Structure-aware Deep Model for MHC-II Peptide Binding Affinity Prediction.
BMC genomics(2024)
摘要
The prediction of major histocompatibility complex (MHC)-peptide binding affinity is an important branch in immune bioinformatics, especially helpful in accelerating the design of disease vaccines and immunity therapy. Although deep learning-based solutions have yielded promising results on MHC-II molecules in recent years, these methods ignored structure knowledge from each peptide when employing the deep neural network models. Each peptide sequence has its specific combination order, so it is worth considering adding the structural information of the peptide sequence to the deep model training. In this work, we use positional encoding to represent the structural information of peptide sequences and validly combine the positional encoding with existing models by different strategies. Experiments on three datasets show that the introduction of position-coding information can further improve the performance built upon the existing model. The idea of introducing positional encoding to this field can provide important reference significance for the optimization of the deep network structure in the future.
更多查看译文
关键词
MHC-II molecules,Affinity prediction,Positional embedding
AI 理解论文
溯源树
样例
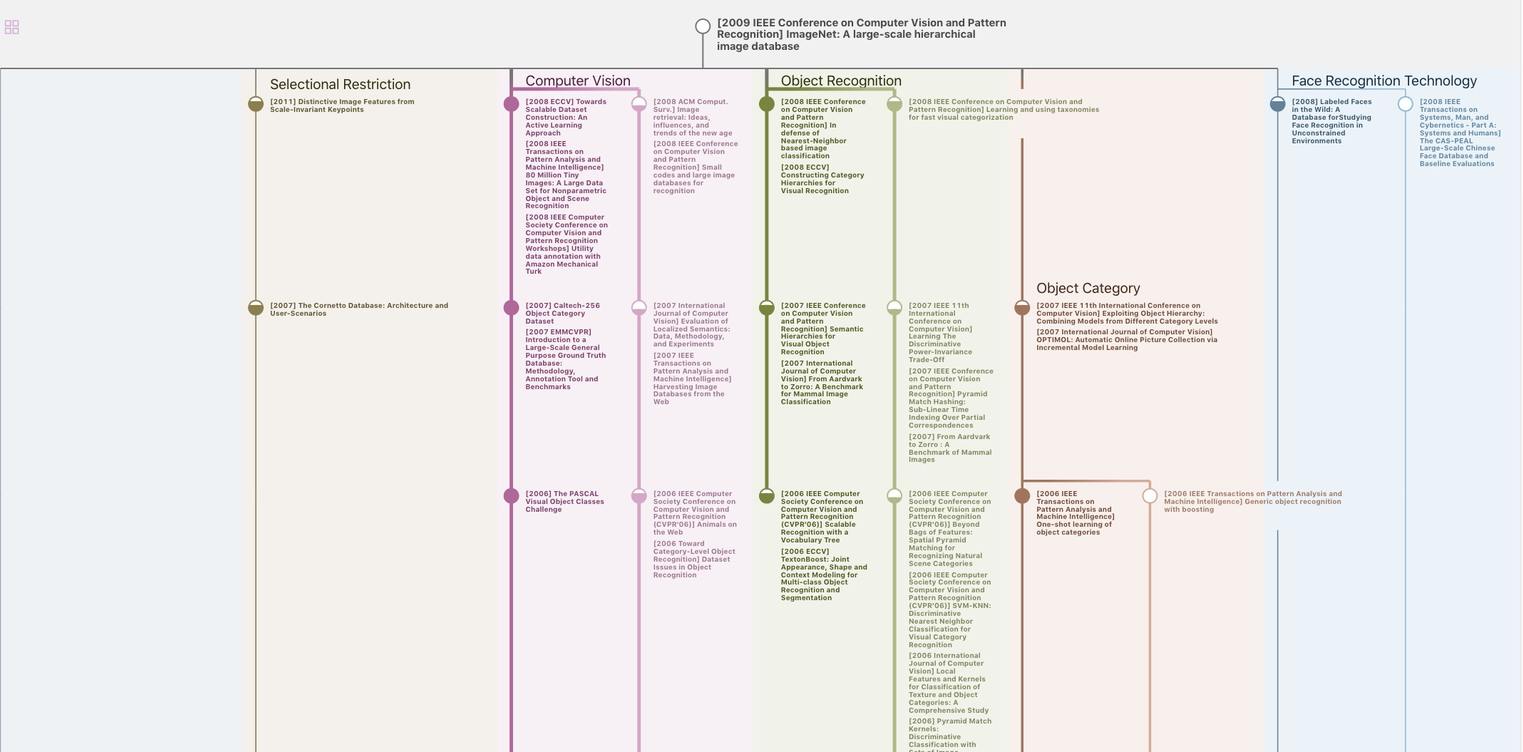
生成溯源树,研究论文发展脉络
Chat Paper
正在生成论文摘要