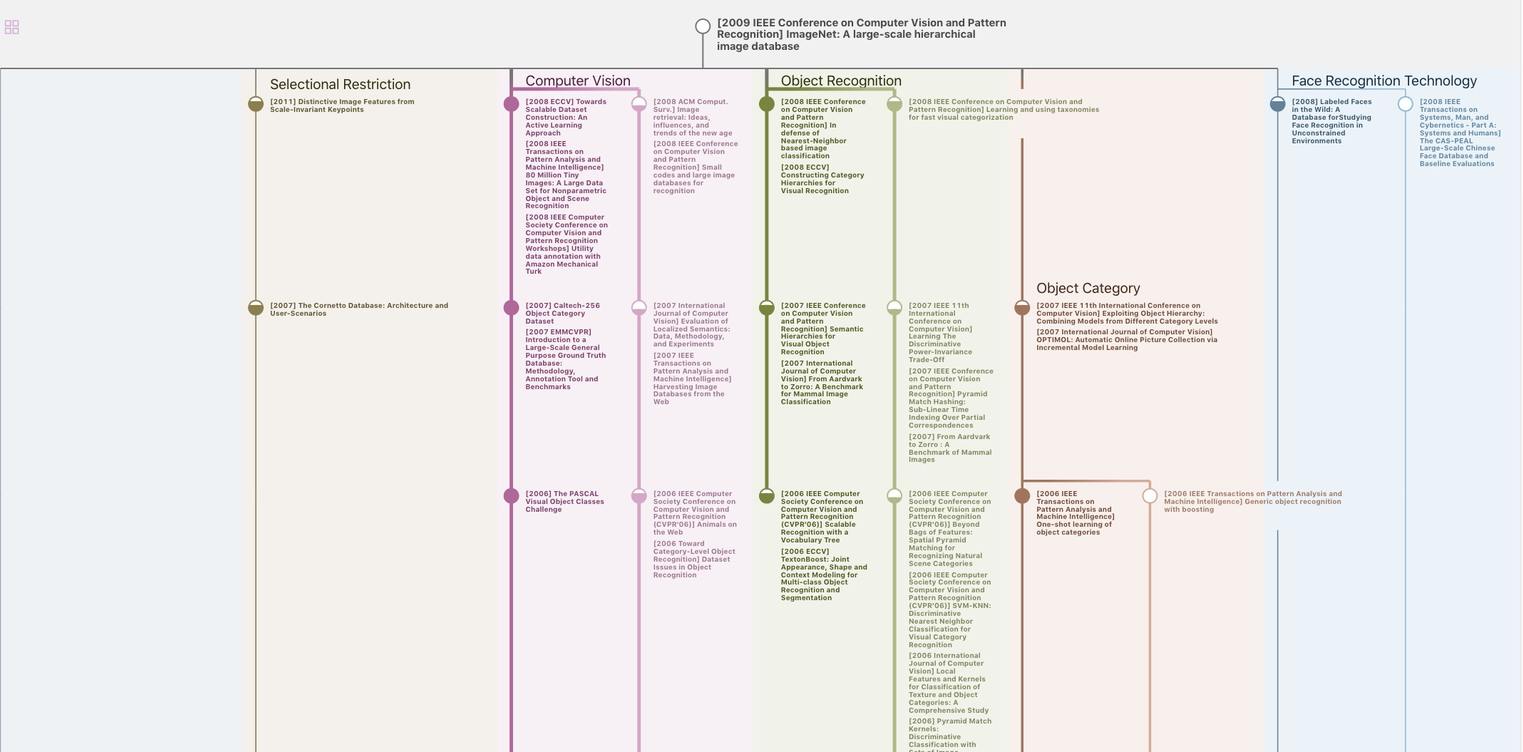
生成溯源树,研究论文发展脉络
Chat Paper
正在生成论文摘要